Geometry repeatability and prediction for personalized medical devices made using multi-jet fusion additive manufacturing
Additive Manufacturing Letters(2024)
摘要
As additive manufacturing (AM) production volumes grow to the industrial scale, quality systems must also scale to verify that every part satisfies requirements. Quality systems are particularly challenging for personalized medical devices, where every patient requires a unique design. This research studies the repeatability of an additively manufactured guide for knee surgery that is personalized to the size and shape of a patient and explores concepts for predicting geometric accuracy. We created 258 unique surgical guide designs with different sizes of the critical features to simulate practical conditions, and manufactured 2100 parts using multi-jet fusion AM. An automated measurement technique collected 8400 individual feature dimensions. Across four critical features, the standard deviation of feature size was 0.076 to 0.173 mm, however the accuracy was consistently different than the target dimensions by -0.308 to 0.017 mm. We show how machine learning (ML) models can predict these geometry distortions and explore the number of parts required to effectively train these models. The accuracy of these models are 0.033 to 0.075 mm, such that the part shape distortion can be accurately predicted to within one standard deviation across a wide range of part sizes.
更多查看译文
关键词
Multi-jet fusion,Surgical guide,Metrology,Process repeatability,Part distortion,Statistical modeling,Machine learning,Additive manufacturing
AI 理解论文
溯源树
样例
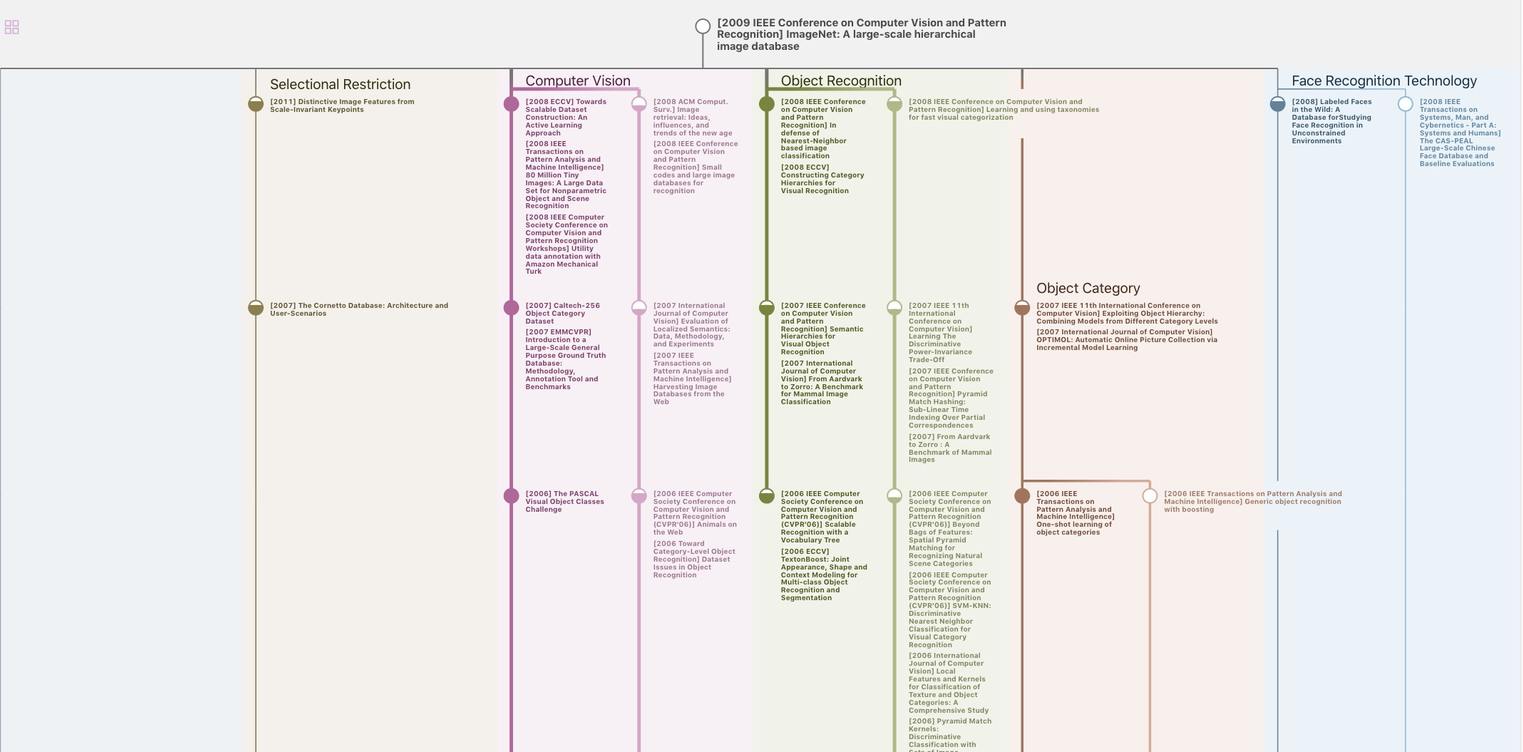
生成溯源树,研究论文发展脉络
Chat Paper
正在生成论文摘要