Pedestrian Attribute Recognition via Spatio-Temporal Relationship Learning for Visual SurveillanceJust Accepted
ACM Transactions on Multimedia Computing, Communications, and Applications(2023)
摘要
Pedestrian attribute recognition (PAR) aims at predicting the visual attributes of a pedestrian image. PAR has been used as soft-biometrics for visual surveillance and IoT security. Most of current PAR methods are developed based on the discrete images. However, it is challenging for the image-based method to handle the occlusion and action-related attributes in real-world applications. Recently, video-based PAR is attracted much attention in order to exploit the temporal cues in the video sequences for better PAR. Unfortunately, existing methods usually ignore the correlations among different attributes and the relations between attribute and spatio region. To address this problem, we propose a novel method for video-based PAR by exploring the relationships among different attributes in both spatio and temporal domains. More specifically, a spatio-temporal saliency module (STSM) is introduced to capture the key visual patterns from the video sequences, and a module for spatio-temporal attribute relationship learning (STARL) is proposed to mine the correlations among these patterns. Meanwhile, a large-scale benchmark for video-based PAR, RAP-Video, is built by extending the image-based dataset RAP-2, which contains 83,216 tracklets with 25 scenes. To the best of our knowledge, this is the largest dataset for video-based PAR. Extensive experiments are performed on the proposed benchmark as well as on MARS Attribute and DukeMTMC-Video Attribute. The superior performance demonstrate the effectiveness of the proposed method.
更多查看译文
关键词
video-based pedestrian attribute recognition,spatio-temporal relationship learning,IoT security
AI 理解论文
溯源树
样例
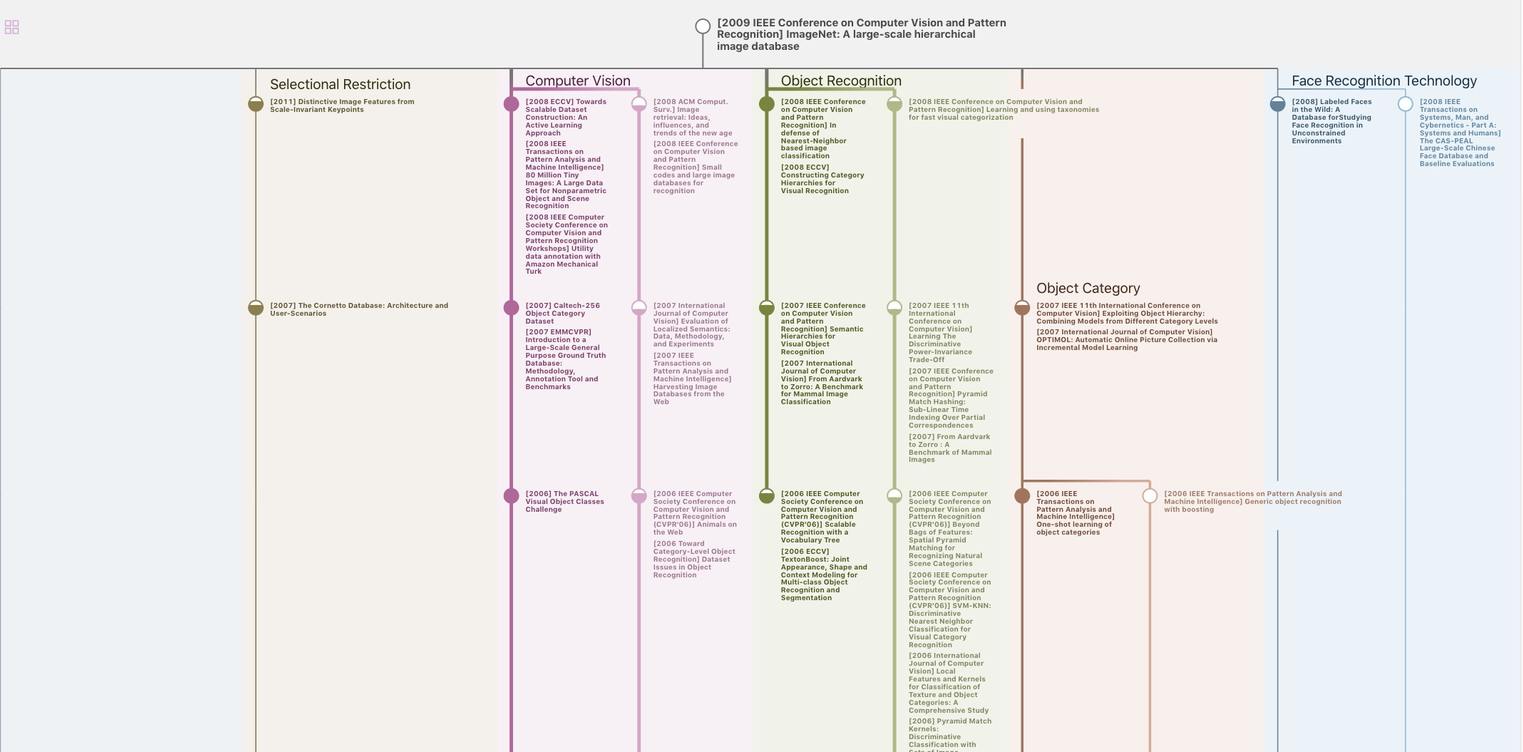
生成溯源树,研究论文发展脉络
Chat Paper
正在生成论文摘要