HCEC: An efficient geo-distributed deep learning training strategy based on wait-free back-propagation
JOURNAL OF SYSTEMS ARCHITECTURE(2024)
摘要
Valuable data is often distributed across multiple data centers (DCs). Deep learning (DL) tasks, constrained by privacy regulations, utilize local training and model averaging to facilitate collaborative training across multiple DCs. However, the hierarchical bandwidth within and between DCs diminishes the training efficiency for decentralized data. Therefore, it is imperative to prioritize research efforts aimed at reducing communication overhead while preserving convergence performance for geographically distributed DL tasks. To address this challenge, we propose a High -Convergence and Efficient -Communication (HCEC) training strategy for geographically distributed data. In this paper, we adopt two approaches: (1) to ensure high convergence, we utilize dynamic learning rates and local epochs to avoid local optima; (2) to ensure efficient communication, we introduce the Adaptive Layerwise Communication (ALC) method to minimize inter -DC communication costs. The ALC method decides whether to communicate all L -layer model parameters at once or perform L -times communication based on the available bandwidth and computational training overhead. Experimental results show that compared to the model averaging method, HCEC ensures convergence and improves training efficiency by at most 37.9%.
更多查看译文
关键词
Stochastic gradient descent,Gradient communication,Decentralized environment,Geo-distributed deep learning
AI 理解论文
溯源树
样例
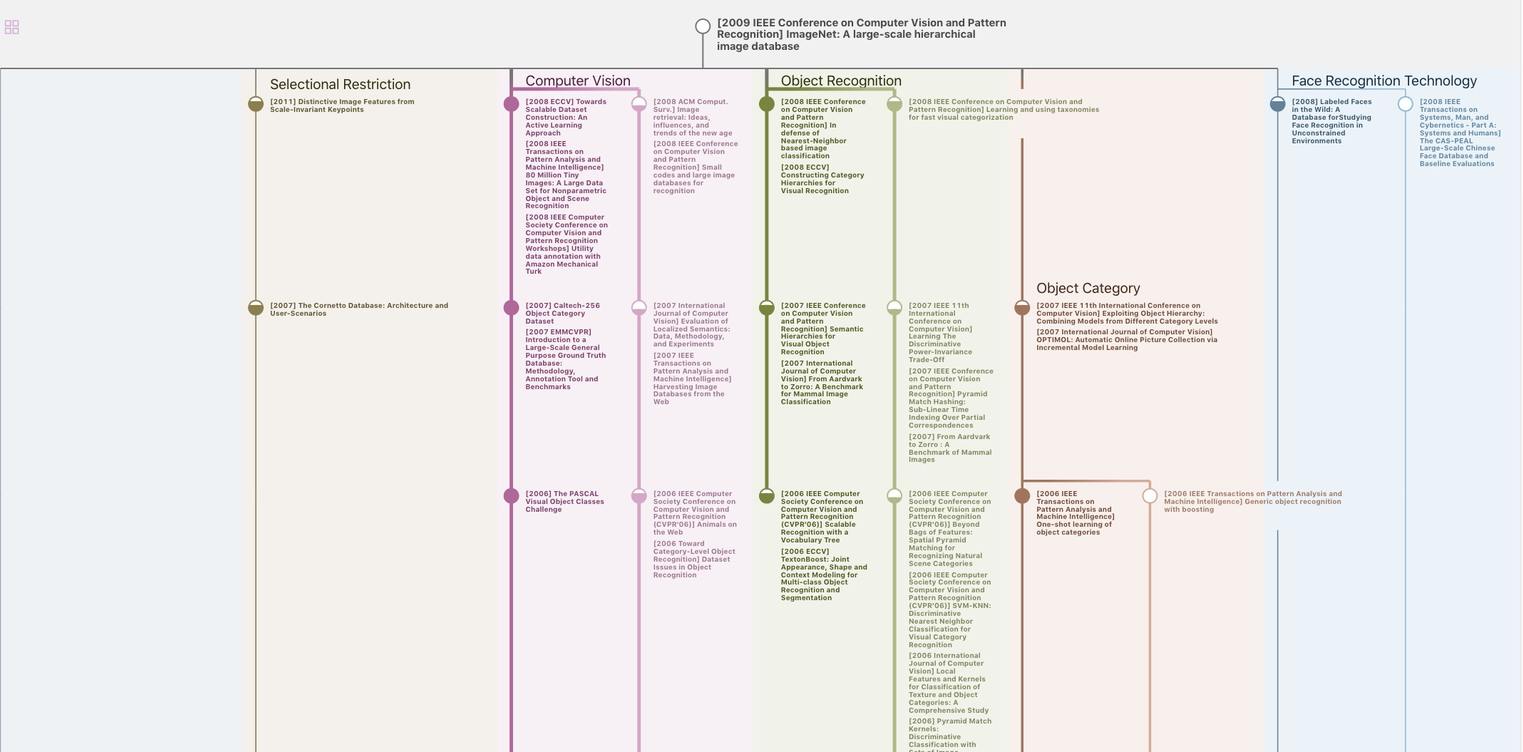
生成溯源树,研究论文发展脉络
Chat Paper
正在生成论文摘要