The influence of optimization algorithm on the signal prediction accuracy of VMD-LSTM for the pumped storage hydropower unit
JOURNAL OF ENERGY STORAGE(2024)
摘要
Pumped storage hydropower units, as a crucial type of energy conversion equipment, play a pivotal role in energy storage and balance. Predicting pressure pulsation signals in pump-turbine units can help anticipate potential unit malfunctions within the pump-turbine system. However, conventional prediction methods may be constrained by their ability to extract signal features and perform pattern recognition. With the continuous advancement in the realm of artificial intelligence, there has been a rise in the evolution of prediction involving variational mode decomposition (VMD) for time series decomposition and the utilization of genetic algorithms (GAs) for parameter optimization of long short-term memory (LSTM) networks has emerged. To address the fitness function issue in the context of genetic algorithms, this study employs three optimization algorithms to combine with the VMD-LSTM model. Three different prediction models are formed and compared with both the conventional LSTM and VMD-LSTM prediction methods. The results indicate that, in the prediction of pressure pulsation data at the three monitoring points of the pump-turbine, the predictive performance of the VMD-whale optimization algorithm (WOA)-LSTM model surpasses that of the conventional LSTM model and the VMD-LSTM prediction approach. Furthermore, the magnitude of prediction errors is reduced in comparison to the VMDsparrow search algorithm (SSA)-LSTM and VMD-improved grey wolf optimization (IGWO)-LSTM prediction models.
更多查看译文
关键词
Optimization algorithm,Variational mode decomposition,Long short-term memory,Pump-turbine
AI 理解论文
溯源树
样例
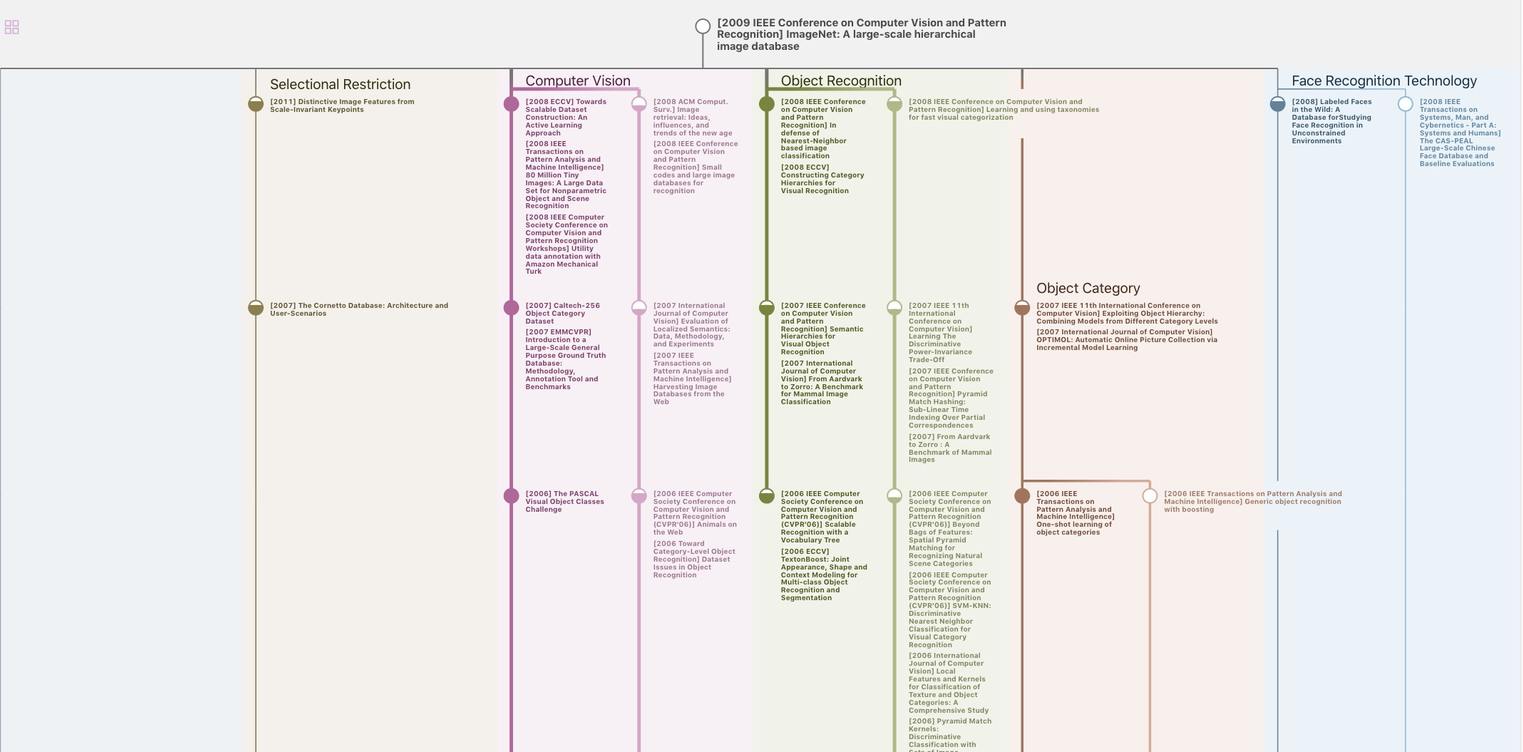
生成溯源树,研究论文发展脉络
Chat Paper
正在生成论文摘要