Development and multicenter validation of deep convolutional neural network–based detection of colorectal cancer on abdominal CT
European Radiology(2024)
摘要
This study aims to develop computer-aided detection (CAD) for colorectal cancer (CRC) using abdominal CT based on a deep convolutional neural network. This retrospective study included consecutive patients with colorectal adenocarcinoma who underwent abdominal CT before CRC resection surgery (training set = 379, test set = 103). We customized the 3D U-Net of nnU-Net (CUNET) for CRC detection, which was trained with fivefold cross-validation using annotated CT images. CUNET was validated using datasets covering various clinical situations and institutions: an internal test set (n = 103), internal patients with CRC first determined by CT (n = 54) and asymptomatic CRC (n = 51), and an external validation set from two institutions (n = 60). During each validation, data from the healthy population were added (internal = 60; external = 130). CUNET was compared with other deep CNNs: residual U-Net and EfficientDet. The CAD performances were evaluated using per-CRC sensitivity (true positive/all CRCs), free-response receiver operating characteristic (FROC), and jackknife alternative FROC (JAFROC) curves. CUNET showed a higher maximum per-CRC sensitivity than residual U-Net and EfficientDet (internal test set 91.3
更多查看译文
关键词
Colorectal neoplasms,Tomography (X-ray computed),Radiographic image interpretation (Computer-assisted),Deep learning,Retrospective studies
AI 理解论文
溯源树
样例
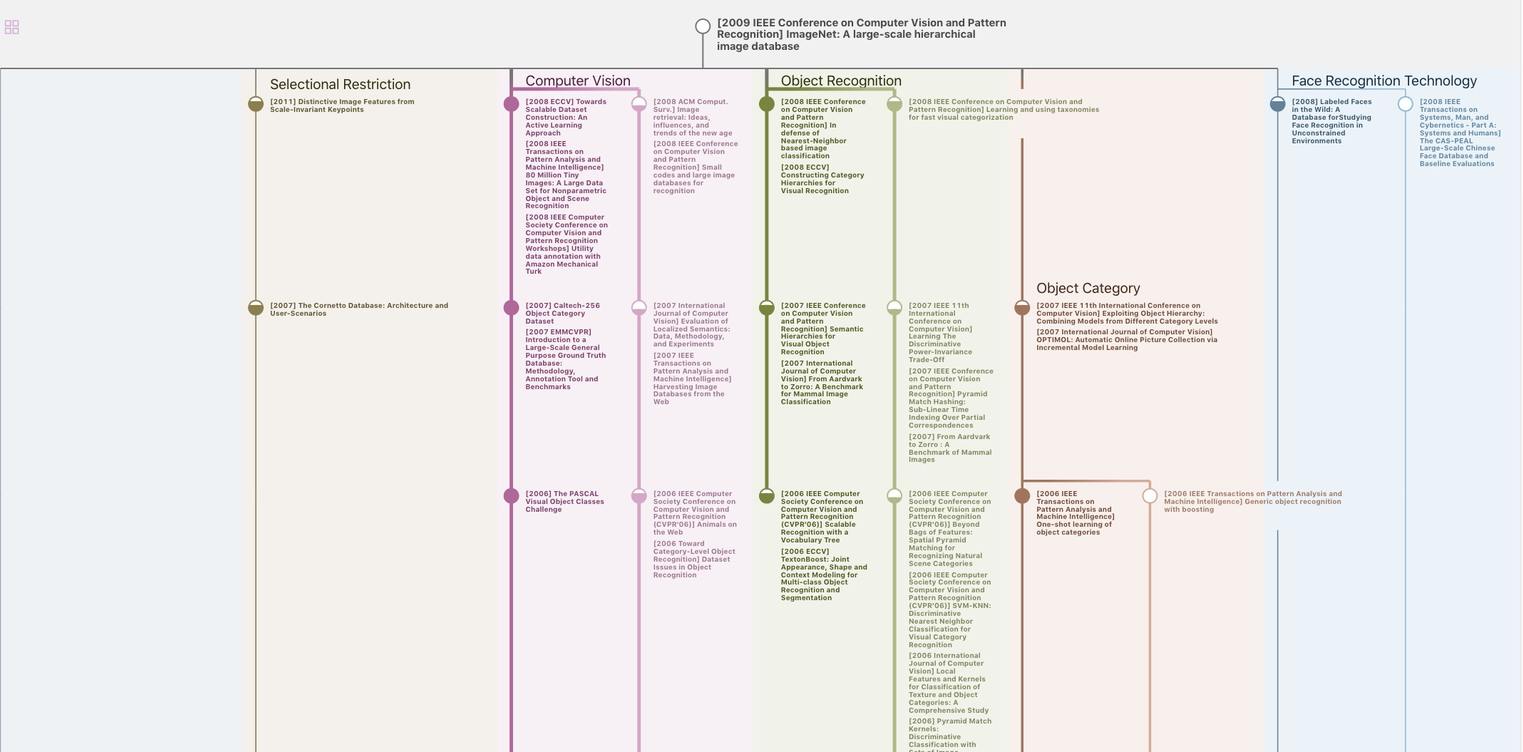
生成溯源树,研究论文发展脉络
Chat Paper
正在生成论文摘要