Preference learning and multiple criteria decision aiding: differences, commonalities, and synergies–part I
4OR(2024)
摘要
Multiple criteria decision aiding (MCDA) and preference learning (PL) are established research fields, which have different roots, developed in different communities – the former in the decision sciences and operations research, the latter in AI and machine learning – and have their own agendas in terms of problem setting, assumptions, and criteria of success. In spite of this, they share the major goal of constructing practically useful decision models that either support humans in the task of choosing the best, classifying, or ranking alternatives from a given set, or even automate decision-making by acting autonomously on behalf of the human. Therefore, MCDA and PL can complement and mutually benefit from each other, a potential that has been exhausted only to some extent so far. By elaborating on the connection between MCDA and PL in more depth, our goal is to stimulate further research at the junction of these two fields. To this end, we first review both methodologies, MCDA in this part of the paper and PL in the second part, with the intention of highlighting their most common elements. In the second part, we then compare both methodologies in a systematic way and give an overview of existing work on combining PL and MCDA.
更多查看译文
关键词
Preference learning,Preference modelling,Multiple criteria decision aiding,Multiple criteria decision making,Machine Learning,68T05,90B50,90B32,91B06,91B08
AI 理解论文
溯源树
样例
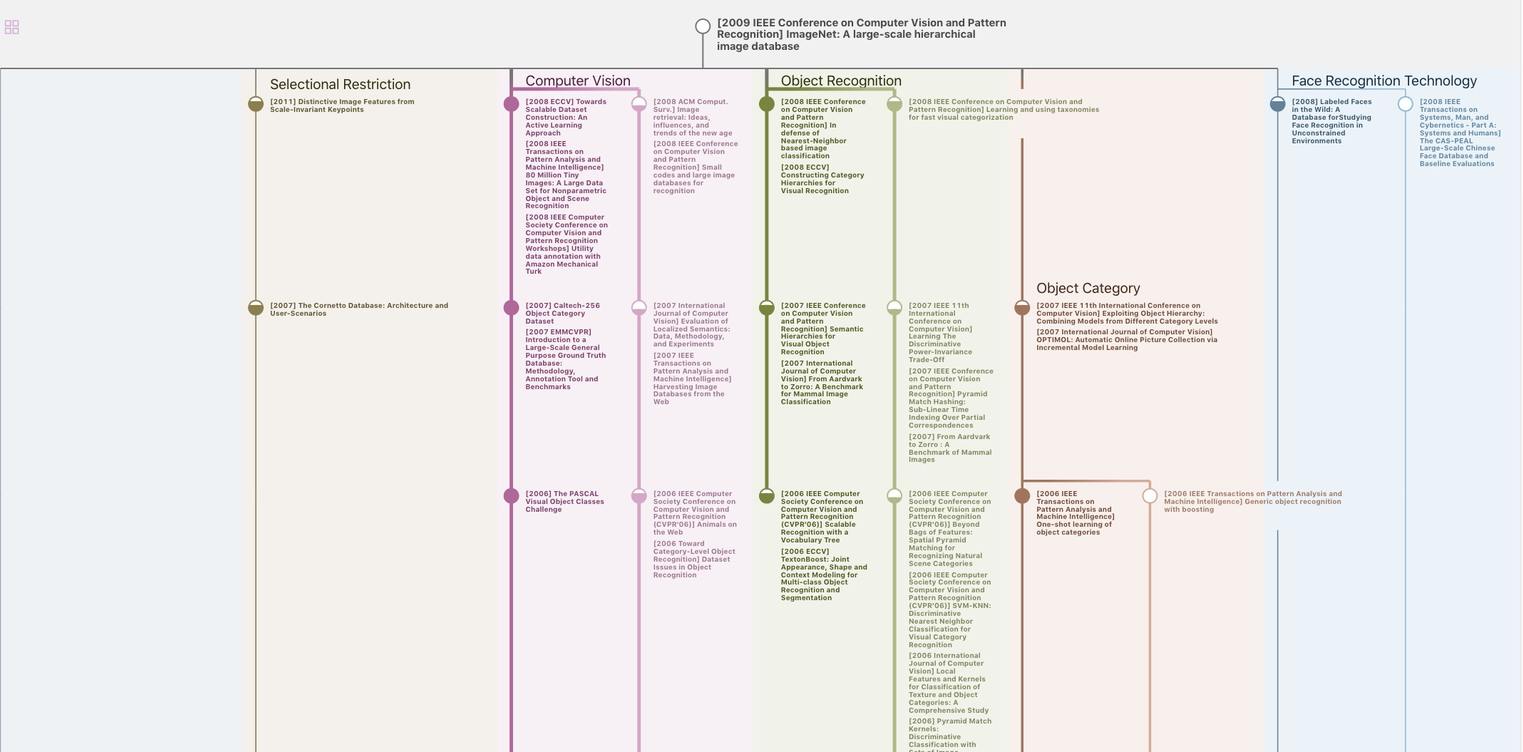
生成溯源树,研究论文发展脉络
Chat Paper
正在生成论文摘要