Remaining useful life prediction towards cycling stability of organic electrochemical transistors
MATERIALS RESEARCH EXPRESS(2024)
摘要
Organic electrochemical transistors (OECTs) show abundant potential in biosensors, artificial neuromorphic systems, brain-machine interfaces, etc With the fast development of novel functional materials and new device structures, OECTs with high transconductance (g(m) > mS) and good cycling stabilities (> 10,000 cycles) have been developed. While stability characterization is always time-consuming, to accelerate the development and commercialization of OECTs, tools for stability prediction are urgently needed. In this paper, OECTs with good cycling stabilities are realized by minimizing the gate voltage amplitude during cycling, while a remaining useful life (RUL) prediction framework for OECTs is proposed. Specifically, OECTs based on p(g2T-T) show tremendously enhanced stability which exhibits only 46.1% on-current (I-ON) and 33.2% peak g(m) decreases after 80,000 cycles (53 min). Then, RUL prediction is proposed based on the run-to-failure (RtF) aging tests (cycling stability test of OECTs). By selecting two aging parameters (I-ON and peak g(m)) as health indicators (HI), a novel multi-scale feature fusion (MFF) method for RUL prediction is proposed, which consists of a long short-term memory (LSTM) neural network based multi-scale feature generator (MFG) module for feature extraction and an attention-based feature fusion (AFF) module for feature fusion. Consequently, richer effective information is utilized to improve the prediction performance, where the experimental results show the superiority of the proposed framework on multiple OECTs in RUL prediction tasks. Therefore, by introducing such a powerful framework for the evaluation of the lifetime of OECTs, further optimization of materials, devices, and integrated systems relevant to OECTs will be stimulated. Moreover, this tool can also be extended to other relevant bioelectronics.
更多查看译文
关键词
organic electrochemical transistor,RUL prediction,cycling aging test,data-driven methodology
AI 理解论文
溯源树
样例
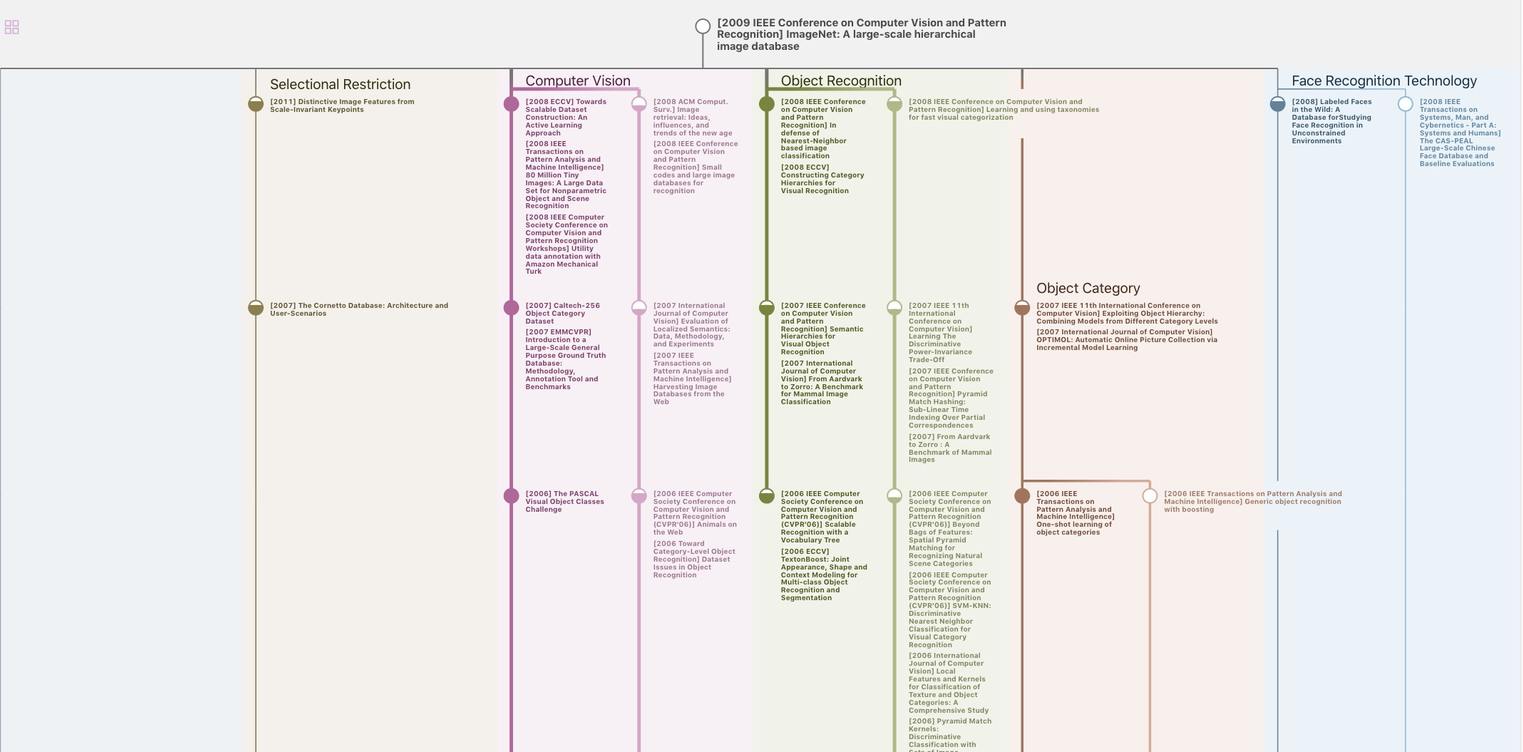
生成溯源树,研究论文发展脉络
Chat Paper
正在生成论文摘要