LiDAR-Link: Observability-Aware Probabilistic Plane-Based Extrinsic Calibration for Non-Overlapping Solid-State LiDARs
IEEE ROBOTICS AND AUTOMATION LETTERS(2024)
摘要
As solid-state LiDAR technology advances, mobile robotics and autonomous driving increasingly rely on multiple solid-state LiDARs for perception. However, limited or non-overlapping fields of view (FoV) among these sensors pose significant challenges for extrinsic calibration. Moreover, there are no quantitative indicators to evaluate calibration quality currently. To tackle these challenges, we introduce LiDAR-Link, a novel approach for calibration and evaluation, consisting of LiDAR-Bridge and LiDAR-Align. LiDAR-Bridge uses a wide-angle LiDAR to act as an intermediary, aligning point clouds from small-angle solid-state LiDARs. These small LiDARs have limited or non-overlapping FoVs, allowing us to indirectly determine the extrinsics between them. LiDAR-Align adaptively constructs a Voxel Probabilistic Plane Map to efficiently match point clouds and differentiate the contributions of various high-quality planar features. To align these point clouds, Iterated Extended Kalman Filter (IEKF) is utilized, which is based on point-to-plane residuals. The resulting covariance verifies whether point cloud alignment constraints are met and assesses calibration reliability. Furthermore, LiDAR-Align supports multi-scene joint calibration to overcome the limitations of fewer constraints caused by smaller LiDAR FoVs. We validate the accuracy of our method and the significance of observability analysis through a comprehensive set of experiments.
更多查看译文
关键词
Observability-aware,solid-state LiDAR,non-overlapping,extrinsic calibration,probabilistic plane,IEKF
AI 理解论文
溯源树
样例
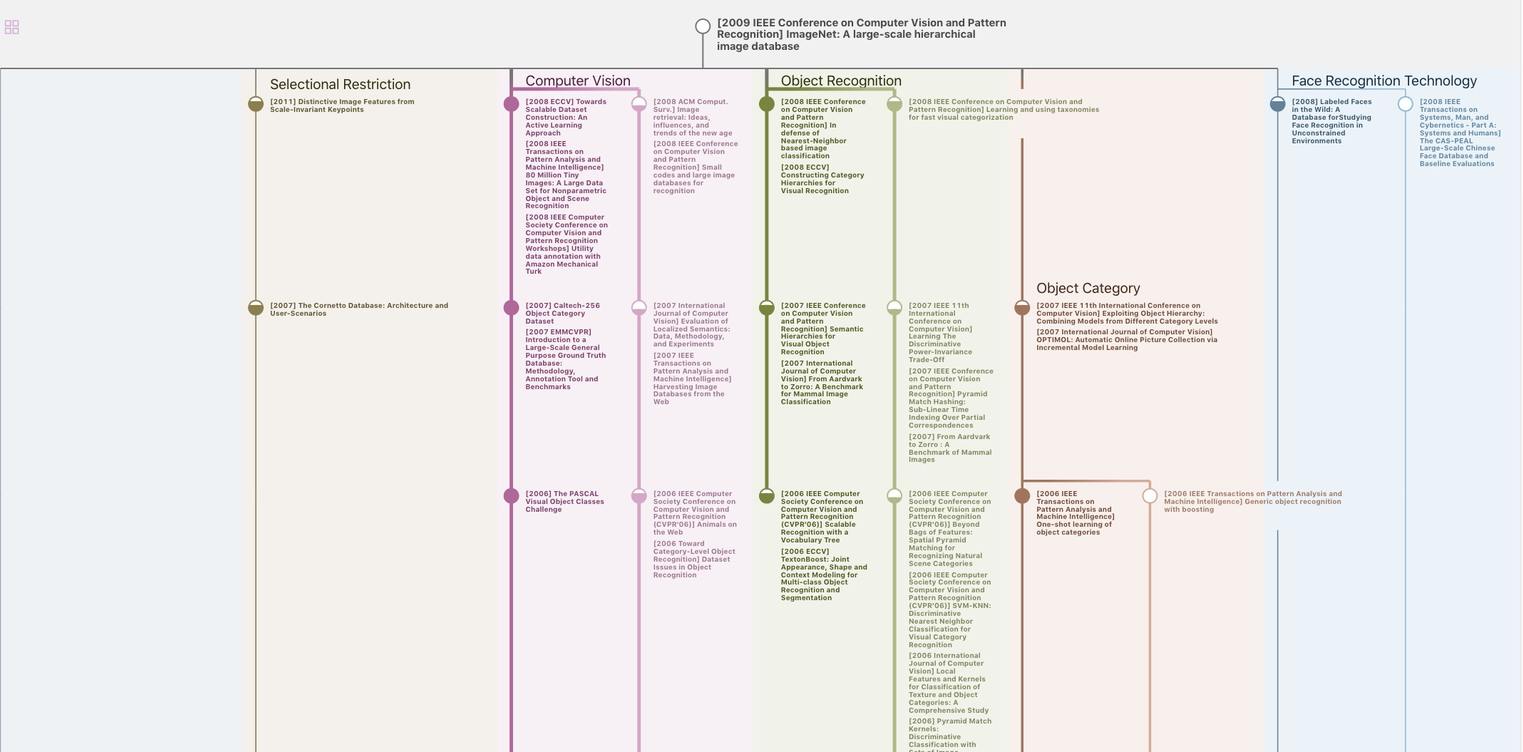
生成溯源树,研究论文发展脉络
Chat Paper
正在生成论文摘要