Simulation Optimization with Multiple Attempts.
Winter Simulation Conference(2023)
摘要
Simulation optimization is a widely utilized approach that allows decision-makers to test various decision variable settings in simulators before implementing a final recommended action on the real systems. In some real-world scenarios, the recommended action can be executed multiple times and the performance is evaluated as the best one among these multiple attempts. In this paper, we introduce such simulation optimization problems with multiple attempts and provide insights into the problem through comparison to risk-averse decision-making problems. We propose a surrogate-assisted algorithm based on the Gaussian process model and the upper confidence bound criterion for efficiently solving such problems. We demonstrate the efficiency and effectiveness of the proposed approach with several numerical examples.
更多查看译文
关键词
Multiple Attempts,Simulation Optimization,Decision-making,Optimization Problem,Process Model,Decision Variables,Gaussian Process,Upper Confidence,Gaussian Process Model,Upper Bound,Function Tests,Posterior Probability,Alternative Models,Black Box,Risk Measures,Design Space,Mean Function,Stochastic Optimization,Design Points,Covariance Function,Upper Confidence Bound,Traditional Problem,Bayesian Optimization,Stochastic Simulations,Benchmark Methods,Logit Transformation,Benchmark Algorithms,Maximum Expectation,Sublinear,Bayesian Optimization Algorithm
AI 理解论文
溯源树
样例
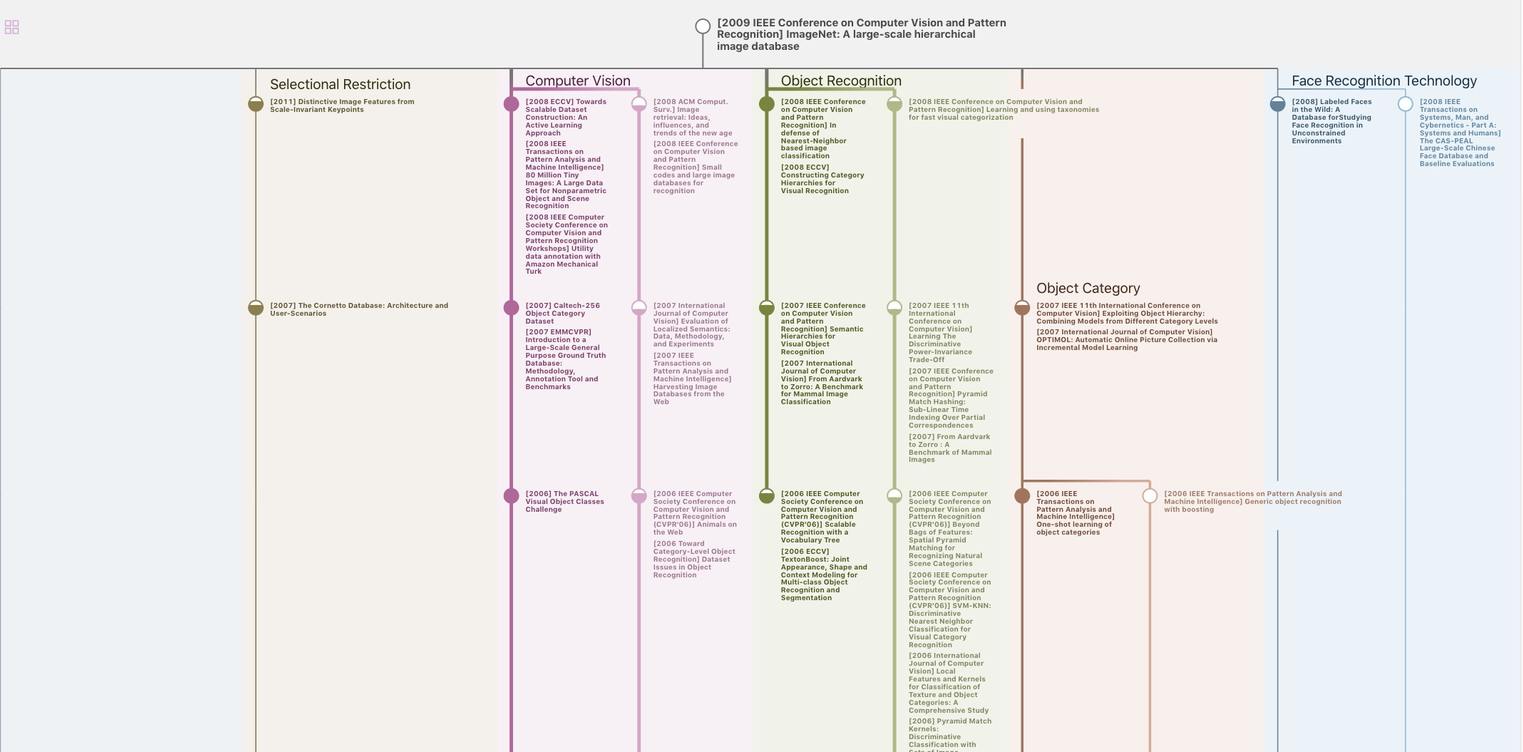
生成溯源树,研究论文发展脉络
Chat Paper
正在生成论文摘要