TransFR: Transferable Federated Recommendation with Pre-trained Language Models
CoRR(2024)
摘要
Federated recommendations (FRs), facilitating multiple local clients to
collectively learn a global model without disclosing user private data, have
emerged as a prevalent architecture for privacy-preserving recommendations. In
conventional FRs, a dominant paradigm is to utilize discrete identities to
represent users/clients and items, which are subsequently mapped to
domain-specific embeddings to participate in model training. Despite
considerable performance, we reveal three inherent limitations that can not be
ignored in federated settings, i.e., non-transferability across domains,
unavailability in cold-start settings, and potential privacy violations during
federated training. To this end, we propose a transferable federated
recommendation model with universal textual representations, TransFR, which
delicately incorporates the general capabilities empowered by pre-trained
language models and the personalized abilities by fine-tuning local private
data. Specifically, it first learns domain-agnostic representations of items by
exploiting pre-trained models with public textual corpora. To tailor for
federated recommendation, we further introduce an efficient federated
fine-tuning and a local training mechanism. This facilitates personalized local
heads for each client by utilizing their private behavior data. By
incorporating pre-training and fine-tuning within FRs, it greatly improves the
adaptation efficiency transferring to a new domain and the generalization
capacity to address cold-start issues. Through extensive experiments on several
datasets, we demonstrate that our TransFR model surpasses several
state-of-the-art FRs in terms of accuracy, transferability, and privacy.
更多查看译文
AI 理解论文
溯源树
样例
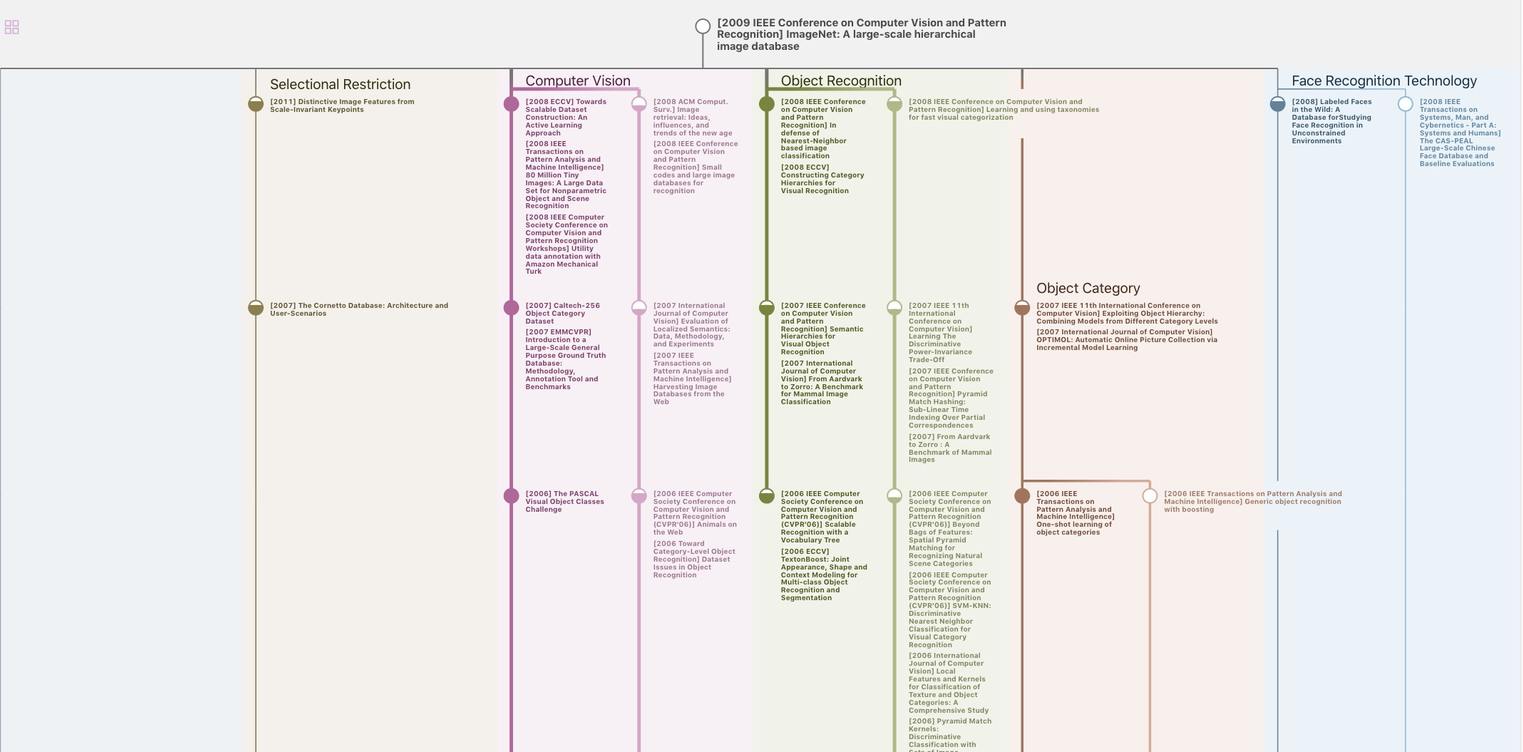
生成溯源树,研究论文发展脉络
Chat Paper
正在生成论文摘要