Influence of machine learning approaches for partial replacement of cement content through waste in construction sector
Asian Journal of Civil Engineering(2024)
摘要
For the purpose of delivering high-quality structures, efficient project management ensures better selection of materials and methods and manpower. To successfully traverse hurdles, optimize workflows, and mitigate risks to achieve deadlines managements deals with latest and smart technologies. Therefore, the Monte Carlo approach was employed to exhaust the data set for this study. The reliability of various regression algorithms is examined by comparing the results of the different eight suitable algorithms. Metrics, such as mean absolute error (MAE), root-mean-squared error (RMSE), R-squared-score, and mean absolute percentage error (MAPE), are used to evaluate the efficacy of a model. According to the findings, three machine learning models—random forest regression, decision tree regression, and the gradient boosting regression model—outperform others when examining the efficacy of various combinations. The effects of using waste material Fly Ash as partial replacements for ordinary Portland cement (OPC) on the compressive, flexural, and split tensile strengths of concrete were explored and compared with the strengths predicted by models using the same experimental datasets. Significance of the basic constituents of the sustainable concrete has been potentially investigated which further leads for potential utilization of waste material, as well. Moreover, XG boost regression algorithms show or almost confirm the close proximity between predicted and actual values of the strengths; it seems the higher accuracy of the model as well.
更多查看译文
关键词
OPC,FA,Root-mean-square error,Strength,Regression models
AI 理解论文
溯源树
样例
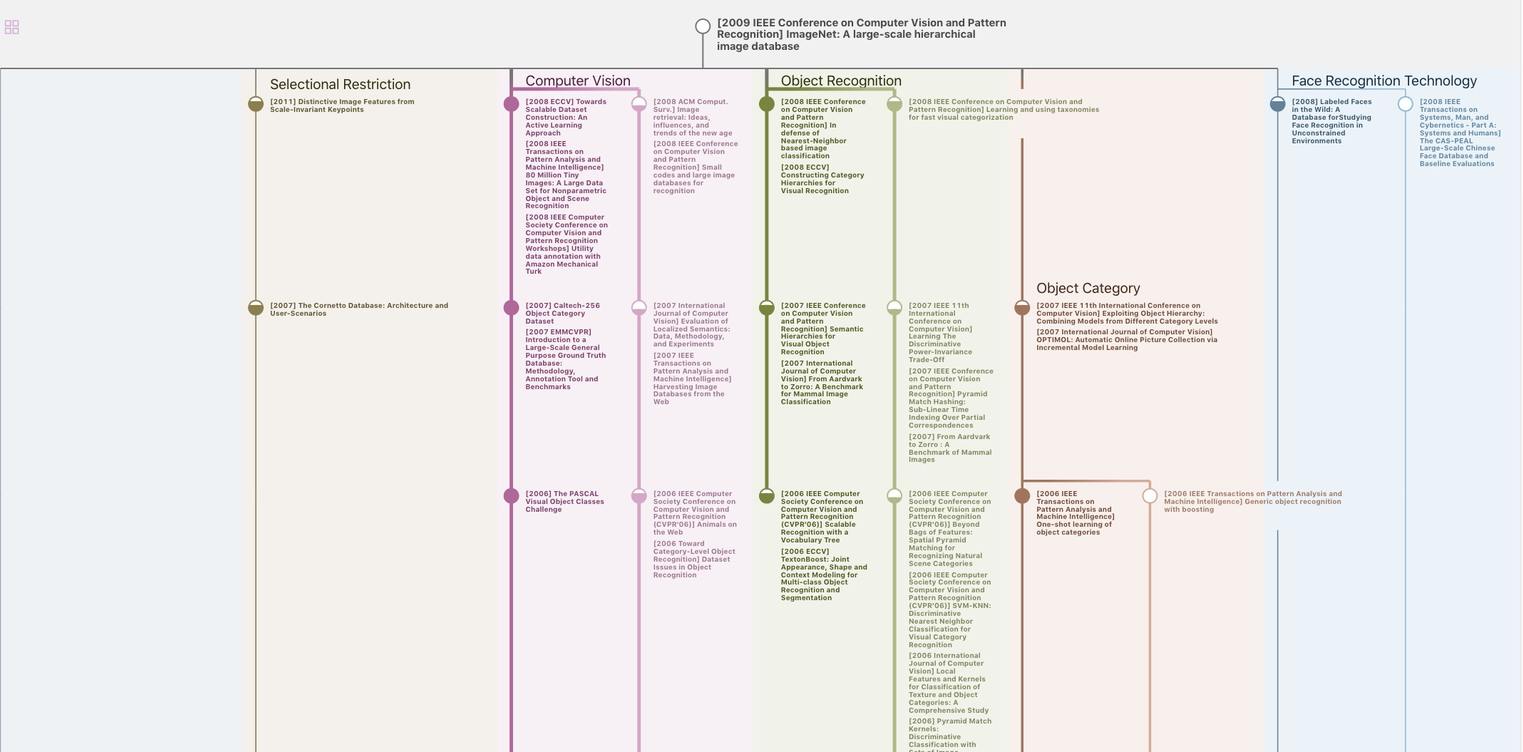
生成溯源树,研究论文发展脉络
Chat Paper
正在生成论文摘要