Identifying virulence factors using graph transformer autoencoder with ESMFold-predicted structures
COMPUTERS IN BIOLOGY AND MEDICINE(2024)
摘要
With the increasing resistance of bacterial pathogens to conventional antibiotics, antivirulence strategies targeting virulence factors (VFs) have become an effective new therapy for the treatment of pathogenic bacterial infections. Therefore, the identification and prediction of VFs can provide ideal candidate targets for the implementation of antivirulence strategies in treating infections caused by pathogenic bacteria. Currently, the existing computational models predominantly rely on the amino acid sequences of virulence proteins while overlooking structural information. Here, we propose a novel graph transformer autoencoder for VF identification (GTAE-VF), which utilizes ESMFold-predicted 3D structures and converts the VF identification problem into a graph-level prediction task. In an encoder-decoder framework, GTAE-VF adaptively learns both local and global information by integrating a graph convolutional network and a transformer to implement all-pair message passing, which can better capture long-range correlations and potential relationships. Extensive experiments on an independent test dataset demonstrate that GTAE-VF achieves reliable and robust prediction accuracy with an AUC of 0.963, which is consistently better than that of other structure-based and sequencebased approaches. We believe that GTAE-VF has the potential to emerge as a valuable tool for assessing VFs and devising antivirulence strategies.
更多查看译文
关键词
Virulence factor identification,ESMFold,Predicted protein structure,Graph transformer autoencoder
AI 理解论文
溯源树
样例
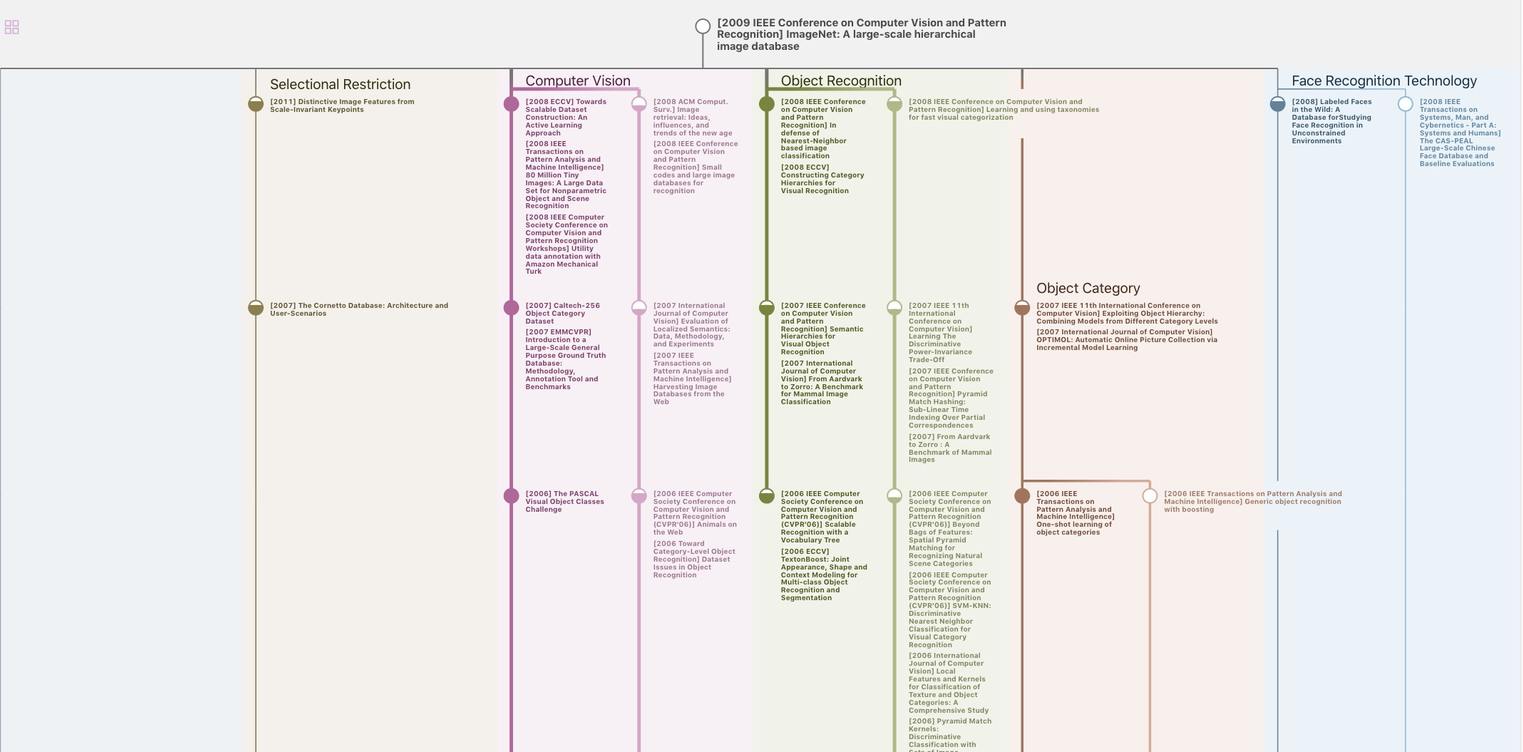
生成溯源树,研究论文发展脉络
Chat Paper
正在生成论文摘要