DeepMMP: Efficient 3D perception of microstructures from Serial Section Microscopic images
COMPUTATIONAL MATERIALS SCIENCE(2024)
摘要
Driven by rapidly increasing volumetric data from serial section microscopy (SSM), deep learning (DL) has been reshaping the research of quantitative materials microstructural analysis and structure-property relationship mining. However, extracting or reconstructing materials microstructures via DL -based image segmentation remains reliant on laborious annotations, and the three-dimensional (3D) spatial information within these volumetric data has yet to be fully exploited. In this work, we introduce DeepMMP, a novel DL -based framework that achieves efficient materials microstructure perception by leveraging the inherent continuity and similarity learned from consecutive SSM images. DeepMMP incorporates unsupervised annotation-free feature learning, 3D image voxel segmentation, and 3D microstructure reconstruction, enabling the effective, efficient, and accurate generation of 3D segmentation and reconstruction results for complex polycrystalline materials with minimal annotations. We believe DeepMMP has the potential to accelerate both process parameter optimization and the design of novel materials.
更多查看译文
关键词
Polycrystalline materials,Serial section microscopy,3D reconstruction,Deep learning,CNNs
AI 理解论文
溯源树
样例
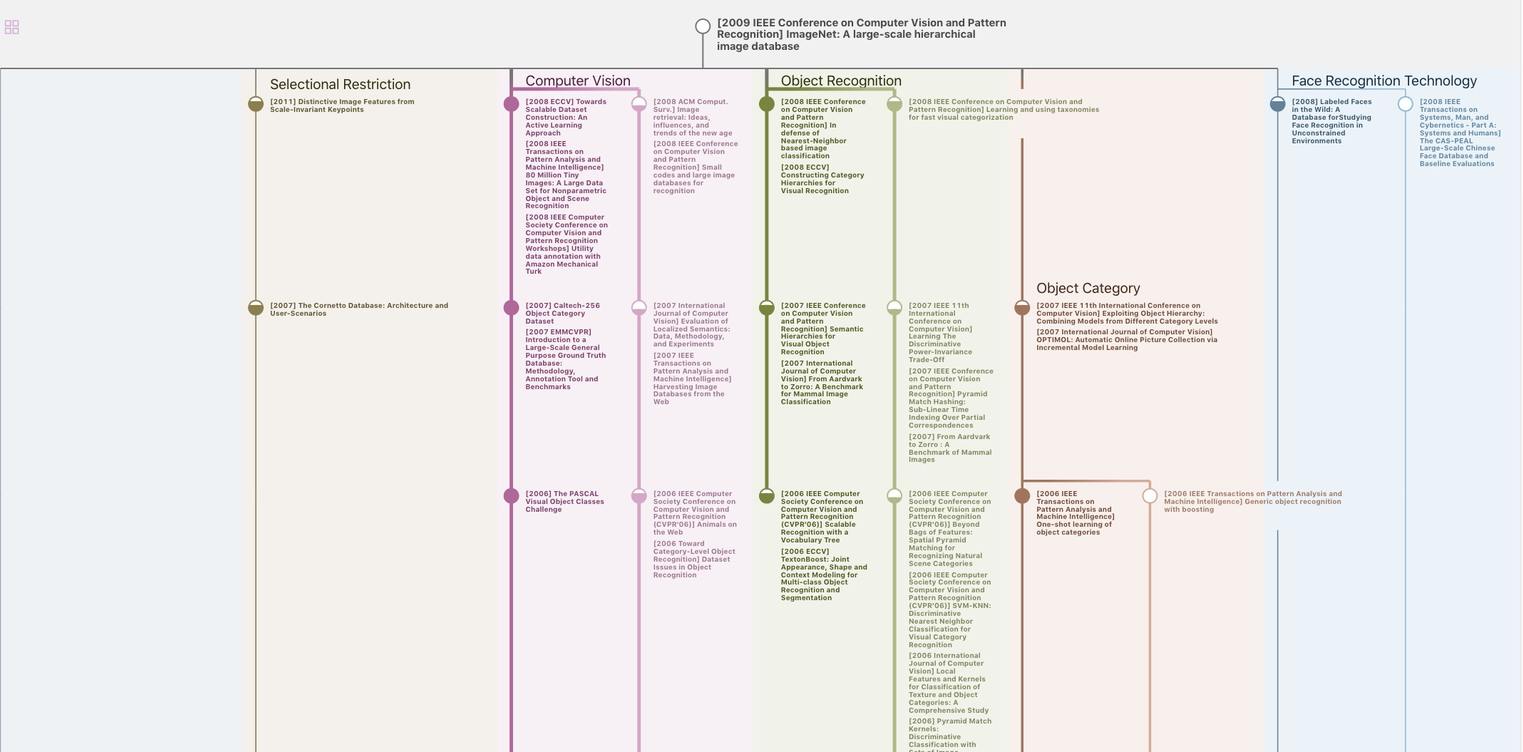
生成溯源树,研究论文发展脉络
Chat Paper
正在生成论文摘要