Enhancing Low-Resource Sanskrit-Hindi Translation through Deep Learning with Ayurvedic TextJust Accepted
ACM Transactions on Asian and Low-Resource Language Information Processing(2023)
摘要
The Machine Translation (MT) community is interested in Neural Machine Translation (NMT) because it can persevere continues data over a range of input and output phrase lengths. The NMT system employs a cutting-edge attention mechanism that enables concentrating on a particular input vector of the input sentence rather than obtaining an entire vector for every input sentence. While the Neural approach enhances translation superiority by addressing issues with enduring dependencies and making contextual analysis more accessible, it also requires a sufficient parallel corpus for training, which is challenging in dialects with limited resources. The primary goal of this research is to tackle the distinctive difficulties associated with translating Ayurvedic texts using neural machine translation (NMT). Ayurvedic texts pose unique challenges due to their specialized vocabulary and domain-specific terminology, necessitating a customized approach to achieve precise and meaningful translations. To create a reliable neural machine translation system for the Sanskrit-Hindi language pair, this study utilized a manually created parallel corpus containing Ayurvedic texts and general domain texts. With the help of this corpus, two NMT models were constructed: an attention-based transformer model (NMT2) and an attention-based encoder-decoder recurrent neural network (NMT1). These models integrated deep neural network techniques to optimize the translation process and overcome the constraints of limited training data and complex grammatical structures of Sanskrit. For Sanskrit-to-Hindi, the transformer-based approach received a higher average BLEU score of 48.1 percent and a uni-gram BLEU Score of 56.86 percent.
更多查看译文
关键词
Corpus,Machine Translation System,Sanskrit,Hindi,Neural machine translation
AI 理解论文
溯源树
样例
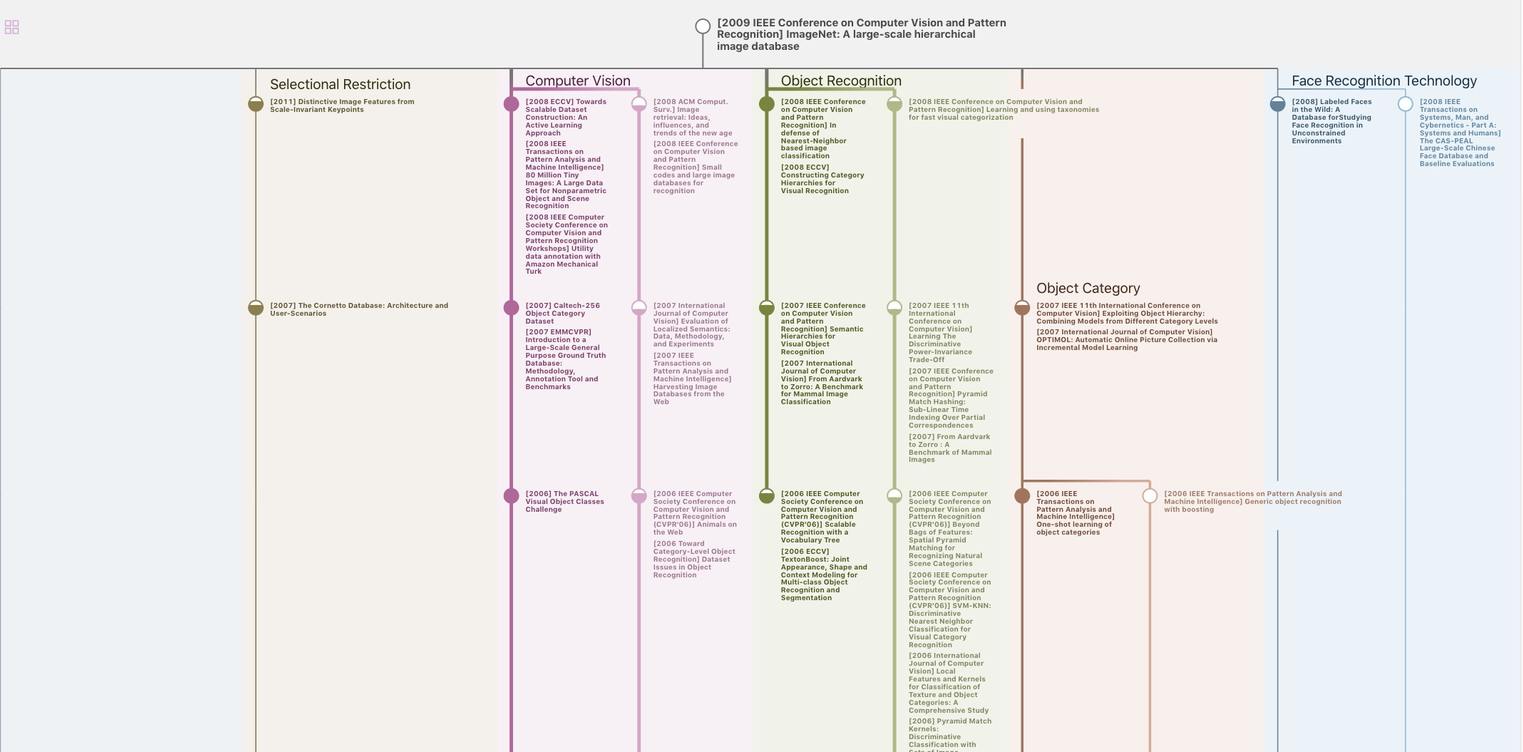
生成溯源树,研究论文发展脉络
Chat Paper
正在生成论文摘要