Efficient Non-Parametric Uncertainty Quantification for Black-Box Large Language Models and Decision Planning
CoRR(2024)
摘要
Step-by-step decision planning with large language models (LLMs) is gaining
attention in AI agent development. This paper focuses on decision planning with
uncertainty estimation to address the hallucination problem in language models.
Existing approaches are either white-box or computationally demanding, limiting
use of black-box proprietary LLMs within budgets. The paper's first
contribution is a non-parametric uncertainty quantification method for LLMs,
efficiently estimating point-wise dependencies between input-decision on the
fly with a single inference, without access to token logits. This estimator
informs the statistical interpretation of decision trustworthiness. The second
contribution outlines a systematic design for a decision-making agent,
generating actions like “turn on the bathroom light” based on user prompts
such as “take a bath”. Users will be asked to provide preferences when more
than one action has high estimated point-wise dependencies. In conclusion, our
uncertainty estimation and decision-making agent design offer a cost-efficient
approach for AI agent development.
更多查看译文
AI 理解论文
溯源树
样例
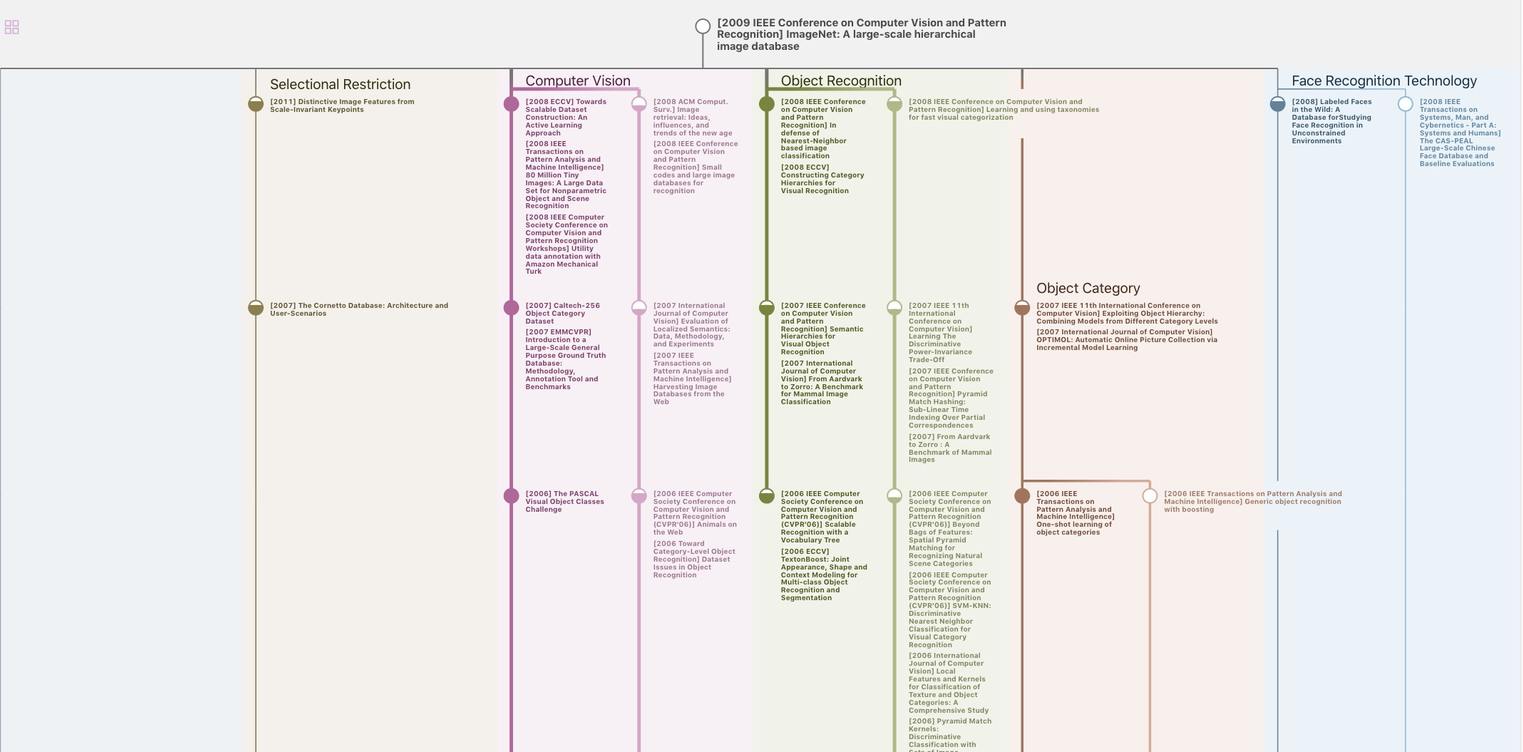
生成溯源树,研究论文发展脉络
Chat Paper
正在生成论文摘要