Prediction of Electrical Characteristics of a-IGZO TFT Based on Transfer Learning-Based Variational Autoencoder.
2023 IEEE International Conference on Systems, Man, and Cybernetics (SMC)(2023)
摘要
In this study, we proposed a transfer-learning based variational autoencoder model for predicting the electrical characteristics in the parameter tuning process of a-IGZO TFT structure design. The result achieve a high R2 score of 0.9704 with a low-computing-power hardware-friendly method that reduced time consumption significantly compared to prior approaches. The findings have practical implications for mitigating the time-consuming nature of TCAD simulations, and the method can expand to various types of input data while ensuring high performance and generalization. We demonstrated significant improvement in generalization and accuracy through a k-fold validation.
更多查看译文
关键词
Electrical Characteristics,Variational Autoencoder,Characteristics Of Thin-film Transistors,a-IGZO Thin-film Transistors,Tuning Parameter,Transfer Learning,General Improvement,Various Types Of Data,K-fold Validation,Autoencoder Model,Types Of Input Data,R2 Score,Simulated Nature,Variational Autoencoder Model,Training Set,Learning Rate,Active Region,Latent Variables,Input Variables,Transfer Curves,Polynomial Regression,Saturation Region,Subthreshold Swing,Manual Feature Extraction,Transfer Learning Method,Threshold Voltage,Metal Gate,Electronic Features,Semiconductor Technology
AI 理解论文
溯源树
样例
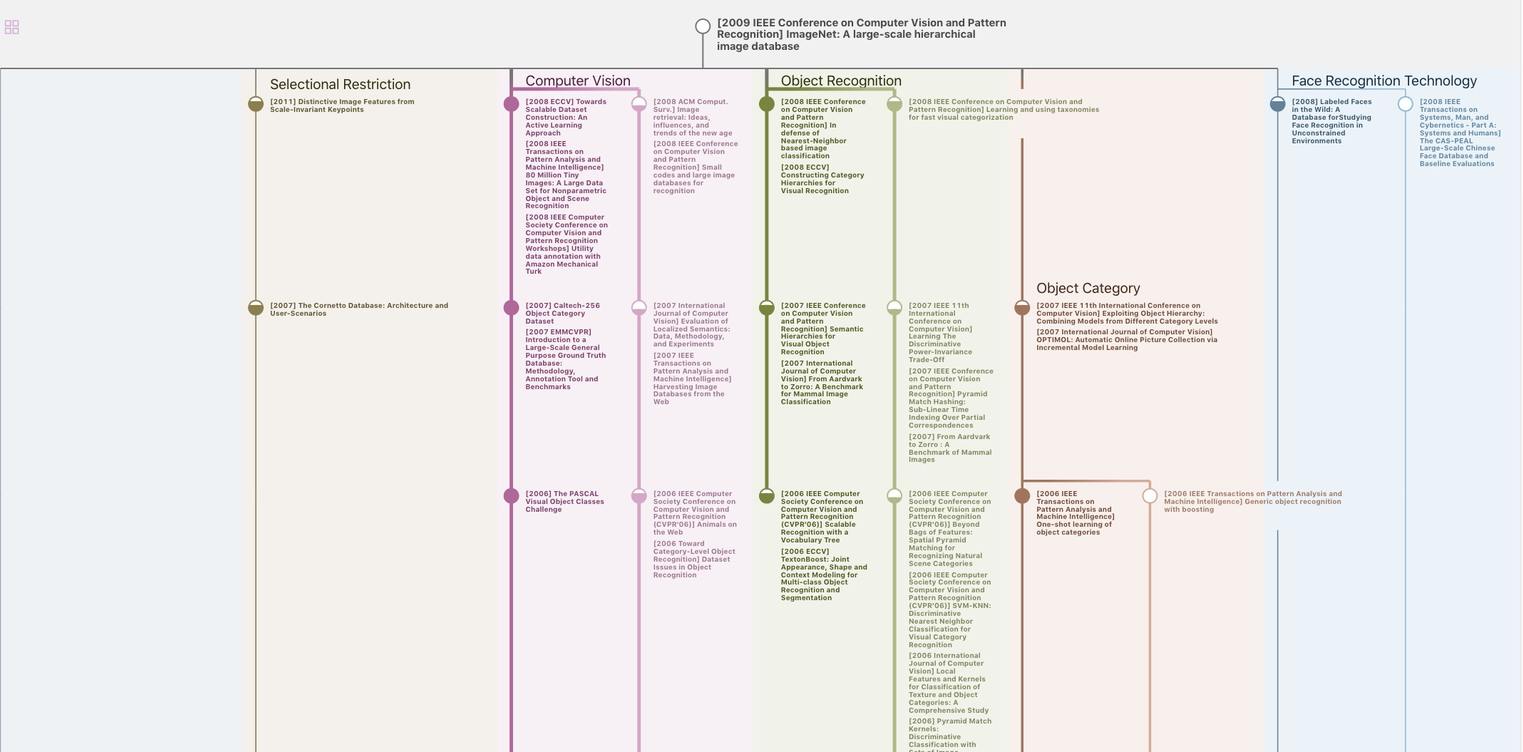
生成溯源树,研究论文发展脉络
Chat Paper
正在生成论文摘要