A new data-driven diagnostic technique for in-situ capacity prediction of lithium-ion batteries
JOURNAL OF ENERGY STORAGE(2024)
摘要
Lithium-ion battery (LIB) charging behavior is of equal importance as discharging with abundant information for the battery management system to determine the state of charge (SOC), state of health (SOH), and state of safety (SOS). However, to the best of the authors' knowledge, little work can be found regarding the fast and accurate prediction of the charging behaviors of LIBs. To fill in this gap, in this work, a data-driven diagnostic technique, long short-term memory in situ capacity prediction (LSTM-ICP), is proposed to predict battery capacity through short-term voltage measurements under constant current charging. Unlike the technique in the previous work, LSTM-ICP does not rely on the interpretation of voltage-time data as incremental capacity (IC) or differential voltage (DV) curves. Thus, the need for differentiating voltage-time data (amplifying the process of measuring noise) and the voltage measurement range including the peak of the IC/DV curve can be ignored and thus significantly shorten the prediction process. The optimization method of the mini-batch gradient descent may reduce the computational complexity and also enhance the randomness of prediction. Results highlight the performance of the proposed diagnostic technique, LSTM-ICP is applied to two datasets, which are composed of four and eight cells. In each case, the evaluation results of different performance indicators in a certain voltage range show that the prediction model has good accuracy and effectiveness.
更多查看译文
关键词
Lithium-ion battery,Capacity prediction,Data-driven,Modeling
AI 理解论文
溯源树
样例
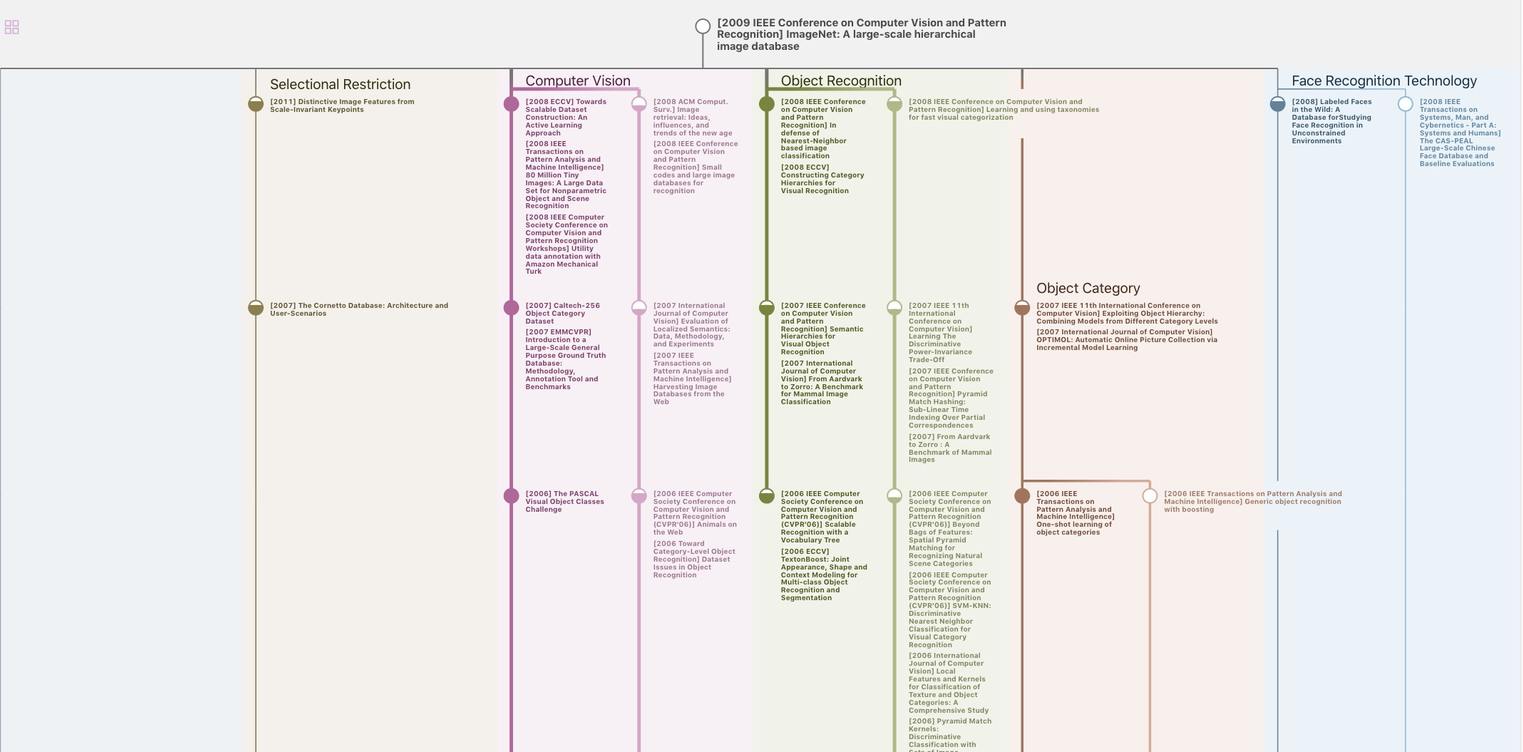
生成溯源树,研究论文发展脉络
Chat Paper
正在生成论文摘要