Machine Learning (In) Security: A Stream of ProblemsJust Accepted
Digital Threats: Research and Practice(2022)
摘要
Machine Learning (ML) has been widely applied to cybersecurity and is considered state-of-the-art for solving many of the open issues in that field. However, it is very difficult to evaluate how good the produced solutions are, since the challenges faced in security may not appear in other areas. One of these challenges is the concept drift, which increases the existing arms race between attackers and defenders: malicious actors can always create novel threats to overcome the defense solutions, which may not consider them in some approaches. Due to this, it is essential to know how to properly build and evaluate an ML-based security solution. In this paper, we identify, detail, and discuss the main challenges in the correct application of ML techniques to cybersecurity data. We evaluate how concept drift, evolution, delayed labels, and adversarial ML impact the existing solutions. Moreover, we address how issues related to data collection affect the quality of the results presented in the security literature, showing that new strategies are needed to improve current solutions. Finally, we present how existing solutions may fail under certain circumstances, and propose mitigations to them, presenting a novel checklist to help the development of future ML solutions for cybersecurity.
更多查看译文
关键词
machine learning,cybersecurity,data streams
AI 理解论文
溯源树
样例
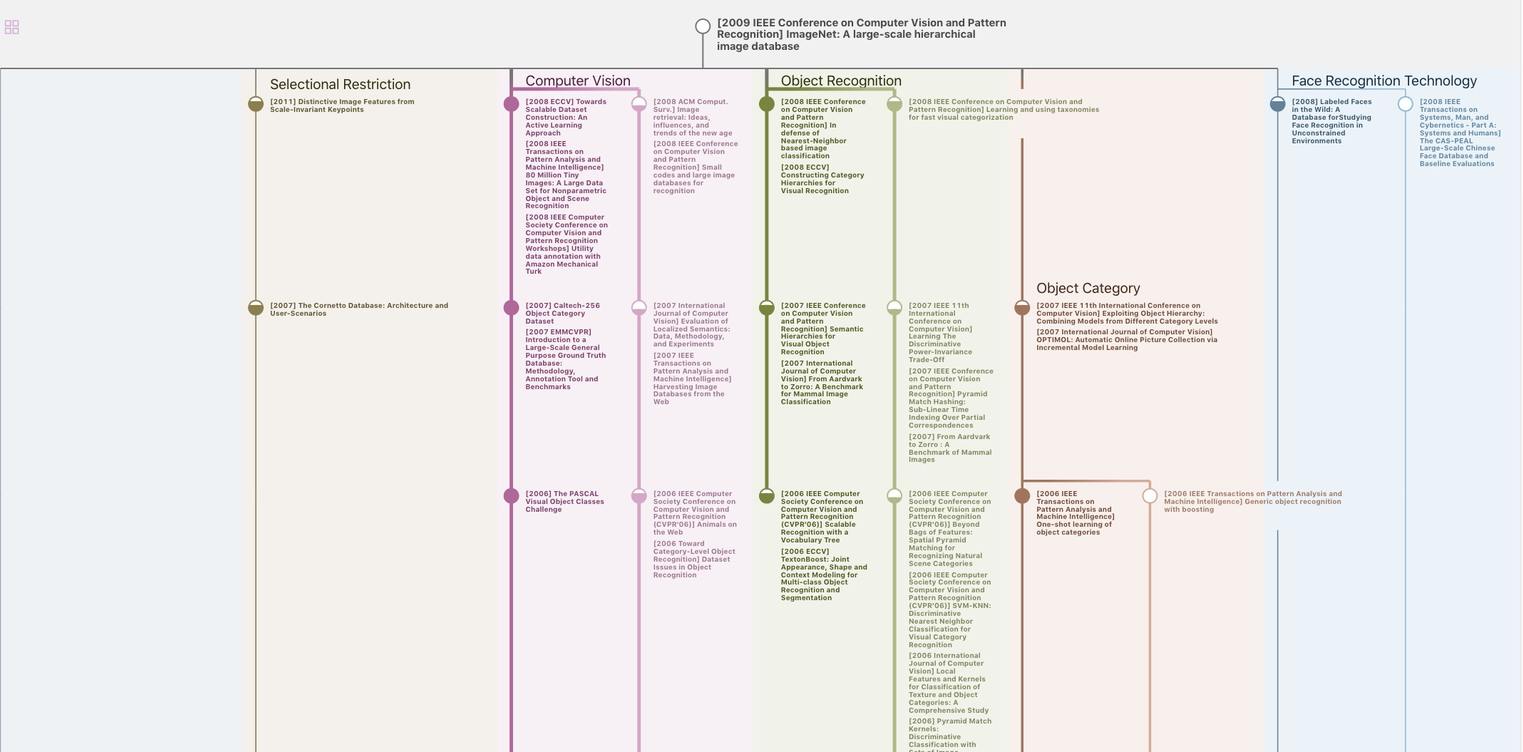
生成溯源树,研究论文发展脉络
Chat Paper
正在生成论文摘要