Temporal Feature Mining in Dynamic Graph of Brain Connectivity Data.
2023 IEEE International Conference on Systems, Man, and Cybernetics (SMC)(2023)
摘要
In recent years, the graph feature mining method of brain connection data based on graph theory has been regarded as a popular and universal technology in the field of neuroscience. How to mine valuable information from brain connection data has become a research hotspot. Current research shows that the pathogenic factors of attention deficit and hyperactivity disorder (ADHD) may be caused by the abnormal connection between brain network structures. In order to find out the pathogenic factors of ADHD patients, we also carried out frequent sub-graph mining on the connectivity graph data of brain functional network. By constantly adjusting the sup-port threshold, all the subgraphs of ADHD patients and healthy control group were mined, and the differences in brain region connectivity were successfully found out. By combining the recently introduced neural document embedding model with traditional pattern mining techniques, we regard the brain network connection structure graph as the document and frequent subgraph as the atomic unit of the embedding process. By learning the mapping, each graph can be mapped to a D-dimensional continuous vector. The mapping needs to capture the similarity between the graphs. Feature vectors can be used as the direct input of graph classification in many traditional machine learning methods. Finally, support vector machine in machine learning is used to verify the accuracy of classification, and the results show that the accuracy is high.
更多查看译文
关键词
fMRI,ADHD,brain network identification,Graph data mining,Frequent subgraph mining
AI 理解论文
溯源树
样例
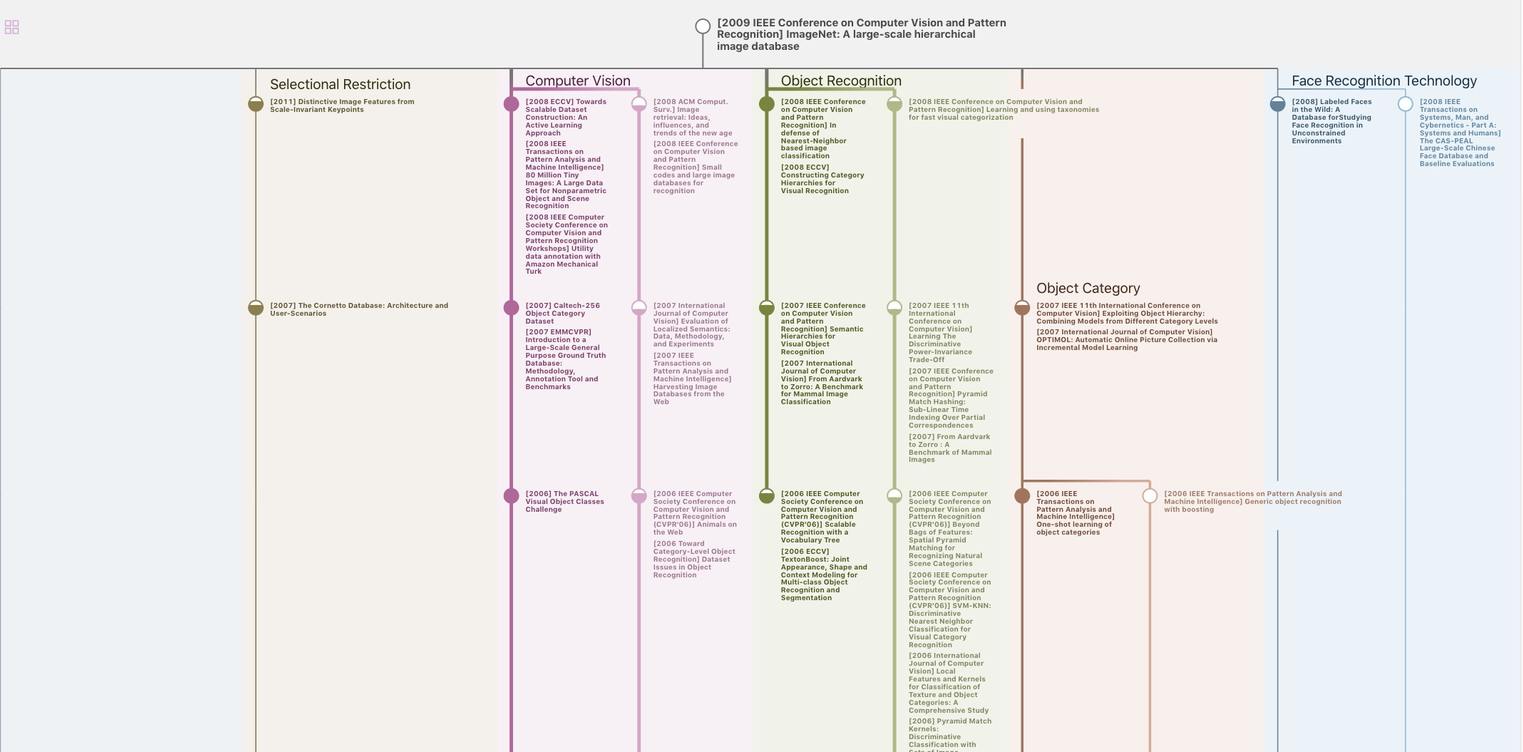
生成溯源树,研究论文发展脉络
Chat Paper
正在生成论文摘要