scCAN: Clustering With Adaptive Neighbor-Based Imputation Method for Single-Cell RNA-Seq Data
IEEE-ACM TRANSACTIONS ON COMPUTATIONAL BIOLOGY AND BIOINFORMATICS(2024)
摘要
Single-cell RNA sequencing (scRNA-seq) is widely used to study cellular heterogeneity in different samples. However, due to technical deficiencies, dropout events often result in zero gene expression values in the gene expression matrix. In this paper, we propose a new imputation method called scCAN, based on adaptive neighborhood clustering, to estimate the zero value of dropouts. Our method continuously updates cell-cell similarity information by simultaneously learning similarity relationships, clustering structures, and imposing new rank constraints on the Laplacian matrix of the similarity matrix, improving the imputation of dropout zero values. To evaluate the performance of this method, we used four simulated and eight real scRNA-seq data for downstream analyses, including cell clustering, recovered gene expression, and reconstructed cell trajectories. Our method improves the performance of the downstream analysis and is better than other imputation methods.
更多查看译文
关键词
Sequential analysis,Matrix decomposition,RNA,Gene expression,Laplace equations,DNA,Sociology,Adaptive neighborhood clustering,imputation,laplace matrix,single -cell RNA sequencing
AI 理解论文
溯源树
样例
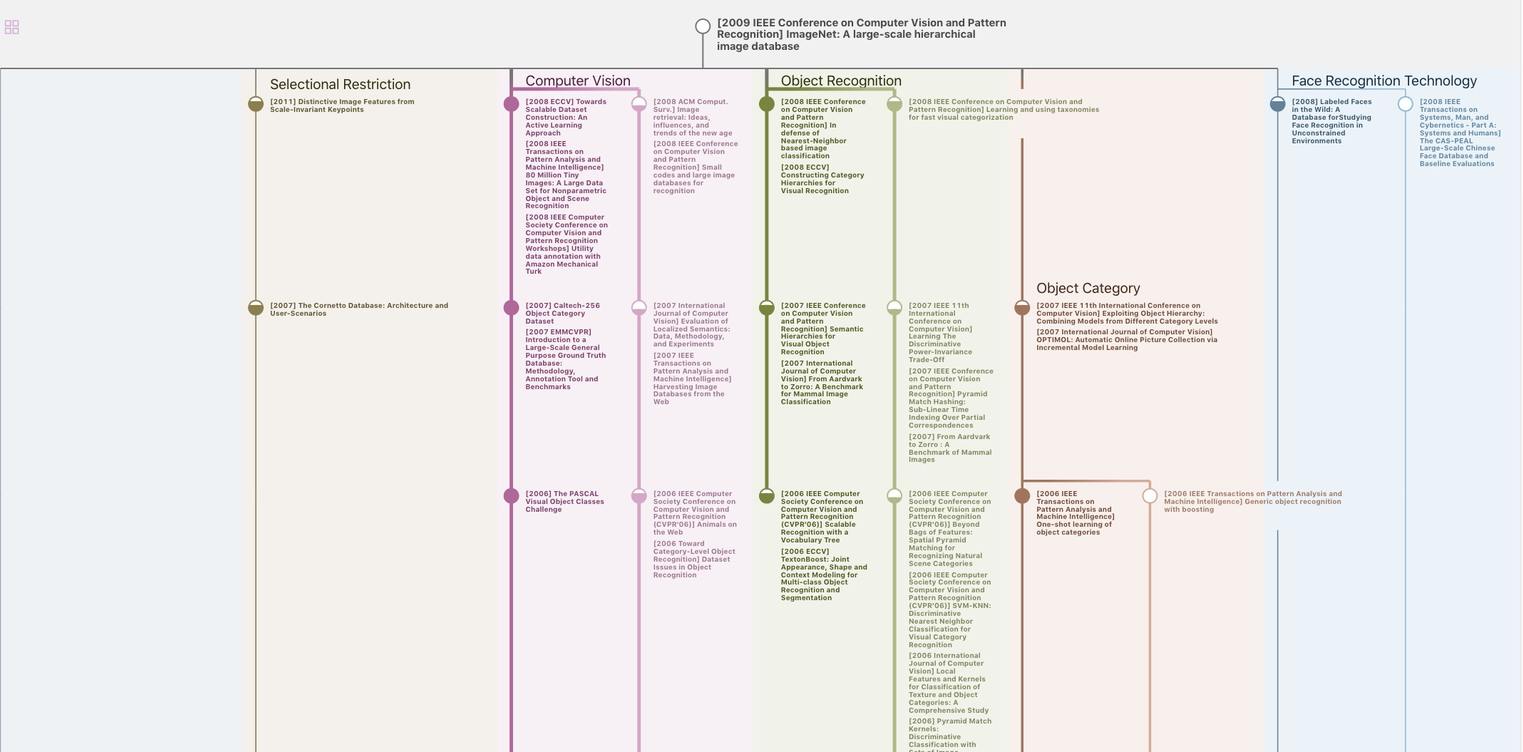
生成溯源树,研究论文发展脉络
Chat Paper
正在生成论文摘要