An Analysis of Evolutionary Migration Models for Multi-Objective, Multi-Fidelity Automl.
2023 IEEE International Conference on Systems, Man, and Cybernetics (SMC)(2023)
摘要
Methods have been proposed to maximize the efficiency of Automated Machine Learning (AutoML) systems while simplifying the search for solutions. Multi-fidelity approaches have been shown as an alternative to achieve this since they are straightforward to implement and reduce the computational cost when modeling large datasets. However, they are not suited to shifting data distributions, and they remove configurations too fast. Therefore, they must be combined with other techniques. Combining multi-fidelity methods with evolutionary algorithms and island models helps to adapt better and can contribute to maintaining diversity. This paper presents a comparative analysis of 10 network topologies distributed over a generalized island model for AutoML. A dynamic migration model with multi-objective and multi-fidelity evaluation is proposed to reduce the complexity of the tasks. This proposal is compared against state-of-the-art AutoML frameworks. It was found that Hypercube, Grid 2-dim, and Grid 3-dim topologies have the best performance as they maintain a balance in the number of connections. Furthermore, these topologies were shown to be competitive against other frameworks in the state of the art.
更多查看译文
关键词
Genetic programming,Pareto optimisation,Machine learning,Parameter tuning and algorithm configuration,Parallelization
AI 理解论文
溯源树
样例
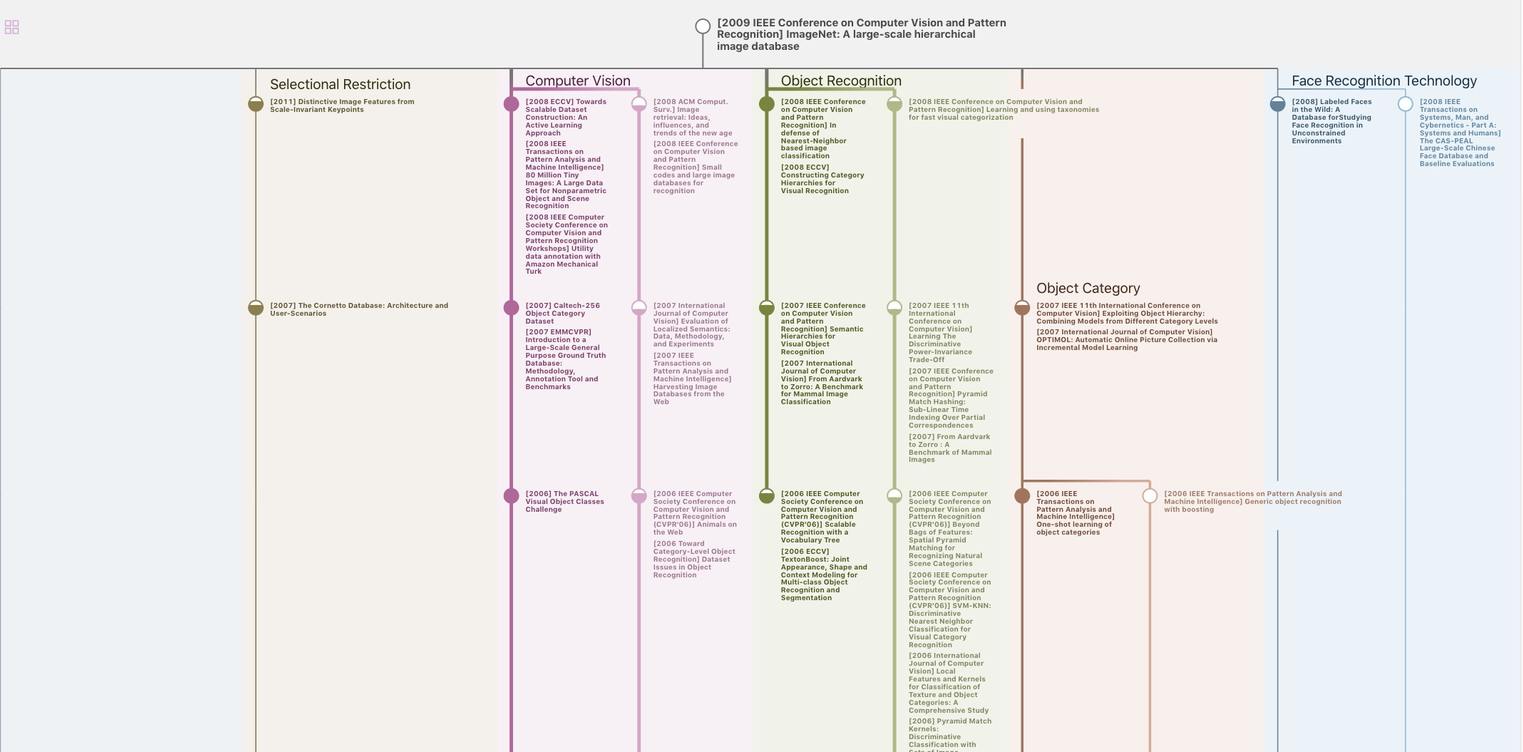
生成溯源树,研究论文发展脉络
Chat Paper
正在生成论文摘要