ALISON: Fast and Effective Stylometric Authorship Obfuscation
AAAI 2024(2024)
摘要
Authorship Attribution (AA) and Authorship Obfuscation (AO) are two competing tasks of increasing importance in privacy research. Modern AA leverages an author's consistent writing style to match a text to its author using an AA classifier. AO is the corresponding adversarial task, aiming to modify a text in such a way that its semantics are preserved, yet an AA model cannot correctly infer its authorship. To address privacy concerns raised by state-of-the-art (SOTA) AA methods,
new AO methods have been proposed but remain largely impractical to use due to their prohibitively slow training and obfuscation speed, often taking hours.
To this challenge, we propose a practical AO method, ALISON, that (1) dramatically reduces training/obfuscation time, demonstrating more than 10x faster obfuscation than SOTA AO methods, (2) achieves better obfuscation success through attacking three transformer-based AA methods on two benchmark datasets, typically performing 15% better than competing methods, (3) does not require direct signals from a target AA classifier during obfuscation, and (4) utilizes unique stylometric features, allowing sound model interpretation for explainable obfuscation. We also demonstrate that ALISON can effectively prevent four SOTA AA methods from accurately determining the authorship of ChatGPT-generated texts, all while minimally changing the original text semantics. To ensure the reproducibility of our findings, our code and data are available at: https://github.com/EricX003/ALISON.
更多查看译文
关键词
NLP: Ethics -- Bias, Fairness, Transparency & Privacy,NLP: (Large) Language Models,NLP: Applications,NLP: Interpretability, Analysis, and Evaluation of NLP Models,APP: Security
AI 理解论文
溯源树
样例
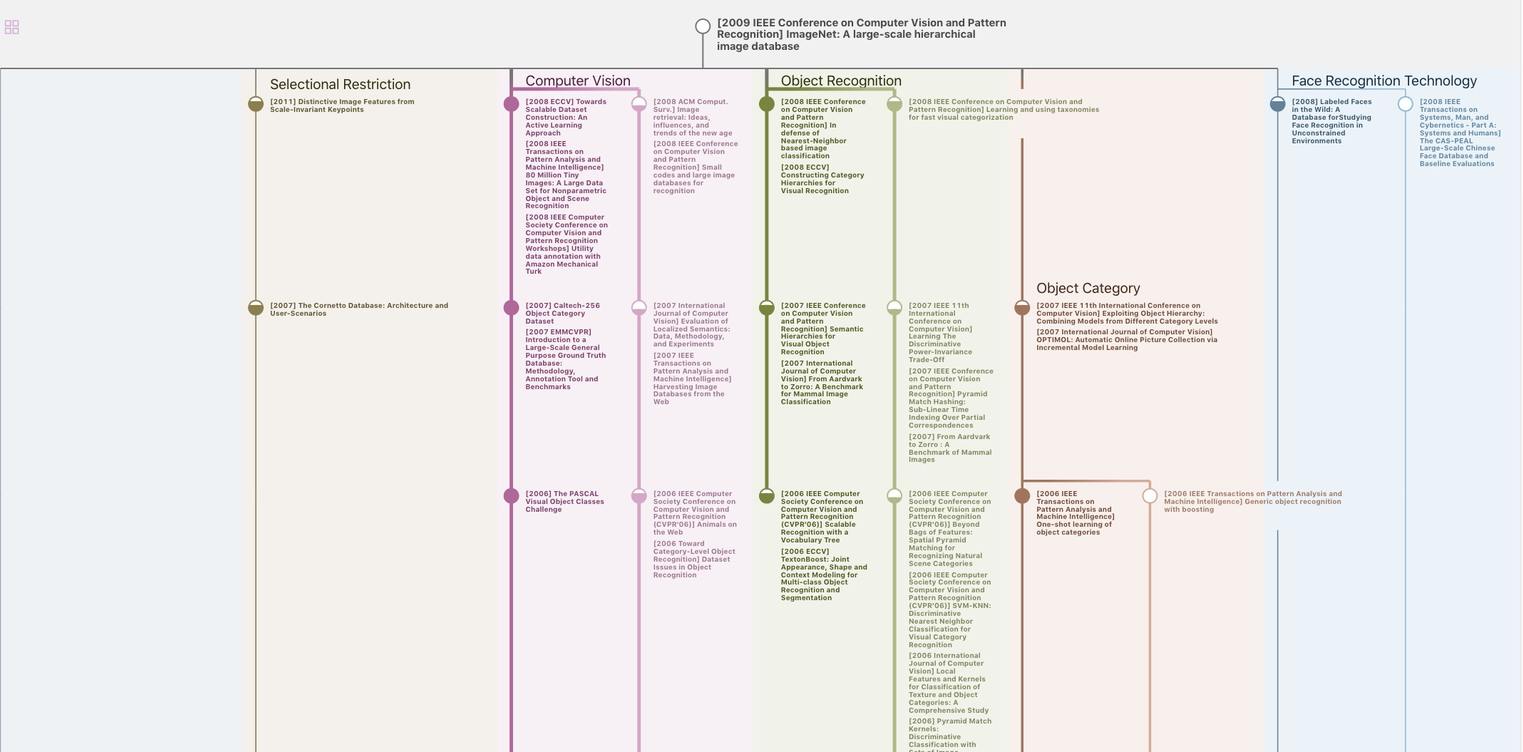
生成溯源树,研究论文发展脉络
Chat Paper
正在生成论文摘要