How to Find a Large Solution Set to Cover the Entire Pareto Front in Evolutionary Multi-Objective Optimization.
2023 IEEE International Conference on Systems, Man, and Cybernetics (SMC)(2023)
摘要
Recently, it has been pointed out in many studies that the performance of evolutionary multi-objective optimization (EMO) algorithms can be improved by selecting solutions from all examined solutions stored in an unbounded external archive. This is because in general the final population is not the best subset of the examined solutions. To obtain a good final solution set in such a solution selection framework, subset selection from a large candidate set (i.e., all examined solutions) has been studied. However, since good subsets cannot be obtained from poor candidate sets, a more important issue is how to find a good candidate set, which is the focus of this paper. In this paper, we first visually demonstrate that the entire Pareto front is not covered by the examined solutions through computational experiments using MOEA/D, NSGA-III and SMS-EMOA on DTLZ test problems. That is, the examined solution set stored in the unbounded archive has some large holes (i.e., some uncovered area of the Pareto front). Next, to evaluate the quality of the examined solution set (i.e., to measure the size of the largest hole), we propose the use of a variant of the inverted generational distance (IGD) indicator. Then, we propose a simple modification of EMO algorithms to improve the quality of the examined solution set. Finally, we demonstrate the effectiveness of the proposed modification through computational experiments.
更多查看译文
关键词
Evolutionary multi-objective optimization,Pareto front,solution selection framework,unbounded external archive
AI 理解论文
溯源树
样例
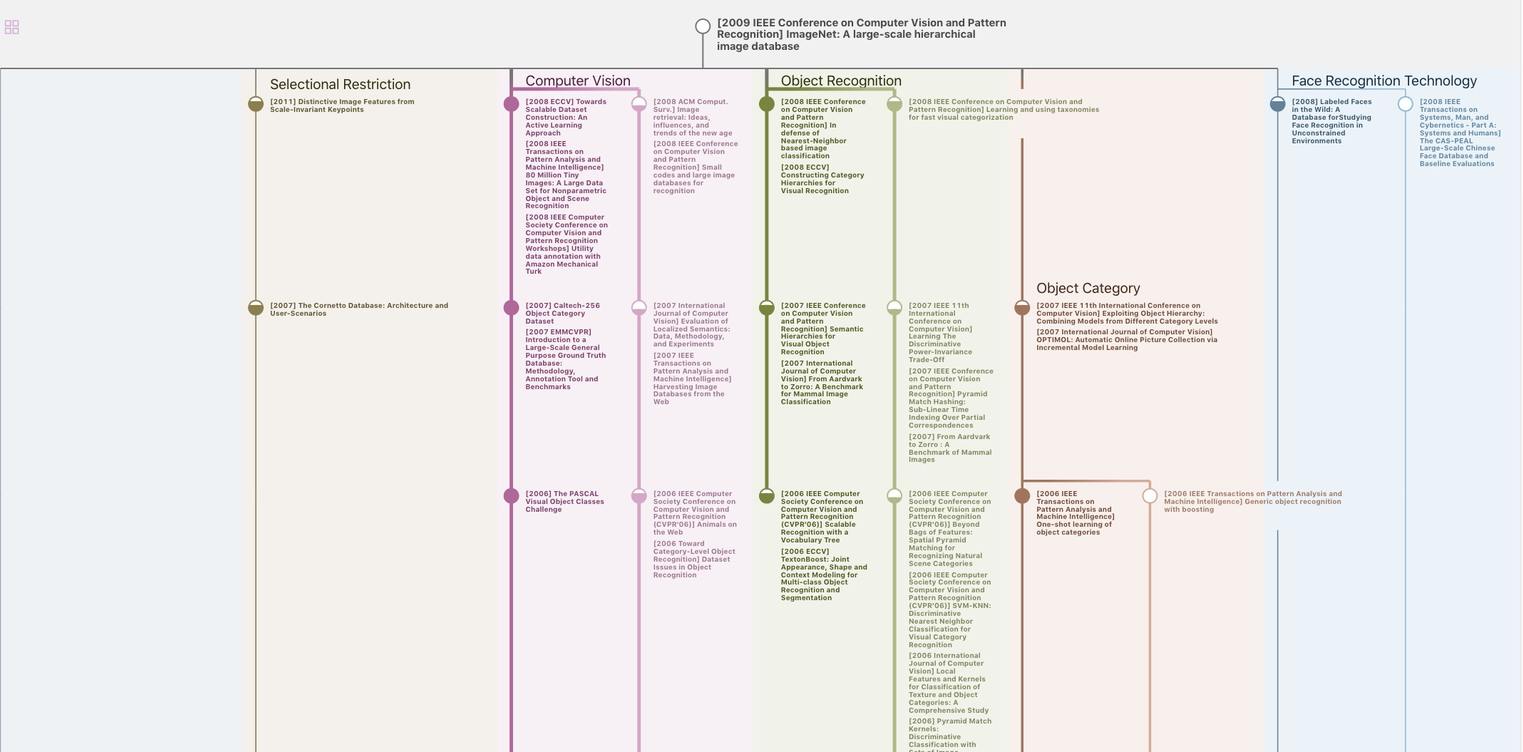
生成溯源树,研究论文发展脉络
Chat Paper
正在生成论文摘要