MIMRD: An Enhanced Masked Image Modeling Reverse Distillation for Anomaly Detection
2023 International Conference on New Trends in Computational Intelligence (NTCI)(2023)
摘要
Anomaly detection is a meaningful and important research topic. Currently, unsupervised methods for anomaly detection demonstrate exceptional performance. Reverse distillation (RD) is an effective approach that ensures minimal latency while demonstrating overly strong generalization ability, causing abnormal features to be generalized as normal features in anomaly detection tasks. However, there may be instances where the model's generalization ability becomes overly dominant. To address this problem, we propose an enhanced masked image modeling reverse distillation (MIMRD). In this paper, we establish a state-of-the-art benchmark on the challenging MVTec dataset for both identifying and localizing anomalies. This model reconstructs the features with pseudo anomalies and enhance the difference in anomalousness through utilization of projection layer. Then, the student model regresses to the normal features generated by the teacher model. From the experimental results, our method has achieved competitive results without a significant increase in inference time.
更多查看译文
关键词
unsupervised learning,anomaly detection,reverse distillation,teacher-student model
AI 理解论文
溯源树
样例
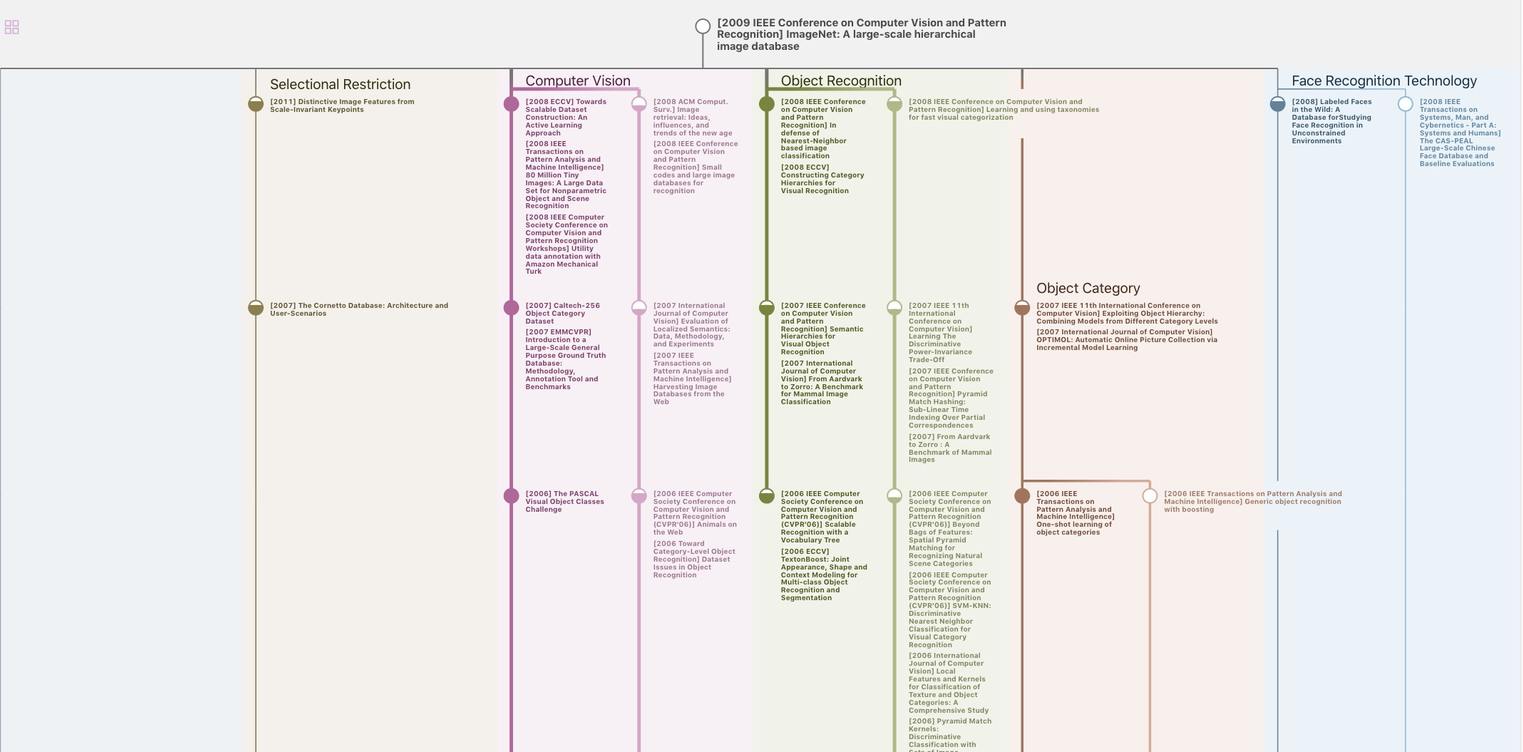
生成溯源树,研究论文发展脉络
Chat Paper
正在生成论文摘要