Using unsupervised machine learning to classify behavioral risk markers of bacterial vaginosis
Archives of Gynecology and Obstetrics(2024)
摘要
Introduction This study used an unsupervised machine learning algorithm, sidClustering and random forests, to identify clusters of risk behaviors of Bacterial Vaginosis (BV), the most common cause of abnormal vaginal discharge linked to STI and HIV acquisition. Methods Participants were 391 cisgender women in Miami, Florida, with a mean of 30.8 (SD = 7.81) years of age; 41.7% identified as Hispanic; 41.7% as Black and 44.8% as White. Participants completed measures of demographics, risk behaviors [sexual, medical, and reproductive history, substance use, and intravaginal practices (IVP)], and underwent collection of vaginal samples; 135 behavioral variables were analyzed. BV was diagnosed using Nugent criteria. Results We identified four clusters, and variables were ranked by importance in distinguishing clusters: Cluster 1: nulliparous women who engaged in IVPs to clean themselves and please sexual partners, and used substances frequently [ n = 118 (30.2%)]; Cluster 2: primiparous women who engaged in IVPs using vaginal douches to clean themselves ( n = 112 (28.6%)]; Cluster 3: primiparous women who did not use IVPs or substances [ n = 87 (22.3%)]; and Cluster 4: nulliparous women who did not use IVPs but used substances [ n = 74 (18.9%)]. Clusters were related to BV ( p < 0.001). Cluster 2, the cluster of women who used vaginal douches as IVPs, had the highest prevalence of BV (52.7%). Conclusions Machine learning methods may be particularly useful in identifying specific clusters of high-risk behaviors, in developing interventions intended to reduce BV and IVP, and ultimately in reducing the risk of HIV infection among women.
更多查看译文
关键词
Bacterial vaginosis,Unsupervised machine learning,Sexual behavior,Women
AI 理解论文
溯源树
样例
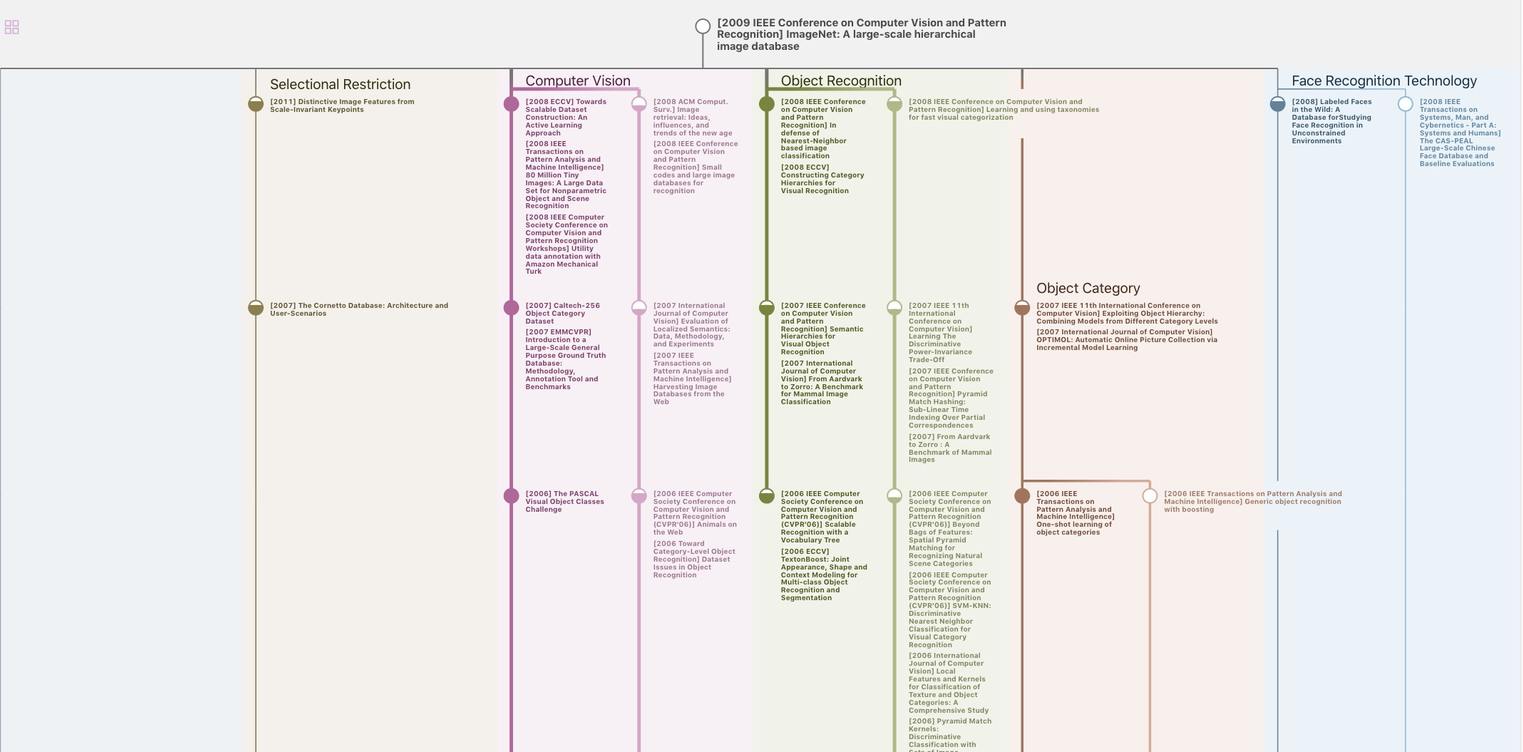
生成溯源树,研究论文发展脉络
Chat Paper
正在生成论文摘要