Multioutput Surrogate Assisted Evolutionary Algorithm for Expensive Multi-Modal Optimization Problems.
2023 IEEE International Conference on Systems, Man, and Cybernetics (SMC)(2023)
摘要
Real-world optimization problems are often computationally expensive and feature multi-modal objective functions. Surrogate-assisted evolutionary optimization has proven to be an effective approach for addressing expensive black-box optimization challenges, but the technique has not been adequately studied in multi-modal situations. In this paper, we propose a simple but effective multi-output surrogate-based approach for empowering surrogate-assisted evolutionary optimization to address expensive multi-modal optimization problems. Specifically, our proposed approach employs a multi-output Gaussian process to capture correlations between data collected from different local areas. Experiments on synthetic benchmark test problems demonstrate the effectiveness of our proposed algorithm against five state-of-the-art peer algorithms.
更多查看译文
关键词
Optimization Problem,Multimodal Problems,Expensive Problems,Expensive Optimization,Multimodal Optimization Problems,Expensive Optimization Problems,Objective Function,Local Area,Gaussian Process,Black-box Optimization,Synthetic Benchmark,Landscape,Comparable Results,Performance Measures,Alternative Models,Optimization Algorithm,Differential Evolution,Global Model,Search Space,Global Optimization,Gaussian Mixture Model,Bayesian Optimization,Radial Basis Function,Local Optimum,Polynomial Regression,Covariance Function,Multi-objective Optimization Problem
AI 理解论文
溯源树
样例
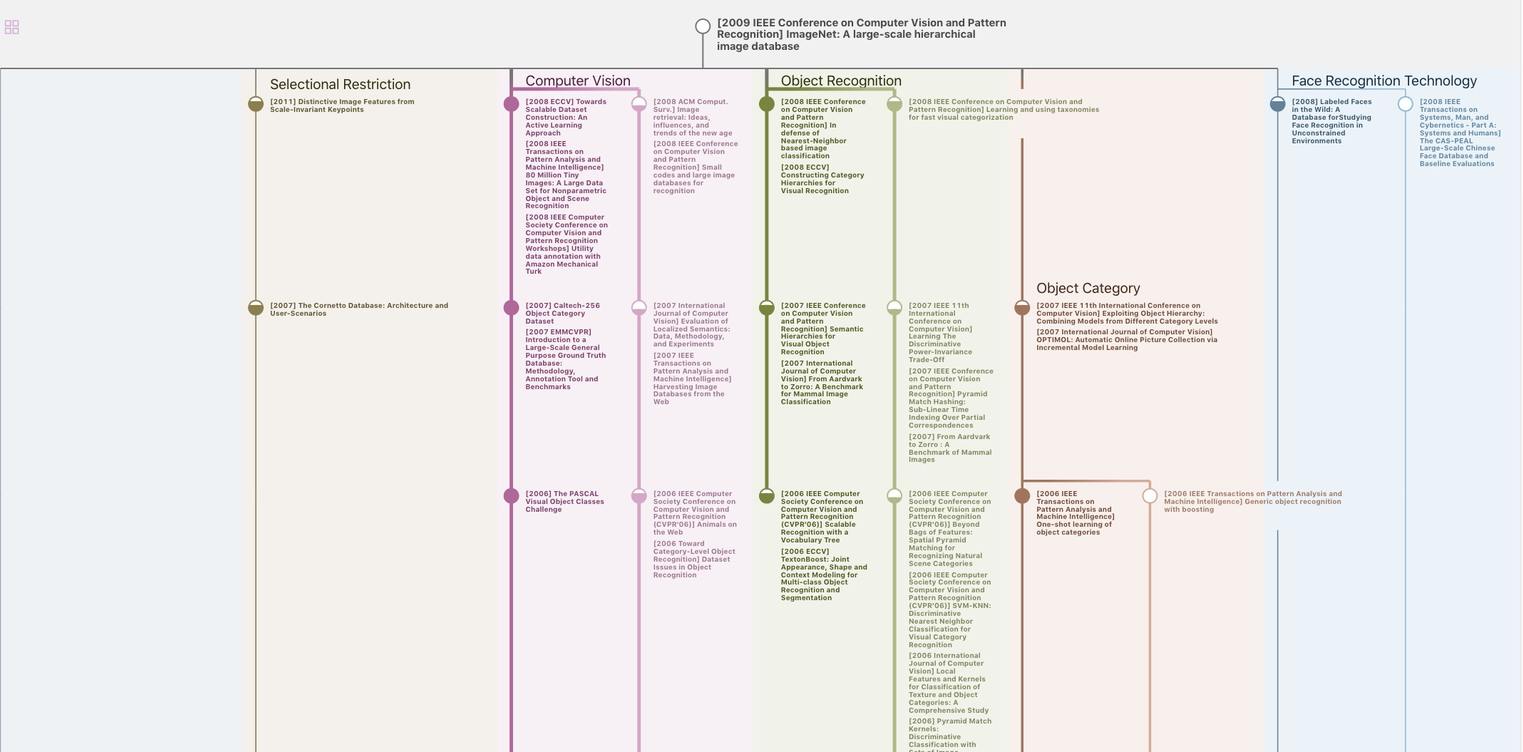
生成溯源树,研究论文发展脉络
Chat Paper
正在生成论文摘要