Distance Maximization and Defences on Deep Hashing Based Image Retrieval
2023 IEEE INTERNATIONAL CONFERENCE ON KNOWLEDGE GRAPH, ICKG(2023)
摘要
Though being remarkably efficient in computation and storage, recent research demonstrates deep hashing based image retrieval models are also vulnerable to adversarial attacks. A substantial amount of defence techniques against adversarial attacks have been developed recently, the overwhelming majority focus on adversarial training. However, adversarial defence focusing on the data side, i.e., cluster-contrasting, still remains a paucity. Albeit a pioneer work makes attempt to maximise the average distances of image clusters by anchoring predefined hash centers, it is still insufficient for a robust defence due to no optimal guarantee and over-sticking to the global average distance. Further, the laser-focus of either adversarial training or cluster contrasting of existing methods hinder them from further robustness boosting by judiciously incorporating them together. In this paper, we propose a novel distance maximization algorithm for defence on deep hashing based image retrieval systems. The model finds the optimal maximum average distance between cluster centers, and then uses a heuristic method to increase the minimum distance in the worst-case by solving a maximum Boolean satisfiability (max-SAT) problem. Our proposed distance maximization algorithm is a boosting algorithm that can be incorporated into adversarial training to enhance or boost the robustness of retrieval systems. Experiments conducted on two datasets demonstrate that our algorithm can generate clusters with a maximised average distance, while the minimum distance is also increased by up to 33% over the state-of-the-art method, and robustness is improved by up to 23%.
更多查看译文
关键词
Adversarial Machine Learning,Adversarial Defence,Deep Hashing,Image Retrieval
AI 理解论文
溯源树
样例
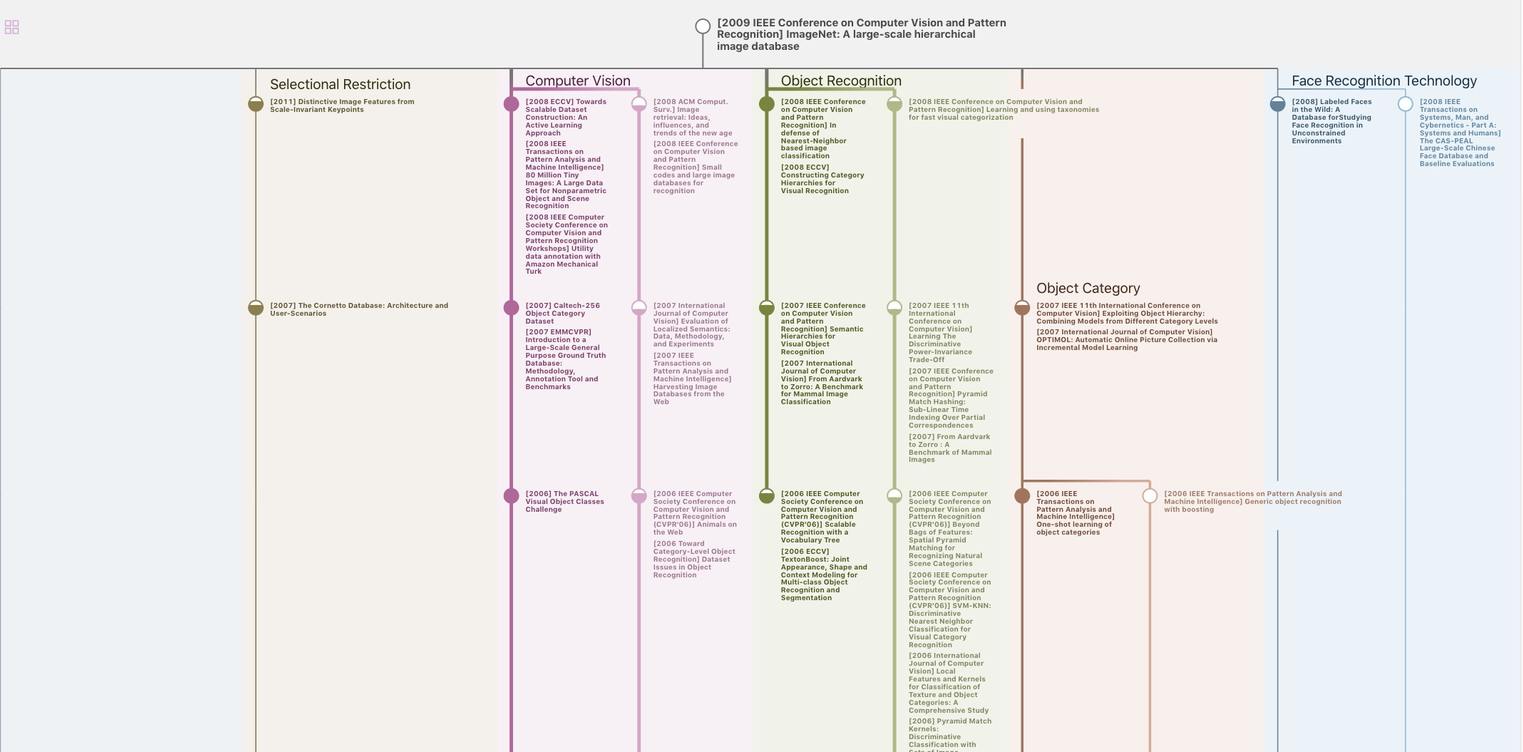
生成溯源树,研究论文发展脉络
Chat Paper
正在生成论文摘要