Mitigate Gender Bias in Construction: Fusion of Deep Reinforcement Learning -Based Contract Theory and Blockchain
2023 IEEE INTERNATIONAL CONFERENCE ON BLOCKCHAIN, BLOCKCHAIN(2023)
摘要
With the remarkable progress in teleoperation, physical litness-based gender bias has become negligible within the construction sector. Nonetheless, the labor market remains male -dominated, posing tremendous unfairness toward females. In light of this, we developed a two-phase recruitment framework that utilizes blockchain, zero-knowledge proofs (ZKPs), deep reinforcement learning (DRL), and contract theory, aiming to enhance fairness, transparency, and automation. First, we devised a resume screening approach independent of gender to ensure fairness and alleviate gender bias in candidate assessment, by leveraging blockchain and ZKPs. In the second phase, we introduce a recruitment process that combines blockchain and DRL-based contract theory. This integration successfully mitigates gender bias that may arise from the self-disclosure property of contract theory. To evaluate the effectiveness of our proposed approach, we conducted comprehensive simulations from various dimensions. The results demonstrated the robustness and superiority of our method.
更多查看译文
关键词
gender bias,blokchain,zero-knowledge proofs,deep reinforcement learning,contract theory
AI 理解论文
溯源树
样例
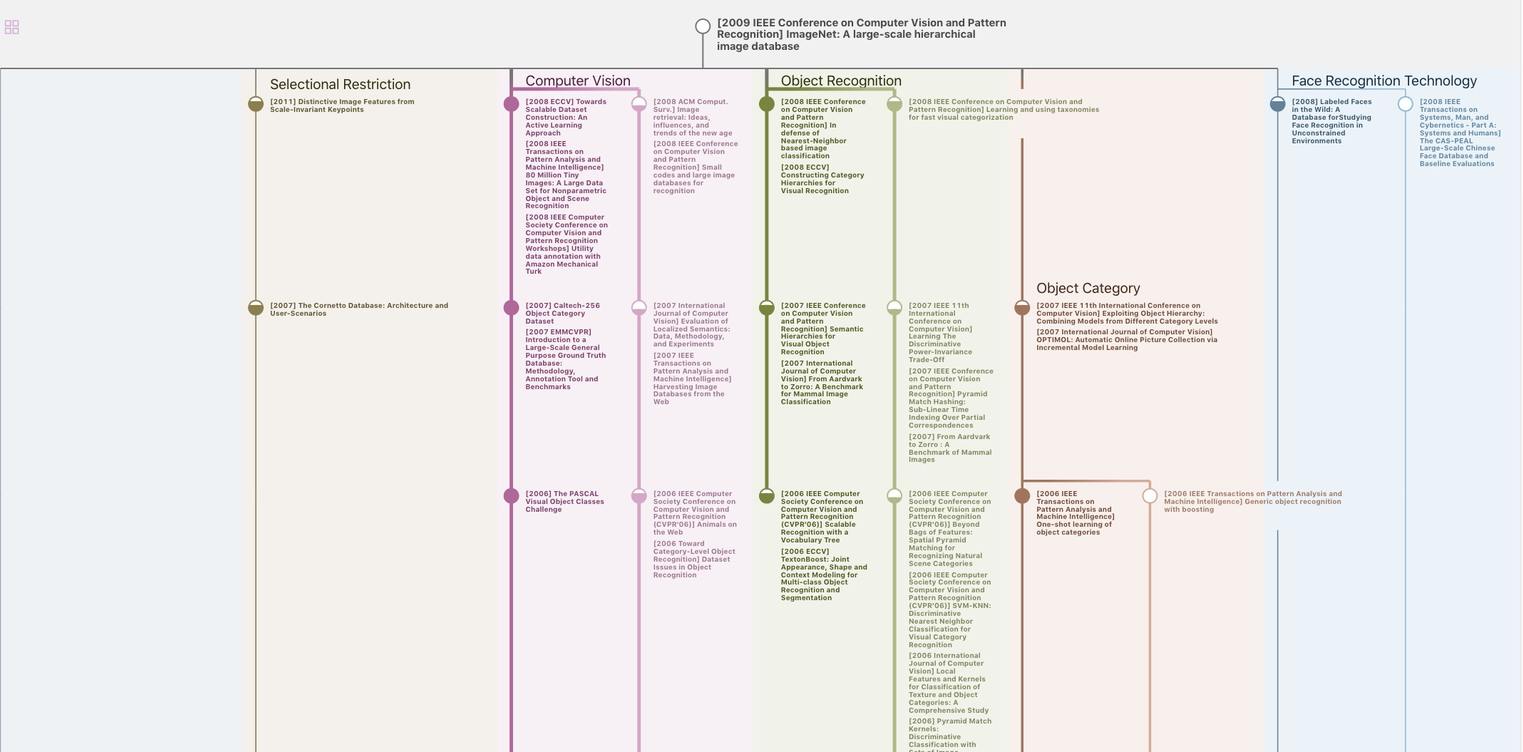
生成溯源树,研究论文发展脉络
Chat Paper
正在生成论文摘要