EPolar-UNet: An edge-attending polar UNet for automatic medical image segmentation with small datasets
MEDICAL PHYSICS(2024)
摘要
BackgroundMedical image segmentation is one of the most key steps in computer-aided clinical diagnosis, geometric characterization, measurement, image registration, and so forth. Convolutional neural networks especially UNet and its variants have been successfully used in many medical image segmentation tasks. However, the results are limited by the deficiency in extracting high resolution edge information because of the design of the skip connections in UNet and the need for large available datasets.PurposeIn this paper, we proposed an edge-attending polar UNet (EPolar-UNet), which was trained on the polar coordinate system instead of classic Cartesian coordinate system with an edge-attending construction in skip connection path.MethodsEPolar-UNet extracted the location information from an eight-stacked hourglass network as the pole for polar transformation and extracted the boundary cues from an edge-attending UNet, which consisted of a deconvolution layer and a subtraction operation.ResultsWe evaluated the performance of EPolar-UNet across three imaging modalities for different segmentation tasks: CVC-ClinicDB dataset for polyp, ISIC-2018 dataset for skin lesion, and our private ultrasound dataset for liver tumor segmentation. Our proposed model outperformed state-of-the-art models on all three datasets and needed only 30%-60% of training data compared with the benchmark UNet model to achieve similar performances for medical image segmentation tasks.ConclusionsWe proposed an end-to-end EPolar-UNet for automatic medical image segmentation and showed good performance on small datasets, which was critical in the field of medical image segmentation.
更多查看译文
关键词
edge-attending,polar transformation,segmentation
AI 理解论文
溯源树
样例
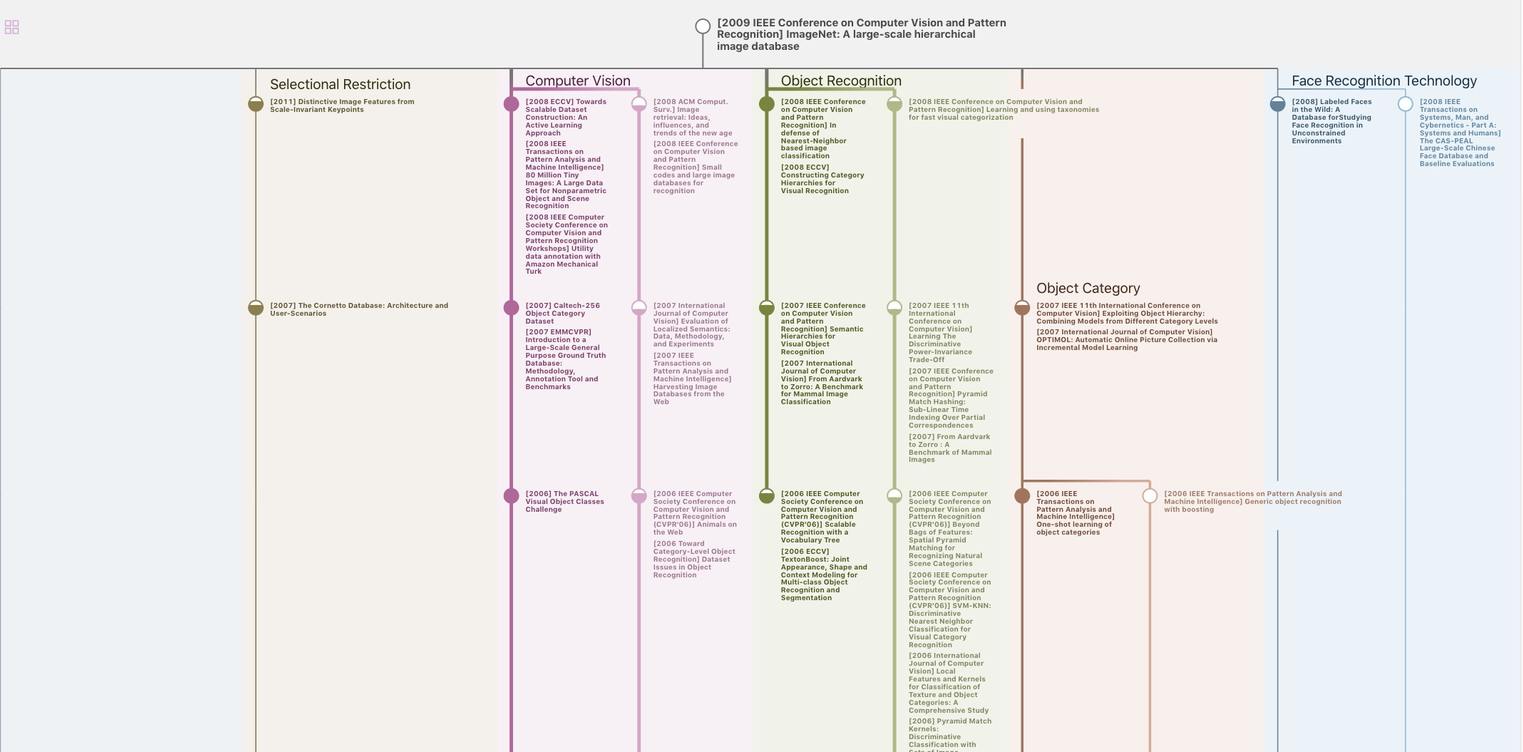
生成溯源树,研究论文发展脉络
Chat Paper
正在生成论文摘要