OverGNN Assisted Power Allocation for Heterogeneous Ultra-Dense Networks.
2023 International Conference on Wireless Communications and Signal Processing (WCSP)(2023)
摘要
With the development of wireless communications, heterogeneous ultra-dense networks (HUDNs) have emerged timely to meet the requirements of massive connectivity, high data rate, and low latency in the 5G era. Nevertheless, HUDN indicates large-scale and high-density scenarios, which usually lead to a high-complexity and non-convex NP-hard resource allocation problem. Graph neural network (GNN) is regarded as a promising approach to deal with the above issue. However, naive GNNs fail to model the high-order interactions among the network nodes and are prone to over-smoothing after multi-layer convolution. Hence, we construct a heterogeneous GNN with a novel high-dimensional computation structure (namely OverGNN) for the power allocation problem under HUDNs in this work. Particularly, OverGNN enables nodes directly interact with high-order neighbors and extract abundant graph topological information, which can facilitate effective feature aggregation among nodes as well as alleviate the over-smoothing problem. Based on this fact, an efficient message passing scheme for user equipments (UEs) under the same base station (BS) is developed to approximate the optimal power allocation strategy for maximizing system throughput. In addition, we propose an unsupervised approach to train the GNN model that can reduce the computation complexity of training and enhance the scalability of our proposed method. Numerical results verify the effectiveness of the proposed OverGNN and demonstrate its advantages over the benchmarks.
更多查看译文
关键词
Heterogeneous network,ultra-dense network,power allocation,GNN,high-order message passing
AI 理解论文
溯源树
样例
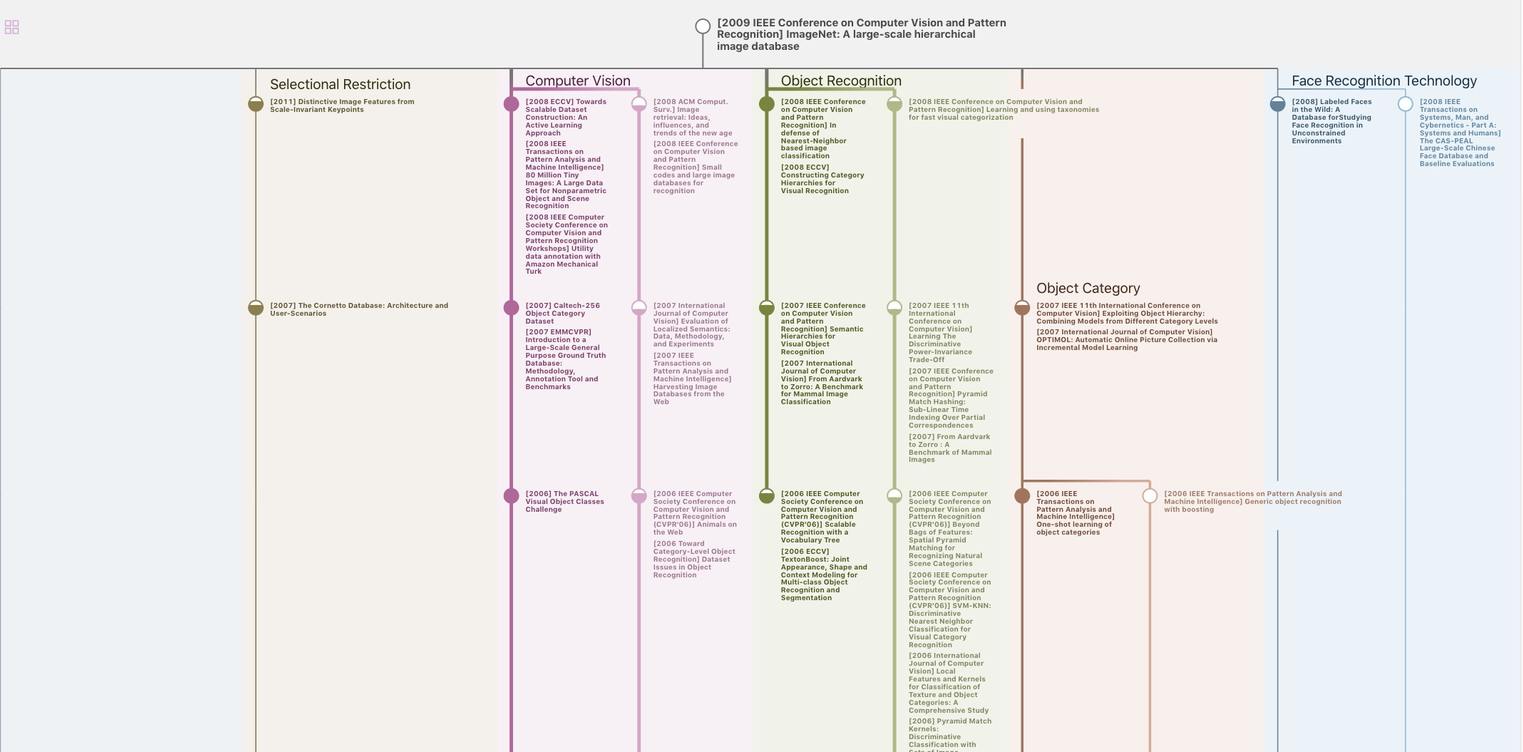
生成溯源树,研究论文发展脉络
Chat Paper
正在生成论文摘要