A systemic approach for hybrid energy management strategy based on a deep neural network
2023 IEEE VEHICLE POWER AND PROPULSION CONFERENCE, VPPC(2023)
摘要
This article presents the development of a parallel hybrid Energy Management Strategy (EMS) based on a Deep Neural Network (DNN) for fuel consumption minimization. The results show that training a NN for energy management using ARTEMIS cycle optimal paths calculated with Dynamic Programming (DP) allows it to predict with high accuracy the optimal control for the lowest fuel consumption path in various normalized cycles (NEDC, WLTC etc.). Indeed, the NN based EMS was battle-tested progressively on quasi-static vehicle model using WLTC cycle as an input. The fuel consumption during a WLTC Cycle using the NN implemented in a Backward Model Algorithm is 1.5 percent higher than the DP optimal path. Future work on the study will include dynamic MIL and HIL testing to further validate the DNN on a real-time use case.
更多查看译文
关键词
Deep Neural Networks,Deep Learning,Energy Management,Parallel Hybrid,Online Control,Fuel Consumption,Dynamic Programming
AI 理解论文
溯源树
样例
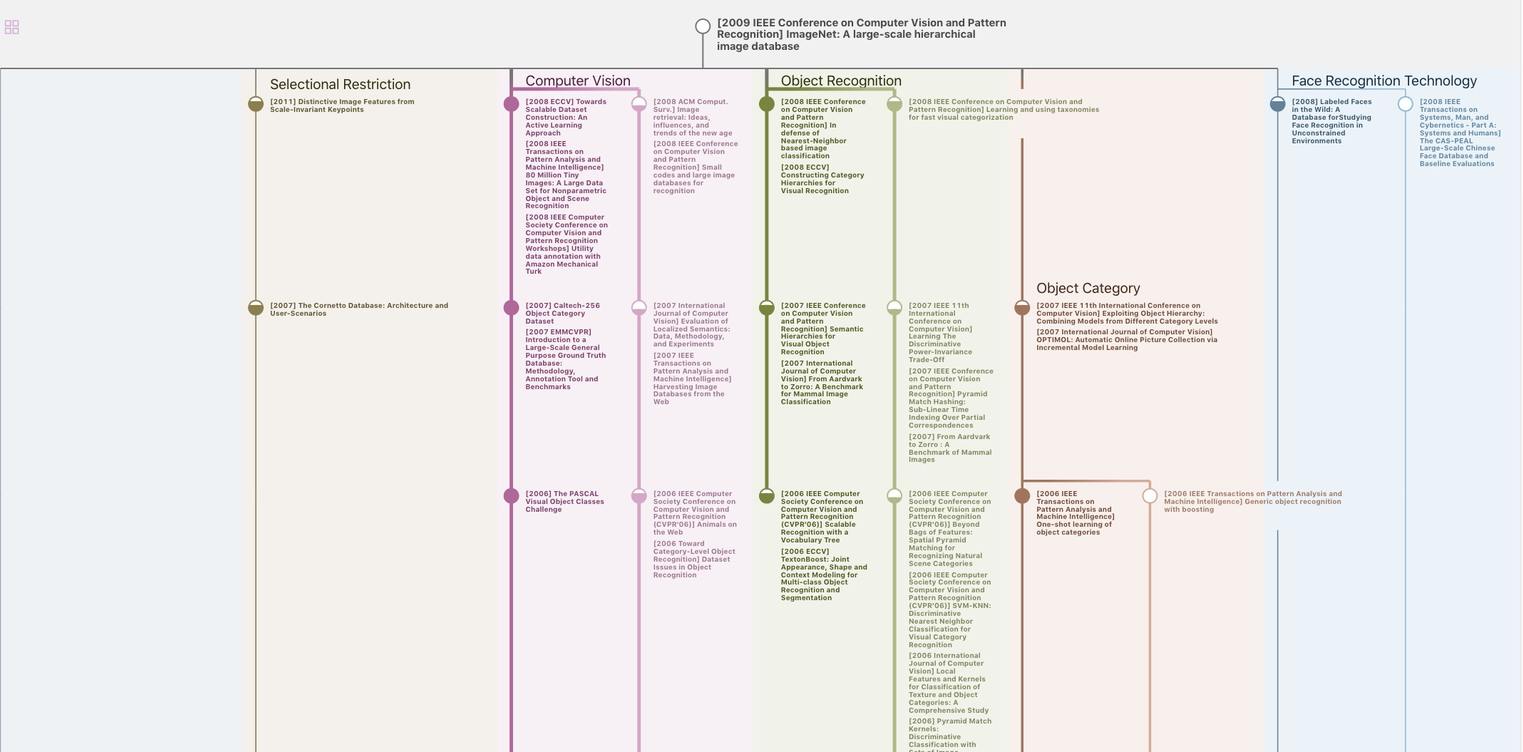
生成溯源树,研究论文发展脉络
Chat Paper
正在生成论文摘要