Profiling and Modeling of Power Characteristics of Leadership-Scale HPC System Workloads
CoRR(2024)
摘要
In the exascale era in which application behavior has large power energy
footprints, per-application job-level awareness of such impression is crucial
in taking steps towards achieving efficiency goals beyond performance, such as
energy efficiency, and sustainability.
To achieve these goals, we have developed a novel low-latency job power
profiling machine learning pipeline that can group job-level power profiles
based on their shapes as they complete. This pipeline leverages a comprehensive
feature extraction and clustering pipeline powered by a generative adversarial
network (GAN) model to handle the feature-rich time series of job-level power
measurements. The output is then used to train a classification model that can
predict whether an incoming job power profile is similar to a known group of
profiles or is completely new. With extensive evaluations, we demonstrate the
effectiveness of each component in our pipeline. Also, we provide a preliminary
analysis of the resulting clusters that depict the power profile landscape of
the Summit supercomputer from more than 60K jobs sampled from the year 2021.
更多查看译文
AI 理解论文
溯源树
样例
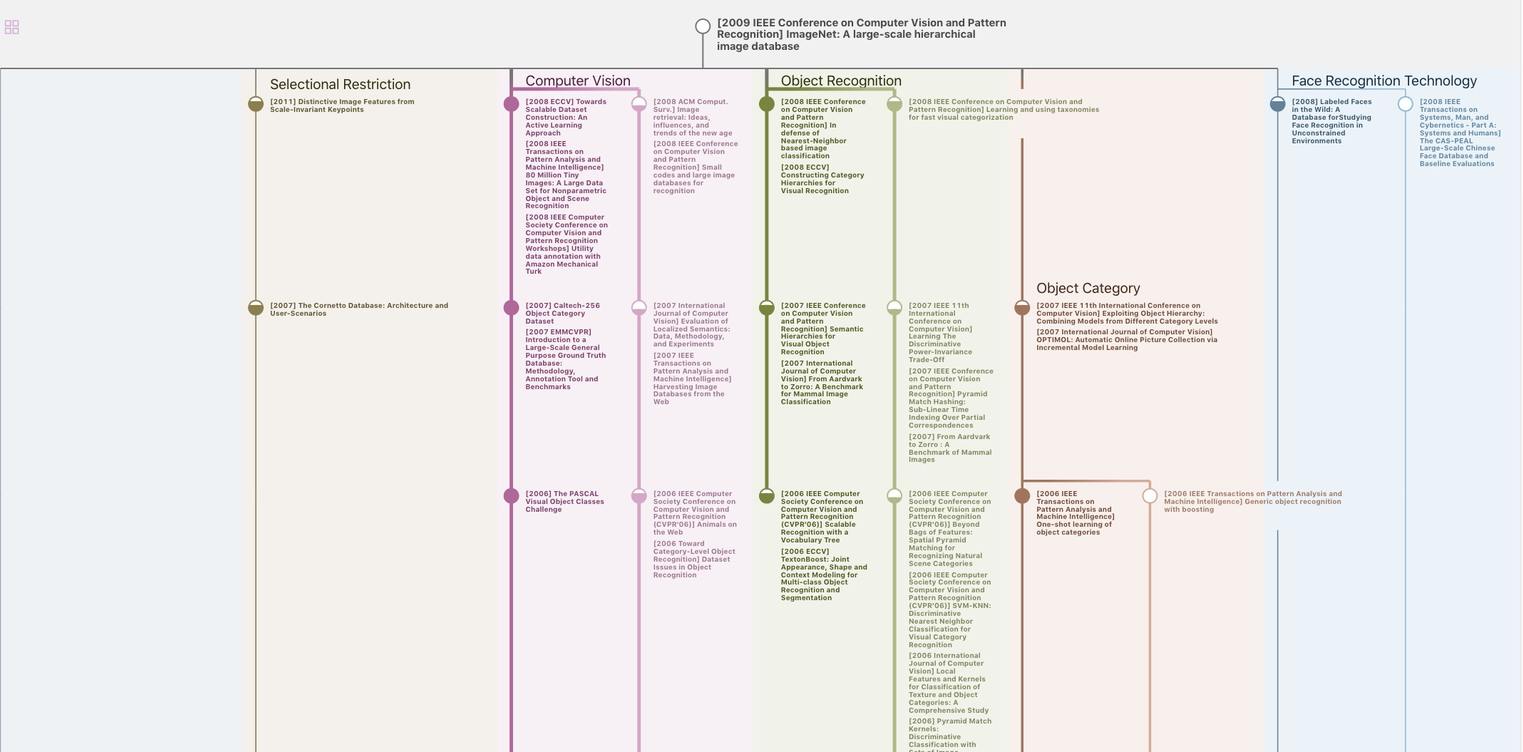
生成溯源树,研究论文发展脉络
Chat Paper
正在生成论文摘要