A Hybrid Machine Learning Framework for Predicting Hydrogen Storage Capacities: Unsupervised Feature Learning with Deep Neural Networks
arxiv(2024)
摘要
In this study, we present a sophisticated hybrid machine-learning framework
that significantly improves the accuracy of predicting hydrogen storage
capacities in metal hydrides. This is a critical challenge due to the scarcity
of experimental data and the complexity of high-dimensional feature spaces. Our
approach employs the power of unsupervised learning through the use of a
state-of-the-art autoencoder. This autoencoder is trained on elemental
descriptors obtained from Mendeleev software, enabling the extraction of a
meaningful and lower dimensional latent space from the input data. This latent
representation serves as the basis for our deep multi-layer perceptron (MLP)
model, which consists of five layers and shows good precision in predicting
hydrogen storage capacities. Furthermore, our results show very good agreement
with the results of density functional theory (DFT). In addition to addressing
the limitations caused by limited and unevenly distributed data in the field of
hydrogen storage materials, we also focus on discovering new materials that
show promising opportunities for hydrogen storage. These materials were
identified using both feature-based approaches and predictions generated by a
large language model. Finally, our investigation into the effectiveness of
transferring weights from the autoencoder to the MLP, in addition to the latent
features, suggests that while this strategy slightly improves model performance
indicated by a slightly higher R^2 value and lower RMSE, it emphasizes the
intricate challenge of adapting pre-trained weights for specific supervised
tasks.
更多查看译文
AI 理解论文
溯源树
样例
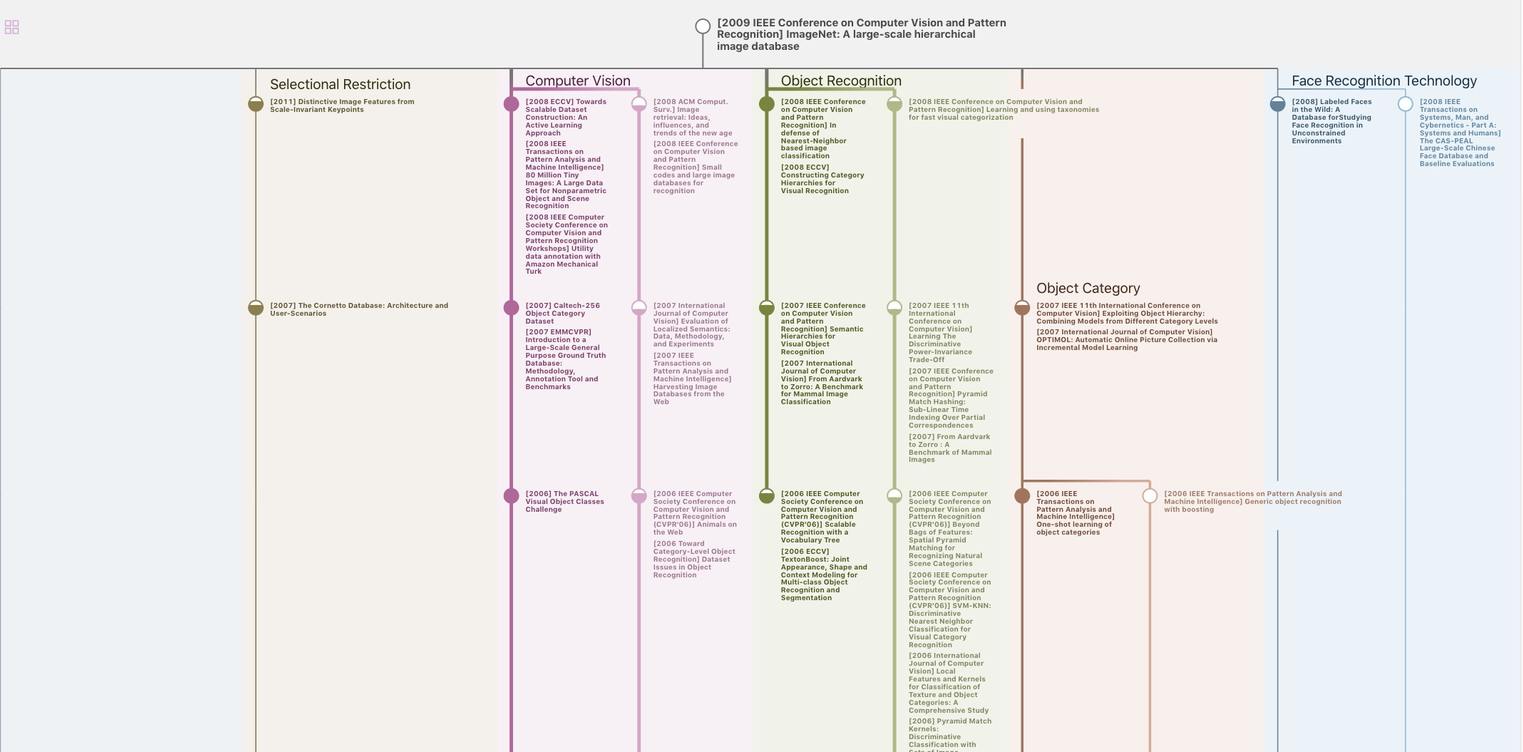
生成溯源树,研究论文发展脉络
Chat Paper
正在生成论文摘要