Speech Separation for Multi-speaker Scenarios Based on Tensor Factorization Initialization.
International Conference on Signal Processing, Communications and Computing(2023)
摘要
Speech separation is considered a complicated problem because the observed signals are made up of latent components and their delayed counterparts. However, speech separation is regarded as a critical pre-processing step for speech recognition. There are two flaws with the previous separation approaches. First, most of them are sensitive to initial values due to the large number of parameters involved. Second, speech separation in the frequency domain can result in permutation ambiguity. In this paper, an efficient system that combines tensor decomposition with Independent Low-rank Matrix Analysis (ILRMA) under the premise that the spatial covariance matrix may be jointly diagonalized is proposed. The higher-order characteristics of tensors can extract the spatial features of the observed signals and provide excellent initial values for the system. Furthermore, ILRMA can effectively avoid the permutation problem. Experimental findings express that the proposed algorithm improves separation accuracy while also achieving quicker convergence.
更多查看译文
关键词
speech separation,tensor decomposition,Low-rank matrix analysis,overlapped speech recognition,feature extraction
AI 理解论文
溯源树
样例
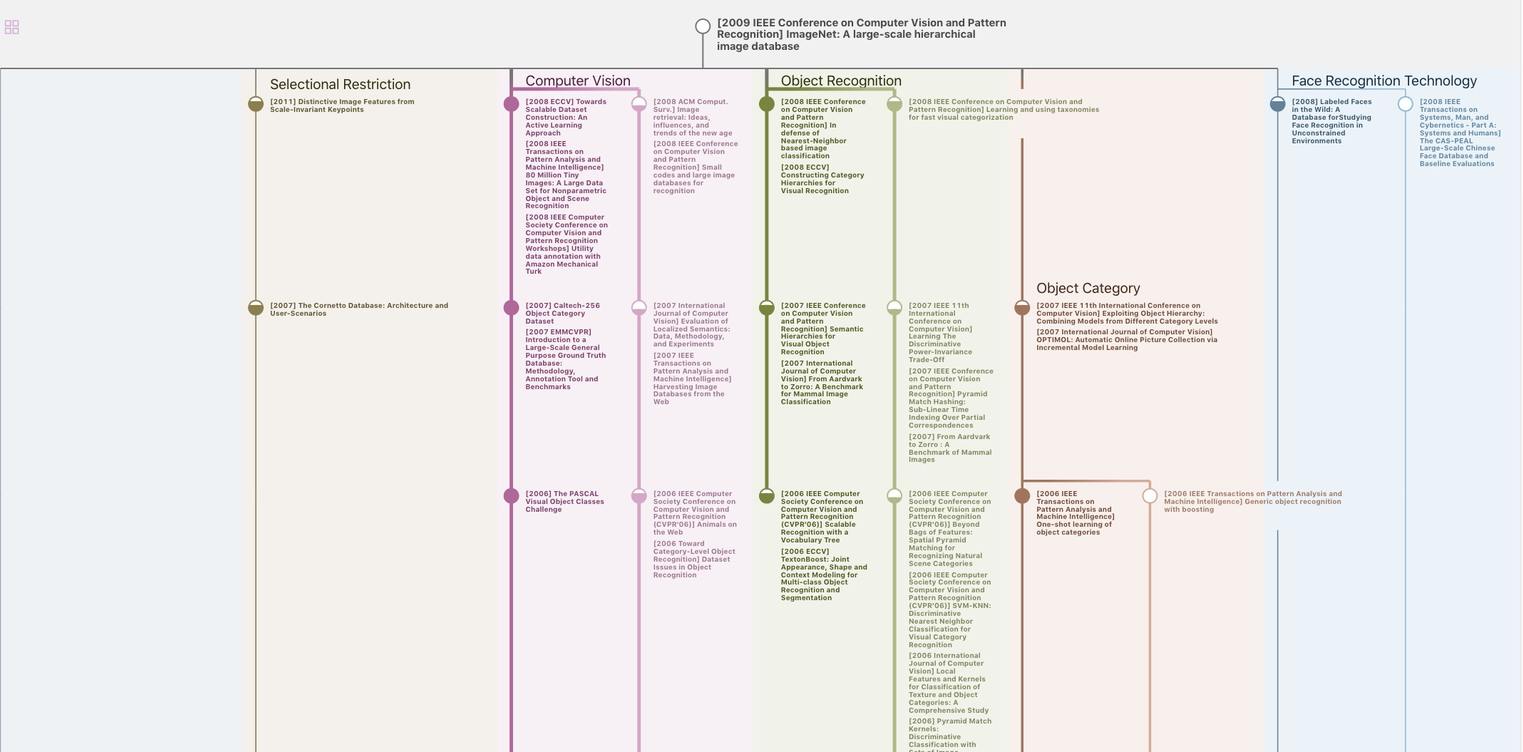
生成溯源树,研究论文发展脉络
Chat Paper
正在生成论文摘要