Hybrid Implicit Neural Image Compression with Subpixel Context Model and Iterative Pruner.
2023 IEEE International Conference on Visual Communications and Image Processing (VCIP)(2023)
摘要
Recently, image compression based on Implicit Neural Representation (INR) has gained interest. However, the performance of INR-based methods is poor because MLP can not fit high-frequency information well. Recently, a kind of hybrid INR method was proposed, which transmits not only the parameters of MLP but also some external latents to help to overfit at the decoder. Despite its low MACs (Multiply–Accumulate Operations per pixel), the decoding time is still slow due to the serial autoregressive context model for entropy coding. Hence, we propose two methods to improve the hybrid INR method’s decoding speed and performance. First, the Subpixel Context Model (SAM) is aimed to speed up the process of entropy coding. The proposed SAM is latent by latent instead of pixel by pixel. Second, we add an iterative pruner to the pipeline of hybrid INR compression, to further reduce the bit rate. The performance and MACs of our approach are on par with the SOTA of the hybrid INR methods. The decoding speed is about eight times faster on CPU, and three times faster on GPU.
更多查看译文
关键词
practical image compression,implicit neural representation,model compression
AI 理解论文
溯源树
样例
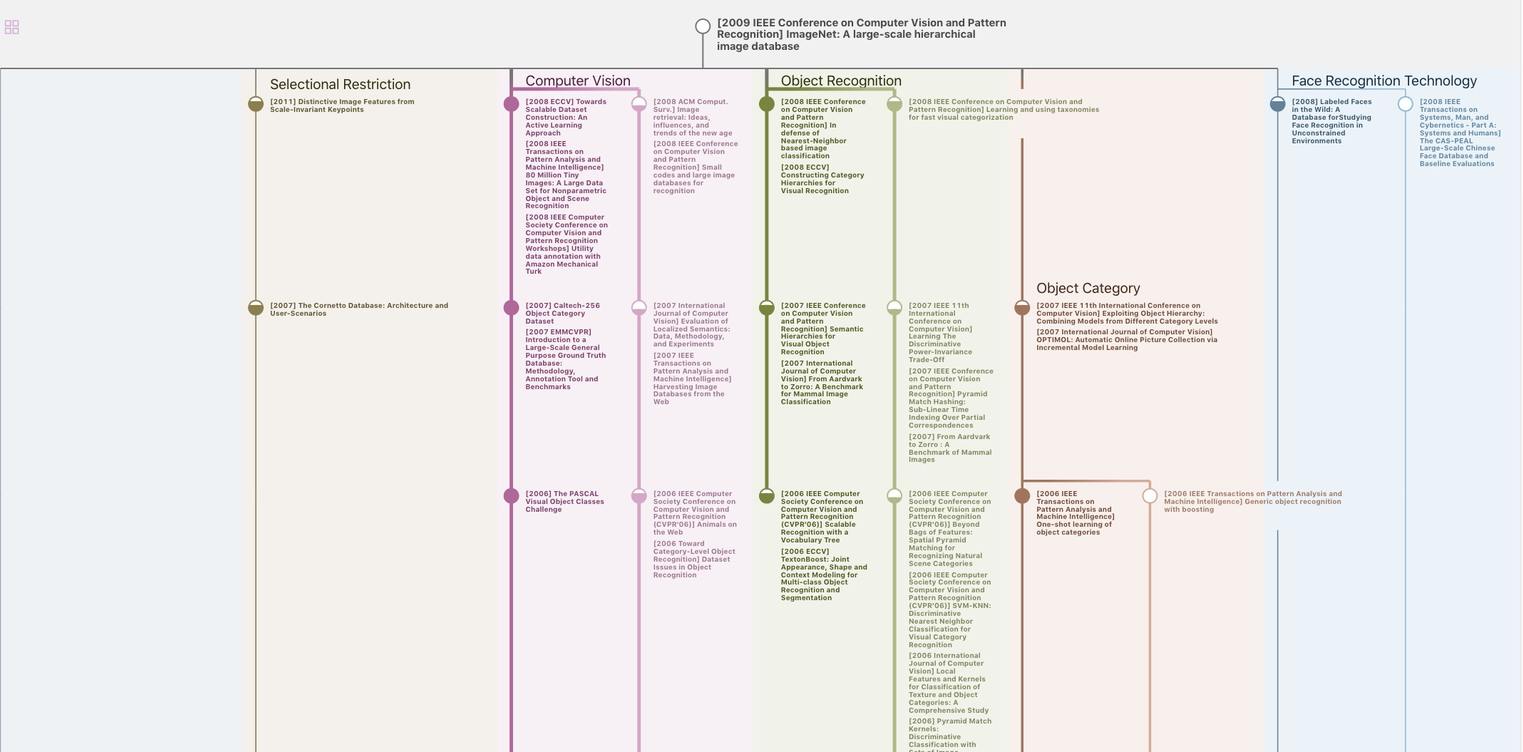
生成溯源树,研究论文发展脉络
Chat Paper
正在生成论文摘要