Multi-scale progressive fusion-based depth image completion and enhancement for industrial collaborative robot applications
Journal of Intelligent Manufacturing(2024)
摘要
The depth image obtained by consumer-level depth cameras generally has low resolution and missing regions due to the limitations of the depth camera hardware and the method of depth image generation. Despite the fact that many studies have been done on RGB image completion and super-resolution, a key issue with depth images is that there will be evident jagged boundaries and a significant loss of geometric information. To address these issues, we introduce a multi-scale progressive fusion network for depth image completion and super-resolution in this paper, which has an asymptotic structure for integrating hierarchical features in different domains. We employ two separate branches to learn the features of a multi-scale image given a depth image and its corresponding RGB image. The extracted features are then fused into different level features of these two branches using a step-by-step strategy to recreate the final depth image. To confine distinct borders and geometric features, a multi-dimension loss is also designed. Extensive depth completion and super-resolution studies reveal that our proposed method outperforms state-of-the-art methods both qualitatively and quantitatively. The proposed methods are also applied to two human–robot interaction applications, including a remote-controlled robot based on an unmanned ground vehicle (UGV), AR-based toolpath planning, and automatic toolpath extraction. All these experimental results indicate the effectiveness and potential benefits of the proposed methods.
更多查看译文
关键词
Human–robot-interaction,Collaborative robot,Fusion-learning,Depth image completion,Super resolution,Multi-scale network
AI 理解论文
溯源树
样例
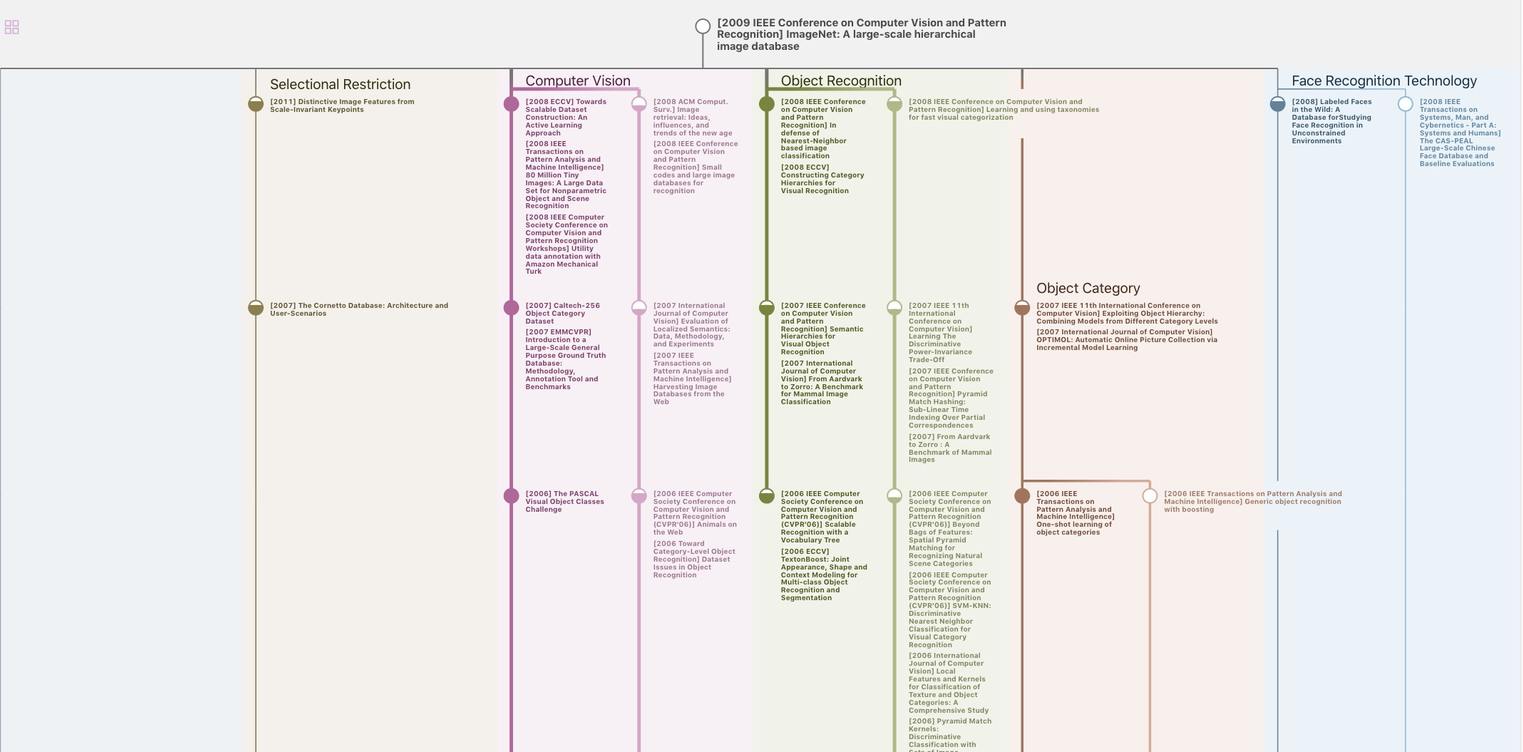
生成溯源树,研究论文发展脉络
Chat Paper
正在生成论文摘要