Eye Blink-Driven EEG: A Step Towards Improved Real-World Data Classification
2023 IEEE EMBS Special Topic Conference on Data Science and Engineering in Healthcare, Medicine and Biology(2023)
摘要
Recent developments in cognitive neuroscience have emphasized the use of naturalistic experimental paradigms, especially for real-world tasks like driving. This research introduced a blink-locked EEG segmentation method and contrasted its efficacy with traditional EEG segmentation. For three difficulty levels of proactive and reactive driving, we show a significant improvement in classification accuracy using a multi-classifier SVM with the blink-locked method, indicating enhancements of 4.3% for proactive driving and 4.4% for reactive driving. These findings underscore the potential of leveraging physiological markers, such as eye blinks, to enhance EEG data segmentation and deepen our understanding of cognitive dynamics in real-life scenarios.
更多查看译文
关键词
Difficulty Level,EEG Data,Eye Blinks,Improve Classification Accuracy,Physiological Markers,EEG Segments,Naturalistic Paradigms,Cognitive Processes,Cohen’s D,Cognitive Status,EEG Techniques
AI 理解论文
溯源树
样例
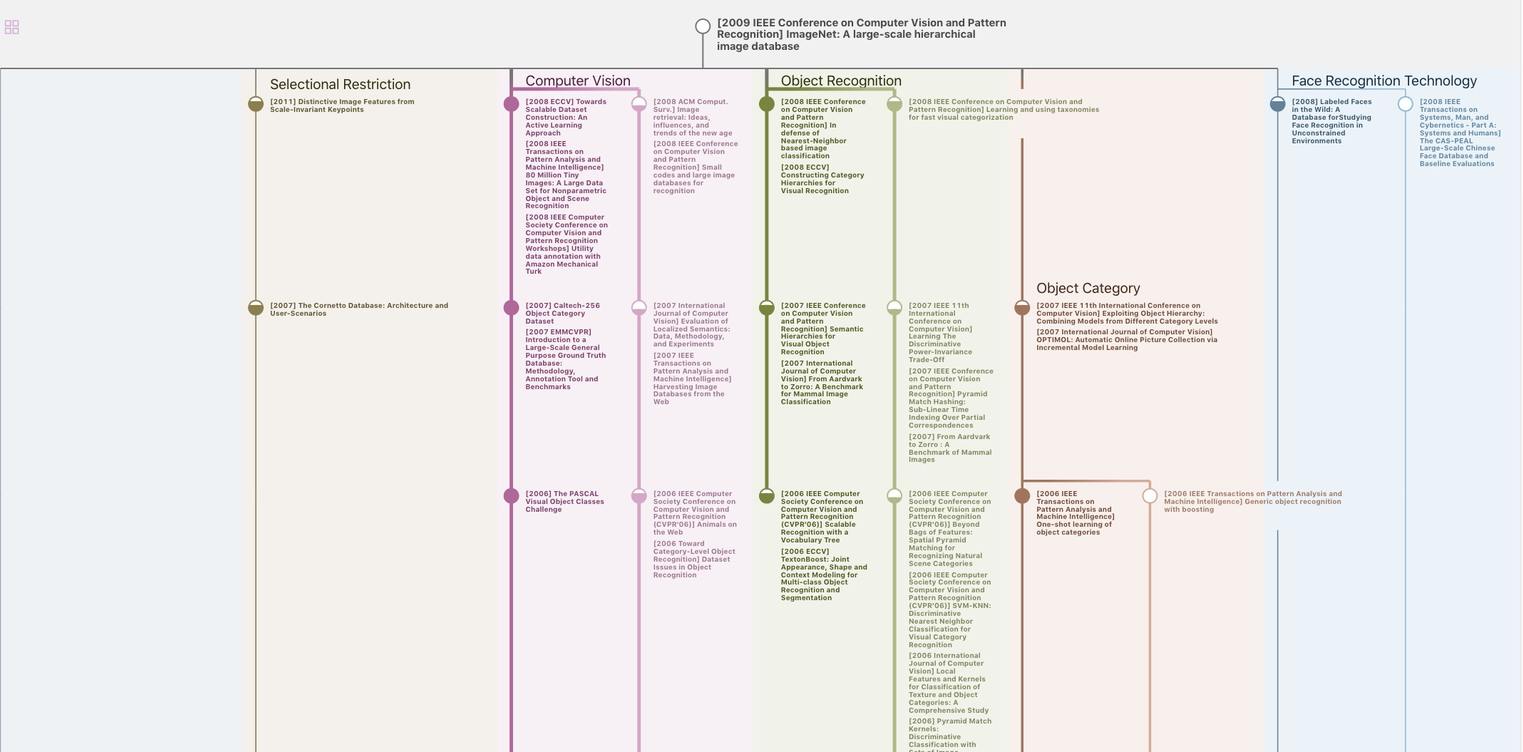
生成溯源树,研究论文发展脉络
Chat Paper
正在生成论文摘要