Density-aware Swin Transformer for Compressed Point Cloud Geometry Artifacts Removal.
2023 IEEE International Conference on Visual Communications and Image Processing (VCIP)(2023)
摘要
Geometry-based point cloud compression (G-PCC), as a prevalent compression technique, has achieved remarkable compression efficiency, thereby significantly reducing the cost of transmission and storage. However, the compressed point clouds inevitably suffer from severe compression artifacts, i.e., geometry distortion, when the compression ratio increases. To address this, we propose the DensityFormer, the first transformer-based network to restore the geometry distortion in the compressed point cloud. Particularly, our approach focuses on two prominent challenges for this: i) the discrete points demand more stringent requirements for long-range contextual information modeling and ii) the point cloud exhibits a non-uniformed point distribution. For the first challenge, our DensityFormer introduce the Swin Transformer-based hierarchical encoder-decoder architecture, intending to model the multi-grained global contextual information for geometric restoration, based on the superior long-range dependency modeling capability of 3D Swin Transformer block. To solve the second challenge, we propose the density-aware Swin Transformer block on the basis of the intuition that the local density of the point cloud can identify the distribution of points, thereby enabling the adaptive non-uniformed restoration for compressed point clouds. By incorporating the above two advanced techniques, our DensityFormer has shown superior restoration capability on multiple typical benchmark datasets, which outperforms existing state-of-the-art (SOTA) methods by an average of 0.55 dB.
更多查看译文
关键词
Geometry-based point cloud compression,point cloud compression geometry restoration
AI 理解论文
溯源树
样例
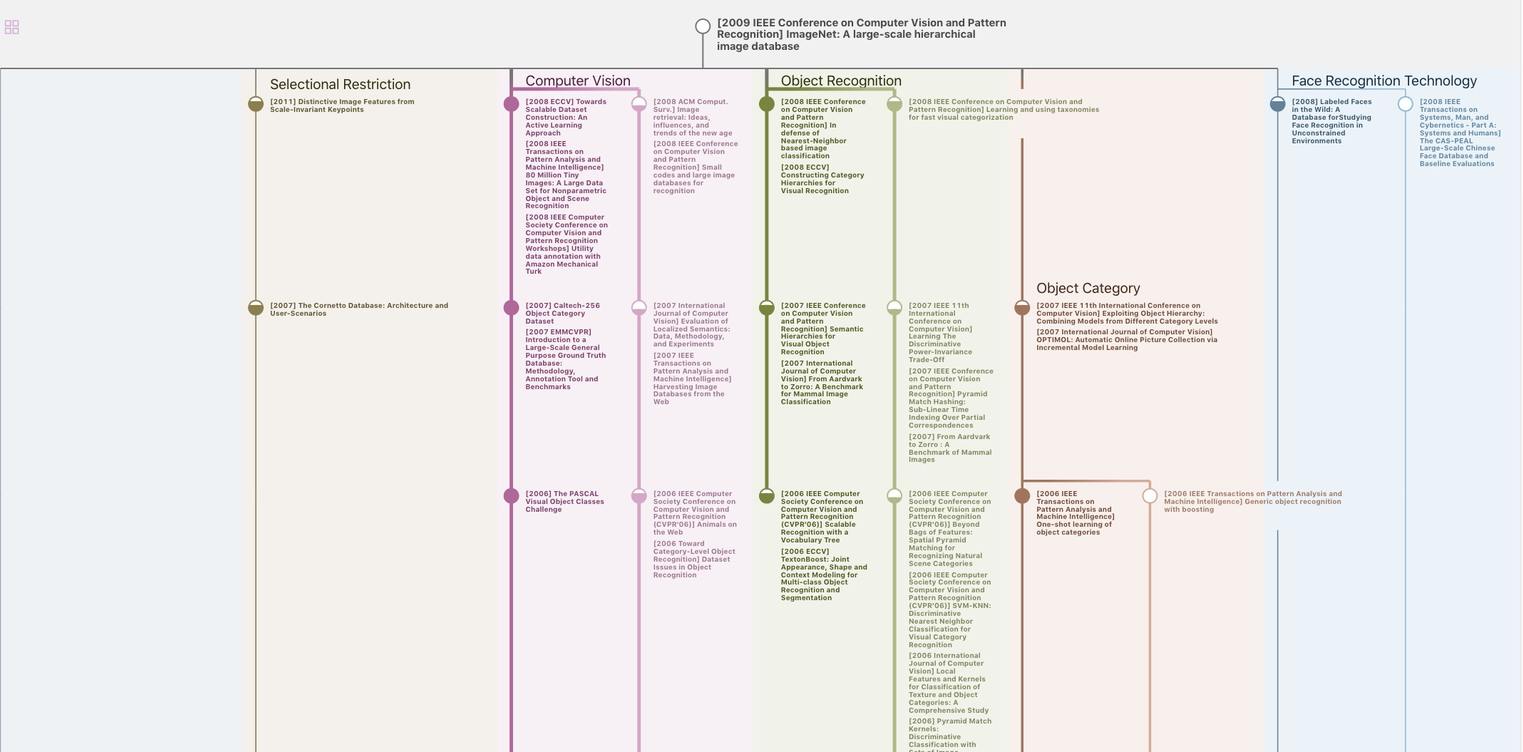
生成溯源树,研究论文发展脉络
Chat Paper
正在生成论文摘要