Surrogate-Assisted Evolutionary Optimization Based on Interpretable Convolution Network.
2023 IEEE International Conference on Systems, Man, and Cybernetics (SMC)(2023)
摘要
When performing evolutionary optimization for computationally expensive objective, surrogate-assisted evolutionary algorithm(SAEA) is an effective approach. However, due to the limited availability of data in these scenarios, it can be challenging to create a highly accurate surrogate model, leading to reduced optimization effectiveness. To address this issue, we propose an Interpretable Convolution Network(ICN) for offline surrogate-assited evolutionary optimization. ICN retains the non-linear expression ability of traditional neural networks, while possessing the advantages of clear physical structure and the ability to incorporate prior knowledge during network parameter design and training process. We compare ICN-SAEA with tri-training method(TT-DDEA) and model-ensemble method(DDEA-SA) in several benchmark problems. Experimental results show that ICN-SAEA is better in searching optimal solution than compared algorithms.
更多查看译文
关键词
Neural Network,Alternative Models,Evolutionary Algorithms,Network Training,Data Scenarios,Benchmark Problems,Convolutional Neural Network,Deep Neural Network,Convolutional Layers,Performance Of Algorithm,Hidden Layer,Feature Maps,Forms Of Knowledge,Time Complexity,Convolution Operation,Convolution Kernel,Taylor Expansion,High-dimensional Problems,Latin Hypercube Sampling,Convolution Module,Weak Knowledge,Strong Knowledge,Ensemble Strategy,Mel-frequency Cepstral Coefficients,Improve Network Performance,Function Tests,Semi-supervised Learning,Deep Learning Models,Root Mean Square Error
AI 理解论文
溯源树
样例
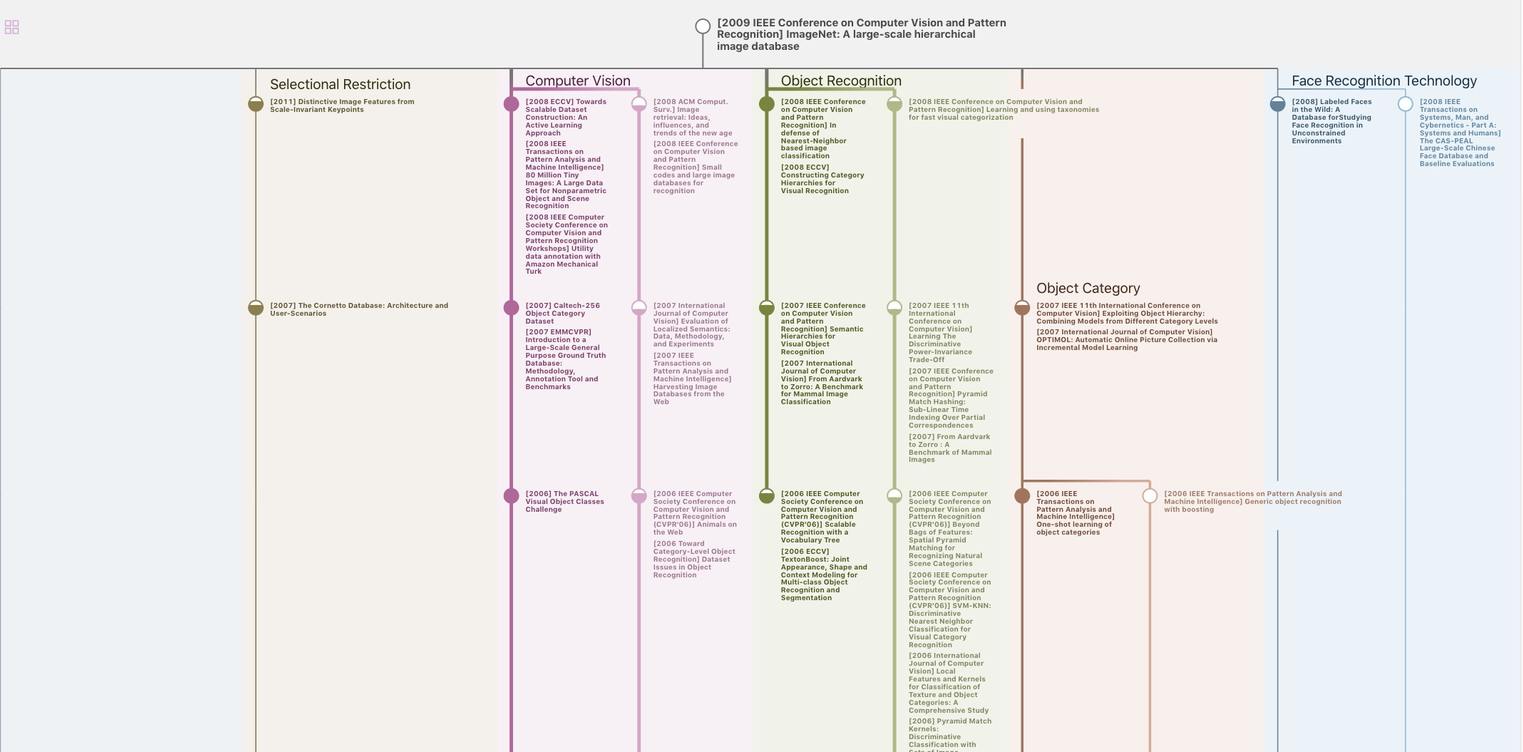
生成溯源树,研究论文发展脉络
Chat Paper
正在生成论文摘要