Cycle-Consistent Generative Adversarial Network Architectures for Audio Visual Speech Recognition.
International Conference on Signal Processing, Communications and Computing(2023)
摘要
Generative Adversarial Networks (GANs) have found extensive applications in image classification and image generation domains. Nevertheless, their utilisation for recognising and detecting multimodal images presents considerable difficulties. Audio Visual Speech Recognition (AVSR) is a classic task in multimodal audio-visual sensing, which leverages audio inputs from human speech and aligned visual inputs from lip movements. However, the performance of AVSR is impacted by the inherent discrepancies present in real-world environments, such as variations in lighting intensity, noise, and sampling devices. To mitigate these challenges, this paper proposed a AVSR architecture based on a specially constructed Cycle-Consistent Adversarial Networks (CycleGAN). First, on the visual side, we used data-augmentation methods such as flipping and rotating to process video data, increasing the number and variety of samples. This increases the robustness and generalisation capabilities of the model. Then, since the AVSR dataset was collected in different environments with different styles, we transformed the original images multiple times through the specially constructed CycleGAN module to address the inherent differences in the different environments. To validate the approaches, we used augmented data from well-known datasets (LRS2-Lip Reading Sentences 2 and LRS3) in the training process. Experimental results validate the correctness and effectiveness of the approach.
更多查看译文
关键词
Generative Adversarial Networks (GANs),deep learning,audio visual speech recognition
AI 理解论文
溯源树
样例
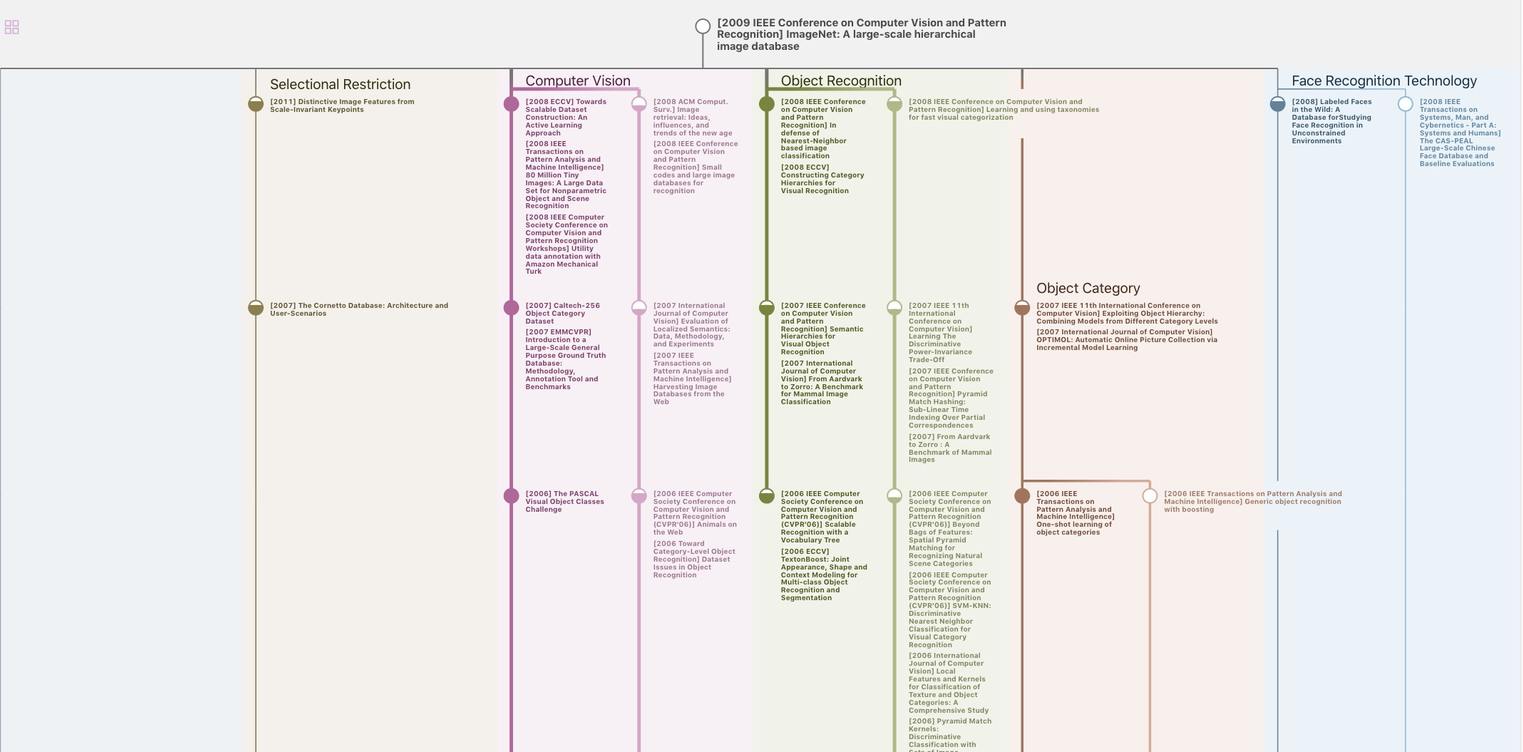
生成溯源树,研究论文发展脉络
Chat Paper
正在生成论文摘要