O2 supplementation disambiguation in clinical narratives to support retrospective COVID-19 studies
BMC Medical Informatics and Decision Making(2024)
摘要
Background Oxygen saturation, a key indicator of COVID-19 severity, poses challenges, especially in cases of silent hypoxemia. Electronic health records (EHRs) often contain supplemental oxygen information within clinical narratives. Streamlining patient identification based on oxygen levels is crucial for COVID-19 research, underscoring the need for automated classifiers in discharge summaries to ease the manual review burden on physicians. Method We analysed text lines extracted from anonymised COVID-19 patient discharge summaries in German to perform a binary classification task, differentiating patients who received oxygen supplementation and those who did not. Various machine learning (ML) algorithms, including classical ML to deep learning (DL) models, were compared. Classifier decisions were explained using Local Interpretable Model-agnostic Explanations (LIME), which visualize the model decisions. Result Classical ML to DL models achieved comparable performance in classification, with an F-measure varying between 0.942 and 0.955, whereas the classical ML approaches were faster. Visualisation of embedding representation of input data reveals notable variations in the encoding patterns between classic and DL encoders. Furthermore, LIME explanations provide insights into the most relevant features at token level that contribute to these observed differences. Conclusion Despite a general tendency towards deep learning, these use cases show that classical approaches yield comparable results at lower computational cost. Model prediction explanations using LIME in textual and visual layouts provided a qualitative explanation for the model performance.
更多查看译文
关键词
Natural language processing,Machine learning,Deep learning,Electronic health records,COVID-19
AI 理解论文
溯源树
样例
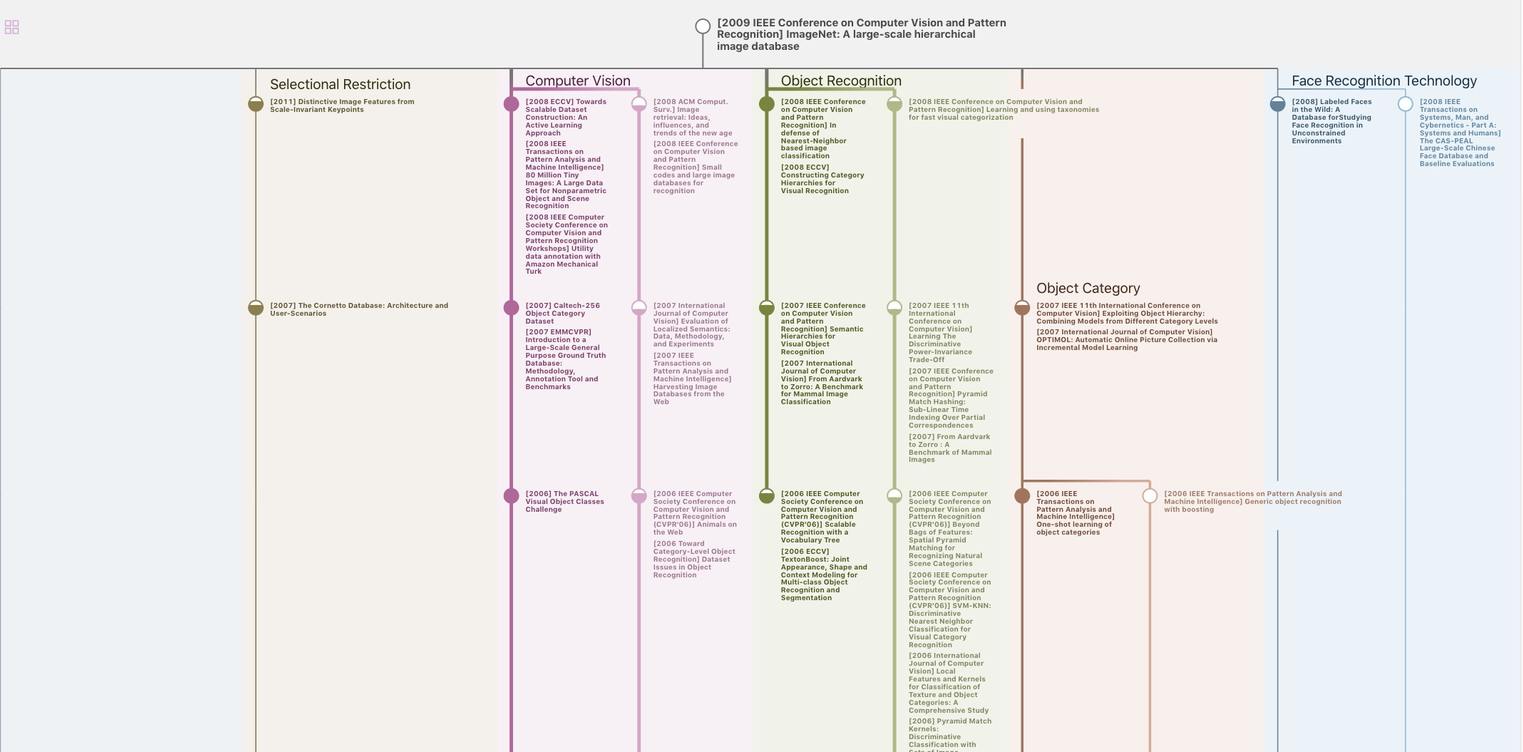
生成溯源树,研究论文发展脉络
Chat Paper
正在生成论文摘要