Classification of executive functioning performance post-longitudinal tDCS using functional connectivity and machine learning methods
2023 IEEE 20th India Council International Conference (INDICON)(2024)
摘要
Executive functioning is a cognitive process that enables humans to plan,
organize, and regulate their behavior in a goal-directed manner. Understanding
and classifying the changes in executive functioning after longitudinal
interventions (like transcranial direct current stimulation (tDCS)) has not
been explored in the literature. This study employs functional connectivity and
machine learning algorithms to classify executive functioning performance
post-tDCS. Fifty subjects were divided into experimental and placebo control
groups. EEG data was collected while subjects performed an executive
functioning task on Day 1. The experimental group received tDCS during task
training from Day 2 to Day 8, while the control group received sham tDCS. On
Day 10, subjects repeated the tasks specified on Day 1. Different functional
connectivity metrics were extracted from EEG data and eventually used for
classifying executive functioning performance using different machine learning
algorithms. Results revealed that a novel combination of partial directed
coherence and multi-layer perceptron (along with recursive feature elimination)
resulted in a high classification accuracy of 95.44
implications of our results in developing real-time neurofeedback systems for
assessing and enhancing executive functioning performance post-tDCS
administration.
更多查看译文
关键词
Functional connectivity,transcranial direct current stimulation,magnitude squared coherence,partial directed coherence,wavelet coherence,Electroencephalography,multi-layer perceptron
AI 理解论文
溯源树
样例
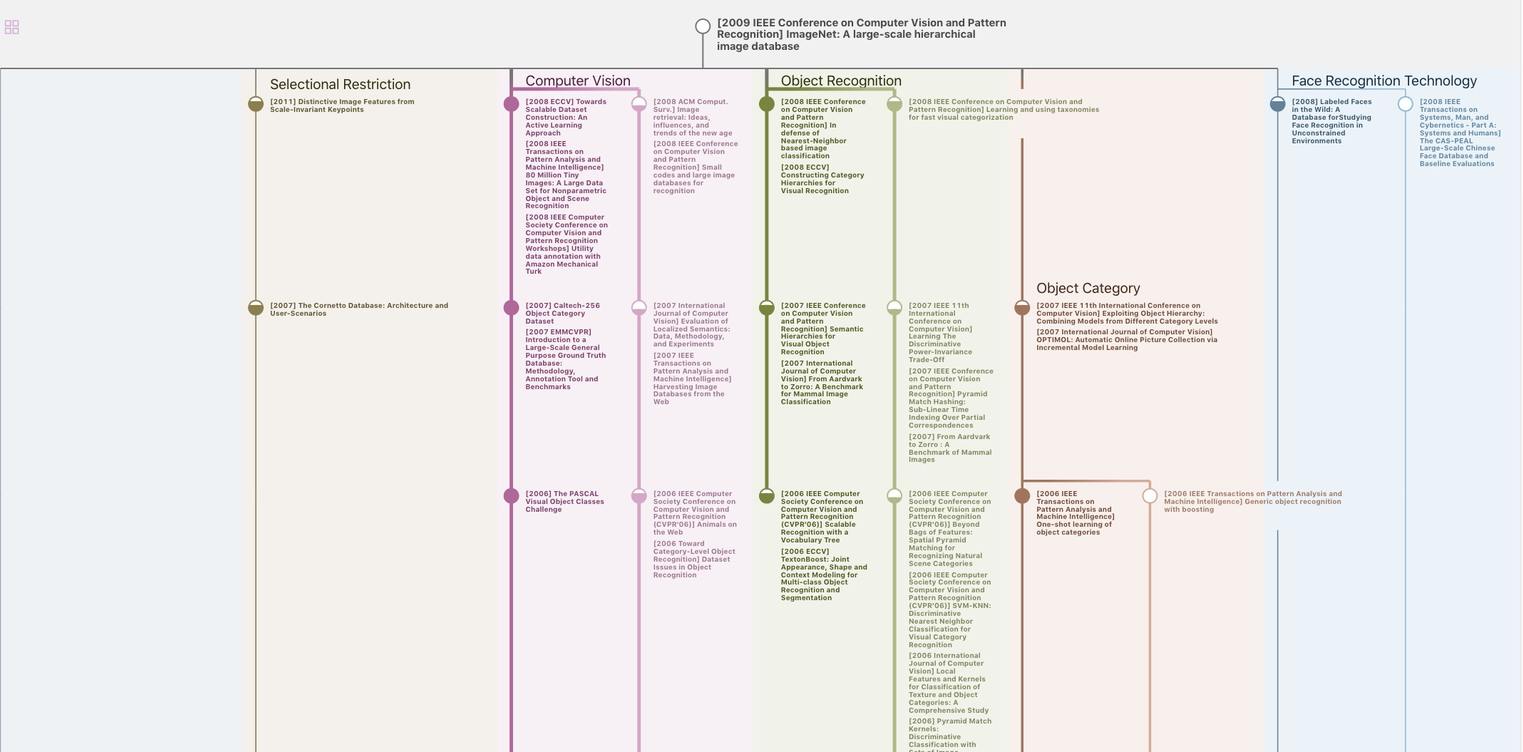
生成溯源树,研究论文发展脉络
Chat Paper
正在生成论文摘要