A Reconfigurable Framework for Neural Network-based Video In-loop FilteringJust Accepted
ACM Transactions on Multimedia Computing, Communications, and Applications(2023)
摘要
This paper proposes a reconfigurable framework for neural network-based video in-loop filtering to guide large-scale models for content-aware processing. Specifically, the backbone neural model is decomposed into several convolutional groups and the encoder systematically traverses all candidate configurations combined by these groups to find the best one. The selected configuration index is then encapsulated as side information and passed to the decoder, enabling dynamic model reconfiguration during the decoding stage. The above reconfiguration process is only deployed in the inference stage on top of a pre-trained backbone model. Furthermore, we devise a Wavelet Multi-scale Poolformer ( WMSPFormer ) as the backbone network structure. WMSPFormer utilizes a wavelet-based multi-scale structure to losslessly decompose the input into multiple scales for spatial-spectral features aggregation. Moreover, it uses the Multi-scale Pooling operations ( MSPoolformer ) instead of complicated matrix calculations to substitute the attention process. We also extend MSPoolformer to a large-scale version using more parameters, referred to as MSPoolformerExt . Extensive experiments demonstrate that the proposed WMSPFormer+Reconfig. and WMSPFormerExt+Reconfig. achieves a remarkable 7.13% and 7.92% BD-Rate reduction over the anchor H.266/VVC, outperforming most existing methods evaluated under the same training and testing conditions. Also, the low-complexity nature of WMSPFormer series makes it attractive for practical applications.
更多查看译文
关键词
In-loop filter,reconfigurable,H.266/VVC,neural model,Transformer
AI 理解论文
溯源树
样例
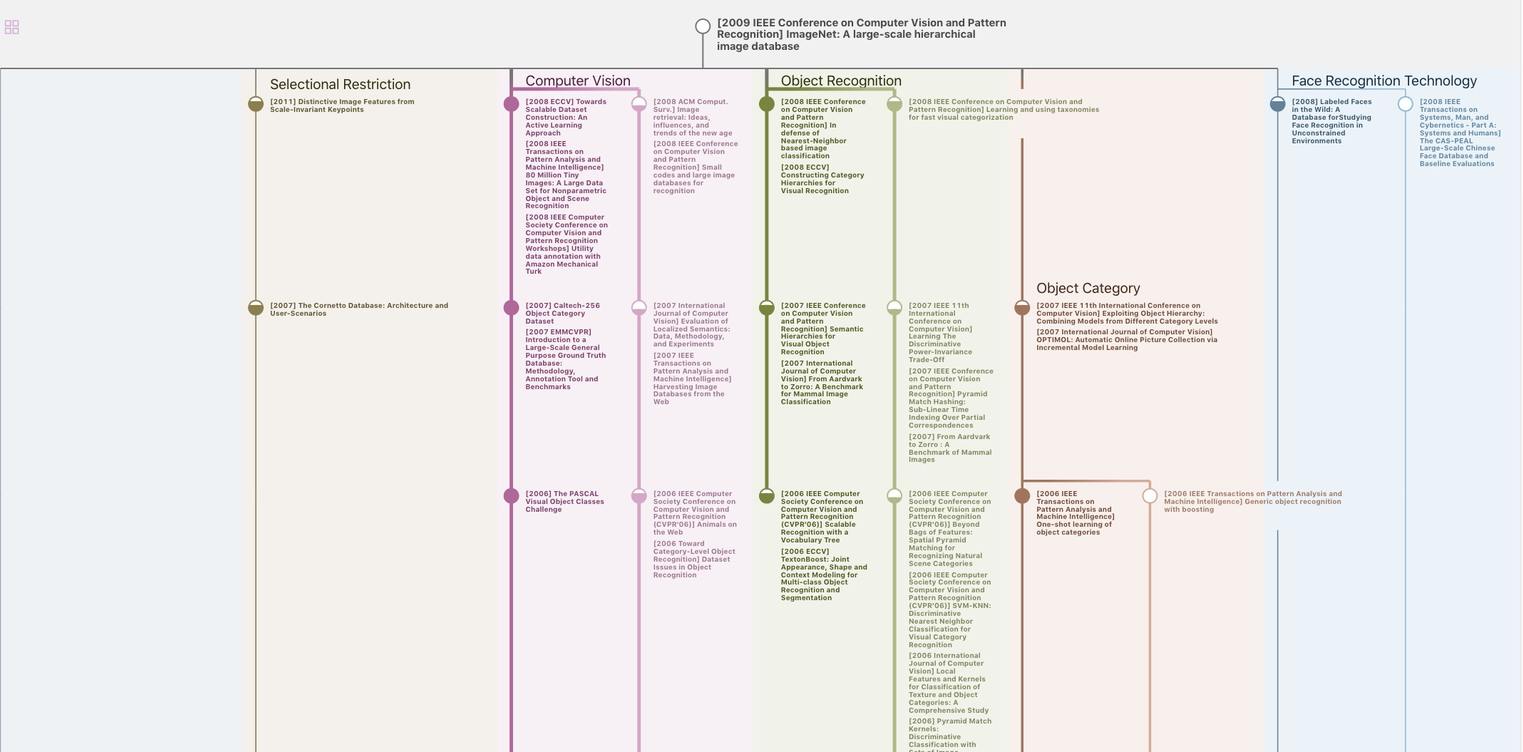
生成溯源树,研究论文发展脉络
Chat Paper
正在生成论文摘要