Proximal Policy Optimization-based Reinforcement Learning for End-to-end Autonomous Driving
2023 38th Youth Academic Annual Conference of Chinese Association of Automation (YAC)(2023)
摘要
This paper proposes an end-to-end method based on deep reinforcement learning (DRL) for autonomous driving (AD) in diverse road conditions. The proposed method is developed based on the proximal policy optimization (PPO). The solution is composed of an encoder network for image processing and a policy network for autonomous driving. By converting the image into a multi-dimensional vector through the encoder network, the time for autonomous driving vehicles (ADVs) to process the environmental information is reduced. Moreover, The attention mechanism-based squeeze-and-excitation networks (SENet) block is added to the encoder network to improve the accuracy of extracting the road information from raw images, thus greatly accelerating the convergence. Next, a specific reward function is designed for the simulation. The reward function is about the deviation from the center lane and the yaw angle for autonomous driving vehicles. The actor-critic structure optimizes network parameters to perform policy improvements and obtain the optimal policy based on accumulated experience. The proposed method has been verified in the CARLA simulation environment. The results demonstrate that the driving stability of autonomous driving vehicles get improvements.
更多查看译文
关键词
Autonomous Driving,Deep Reinforcement Learning,Attention Mechanism
AI 理解论文
溯源树
样例
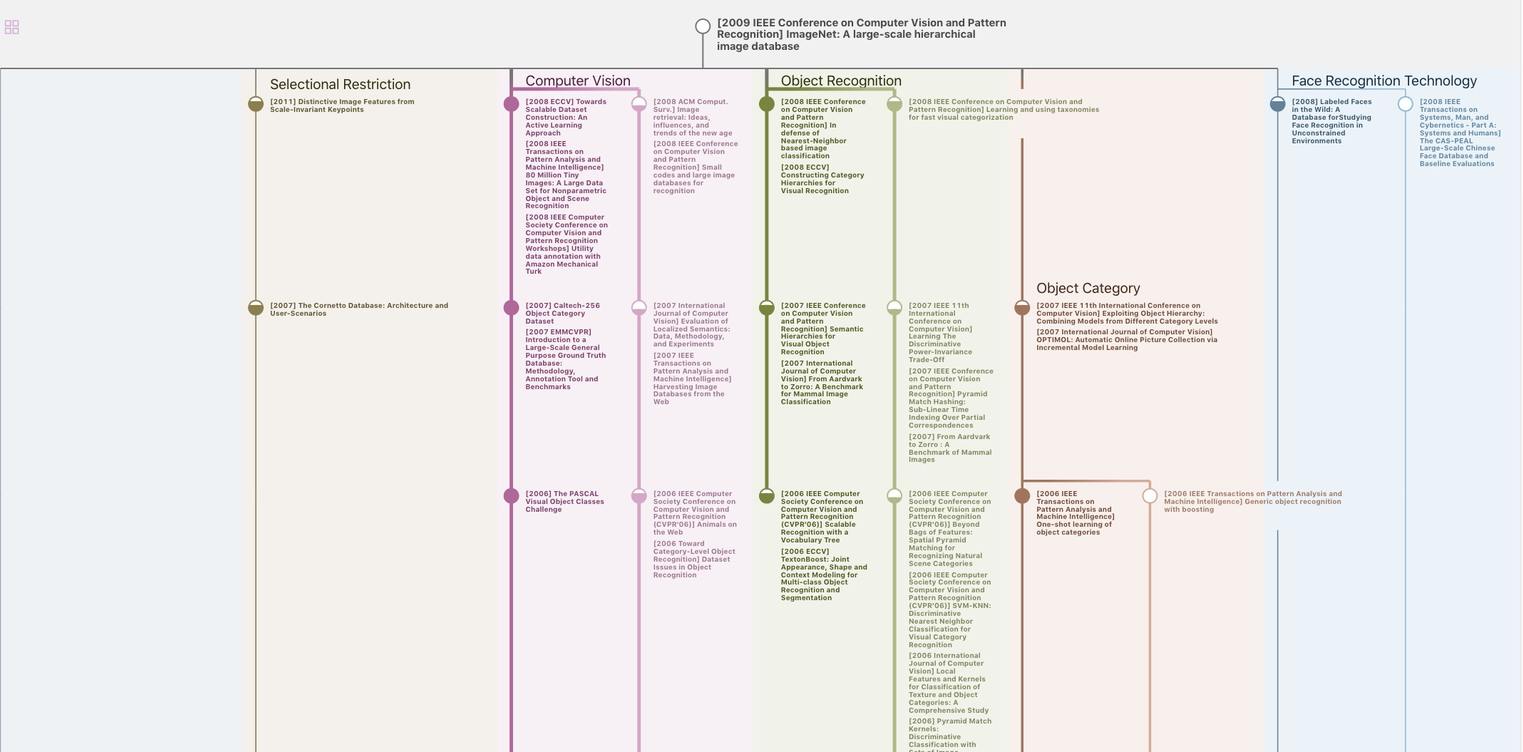
生成溯源树,研究论文发展脉络
Chat Paper
正在生成论文摘要