Spatiotemporal Graph Autoencoder Network for Skeleton-Based Human Action Recognition
crossref(2024)
摘要
Skeleton-based human action recognition is a challenging yet important technique because of its wide range of applications in many fields, including patient monitoring, security surveillance, and observing human-machine interactions. Many algorithms that attempt to distinguish between many types of activities have been proposed. However, most practical applications require highly accurate detection of specific types of activities. In this study, a novel and highly accurate spatiotemporal graph autoencoder network for skeleton-based human action recognition is proposed. Furthermore, an extensive study was conducted using different modalities. For this purpose, a spatiotemporal graph autoencoder that automatically learns spatial as well as temporal patterns from human skeleton datasets was built. The powerful graph convolutional network named GA-GCN, developed in this study, notably outperforms most of the existing state-of-the-art methods based on two common datasets, namely NTU RGB+D and NTU RGB+D 120. On the first dataset, we achieved an accuracy of 92.3% and 96.7% based on the cross-subject and cross-view evaluations, respectively. On the other more challenging dataset (i.e. NTU RGB+D 120), GA-GCN achieved 88.8% and 90.4% based on the cross-subject and cross-set evaluation, respectively.
更多查看译文
AI 理解论文
溯源树
样例
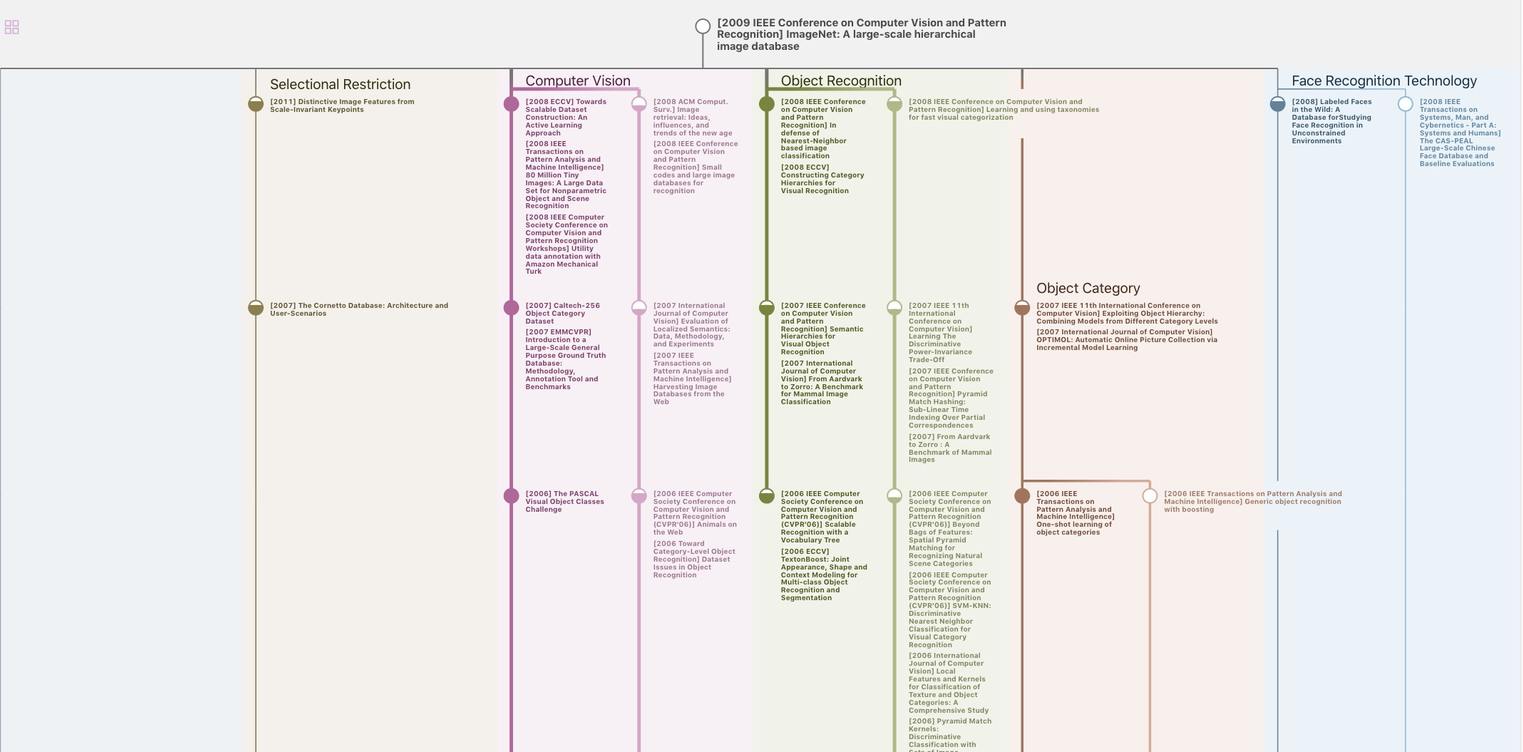
生成溯源树,研究论文发展脉络
Chat Paper
正在生成论文摘要