Ensemble reinforcement learning: A survey
Applied Soft Computing(2023)
摘要
Reinforcement Learning (RL) has emerged as a highly effective technique for addressing various scientific and applied problems. Despite its success, certain complex tasks remain challenging to be addressed solely with a single model and algorithm. In response, ensemble reinforcement learning (ERL), a promising approach that combines the benefits of both RL and ensemble learning (EL), has gained widespread popularity. ERL leverages multiple models or training algorithms to comprehensively explore the problem space and possesses strong generalization capabilities. In this study, we present a comprehensive survey on ERL to provide readers with an overview of recent advances and challenges in the field. Firstly, we provide an introduction to the background and motivation for ERL. Secondly, we conduct a detailed analysis of strategies such as model selection and combination that have been successfully implemented in ERL. Subsequently, we explore the application of ERL, summarize the datasets, and analyze the algorithms employed. Finally, we outline several open questions and discuss future research directions of ERL. By offering guidance for future scientific research and engineering applications, this survey significantly contributes to the advancement of ERL. • Strategies used to improve the performance of ERL are analyzed. • The applications of ERL in several areas are introduced and discussed. • Datasets and compared methods are described.
更多查看译文
关键词
Ensemble reinforcement learning,Reinforcement learning,Ensemble learning,Artificial neural network,Ensemble strategy
AI 理解论文
溯源树
样例
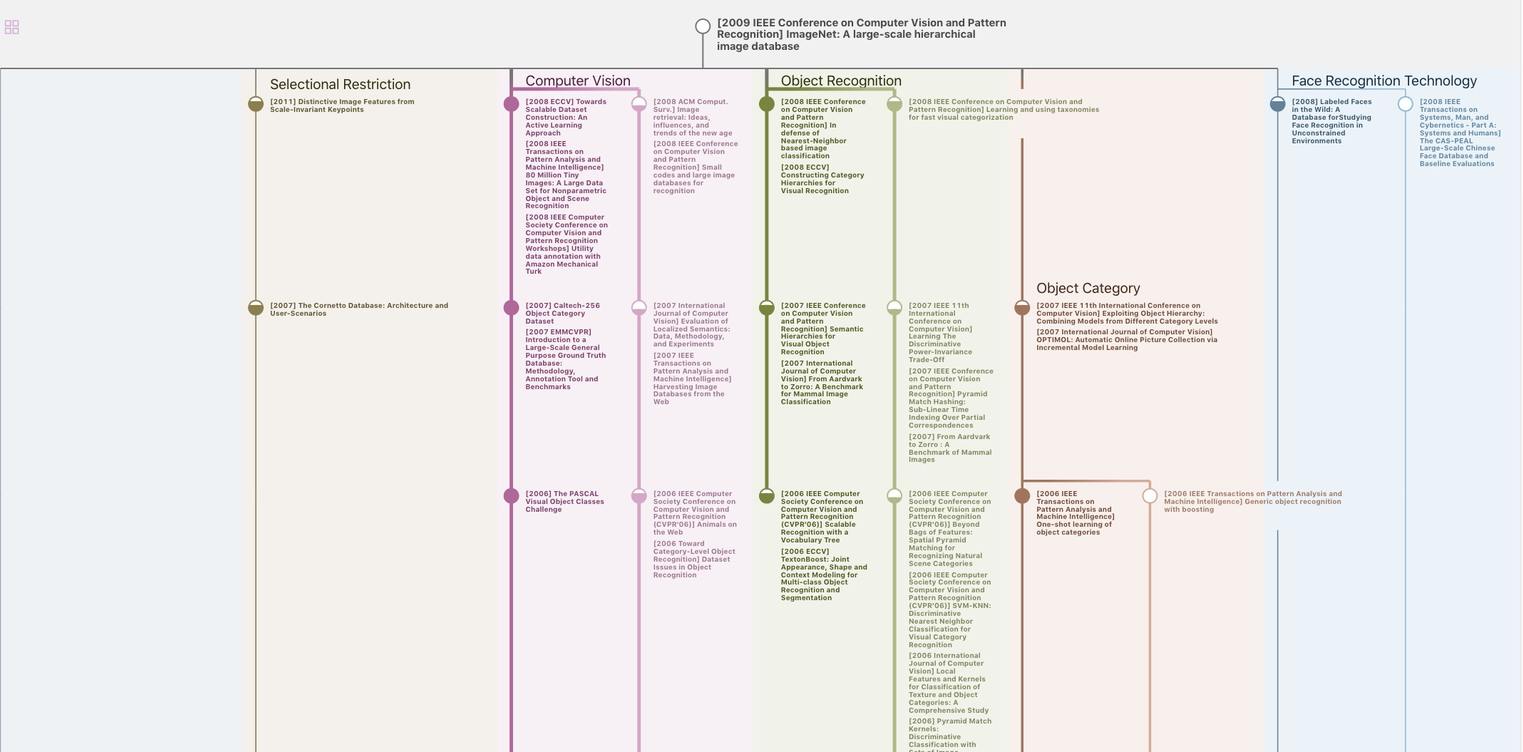
生成溯源树,研究论文发展脉络
Chat Paper
正在生成论文摘要