Marginal treatment effects in the absence of instrumental variables
arxiv(2024)
摘要
We propose a method for defining, identifying, and estimating the marginal
treatment effect (MTE) without imposing the instrumental variable (IV)
assumptions of independence, exclusion, and separability (or monotonicity).
Under a new definition of the MTE based on reduced-form treatment error that is
statistically independent of the covariates, we find that the relationship
between the MTE and standard treatment parameters holds in the absence of IVs.
We provide a set of sufficient conditions ensuring the identification of the
defined MTE in an environment of essential heterogeneity. The key conditions
include a linear restriction on potential outcome regression functions, a
nonlinear restriction on the propensity score, and a conditional mean
independence restriction that will lead to additive separability. We prove this
identification using the notion of semiparametric identification based on
functional form. We suggest consistent semiparametric estimation procedures,
and provide an empirical application for the Head Start program to illustrate
the usefulness of the proposed method in analyzing heterogenous causal effects
when IVs are elusive.
更多查看译文
AI 理解论文
溯源树
样例
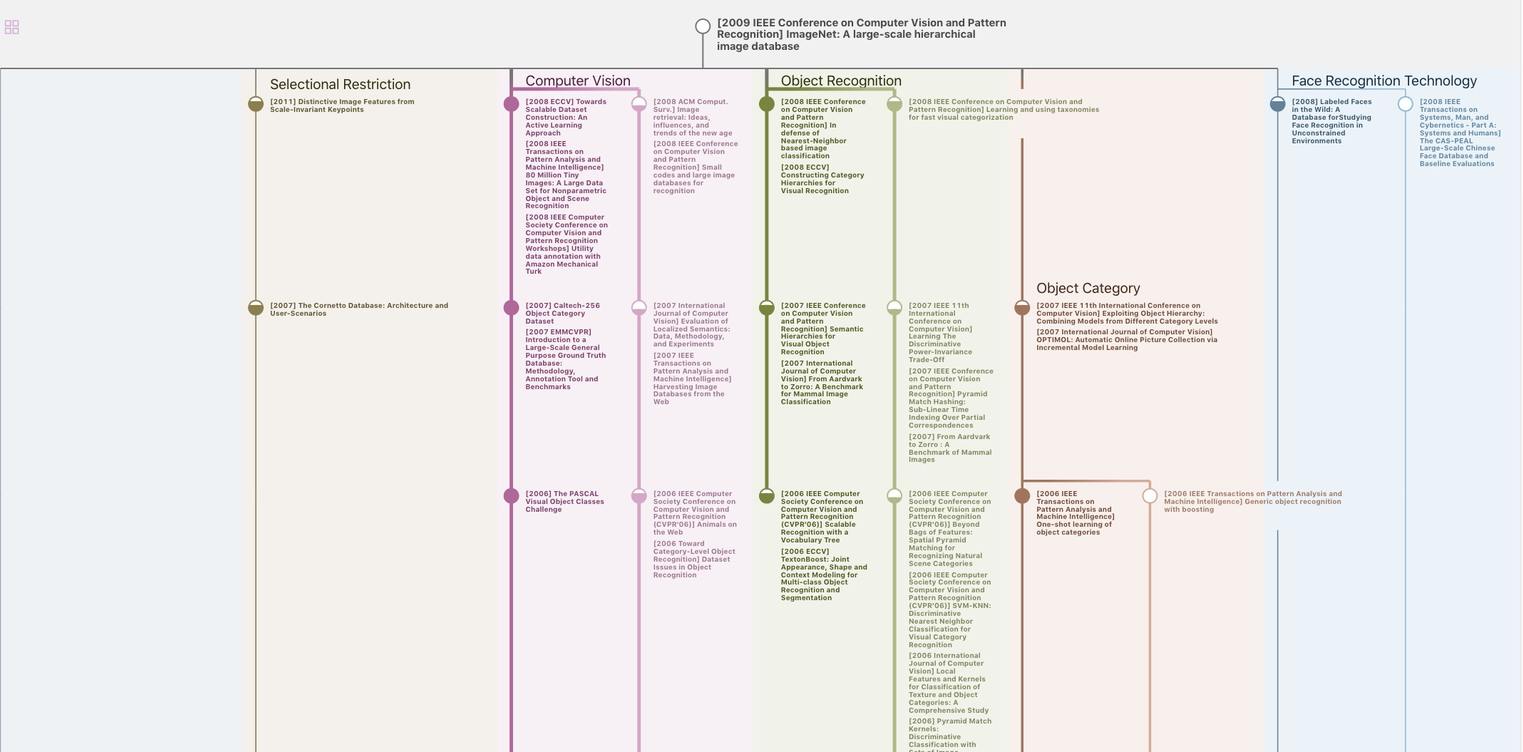
生成溯源树,研究论文发展脉络
Chat Paper
正在生成论文摘要