Network-level short-term traffic state prediction incorporating critical nodes: A knowledge-based deep fusion approach
INFORMATION SCIENCES(2024)
摘要
The critical nodes (CNs) in urban transportation networks, defined as road entities (such as road segments or detectors in a road network) that present highly volatile traffic states, can significantly impact the overall traffic conditions. However, recent studies are unable to explicitly address CNs in traffic state prediction tasks. In this study, we develop a novel knowledgebased graph convolutional gated recurrent network incorporating critical nodes (KGCGRN-CN) to forecast the network-level short-term traffic states. The KGCGRN-CN model consists of three learning blocks: (i) a spectral graph convolution block that captures the network-level and CNlevel spatial features in the data; (ii) a novel knowledge-based spatial feature fusion block that tailors the fusion of the network-level and the CN-level spatial features using geographical and traffic information of each road entity; and (iii) a temporal feature learning block, which captures the temporal patterns of the fused features. The KGCGRN-CN model is compared with several state-of-the-art benchmark models using a real-world freeway dataset. Results show that the developed model outperforms the benchmark models by a mean absolute error of 1.03 km/h on average. Further numerical experiments are conducted to demonstrate the effectiveness of the KGCGRN-CN model.
更多查看译文
关键词
Traffic state prediction,Critical node,Spatiotemporal feature,Graph convolution,Recurrent network,Feature fusion
AI 理解论文
溯源树
样例
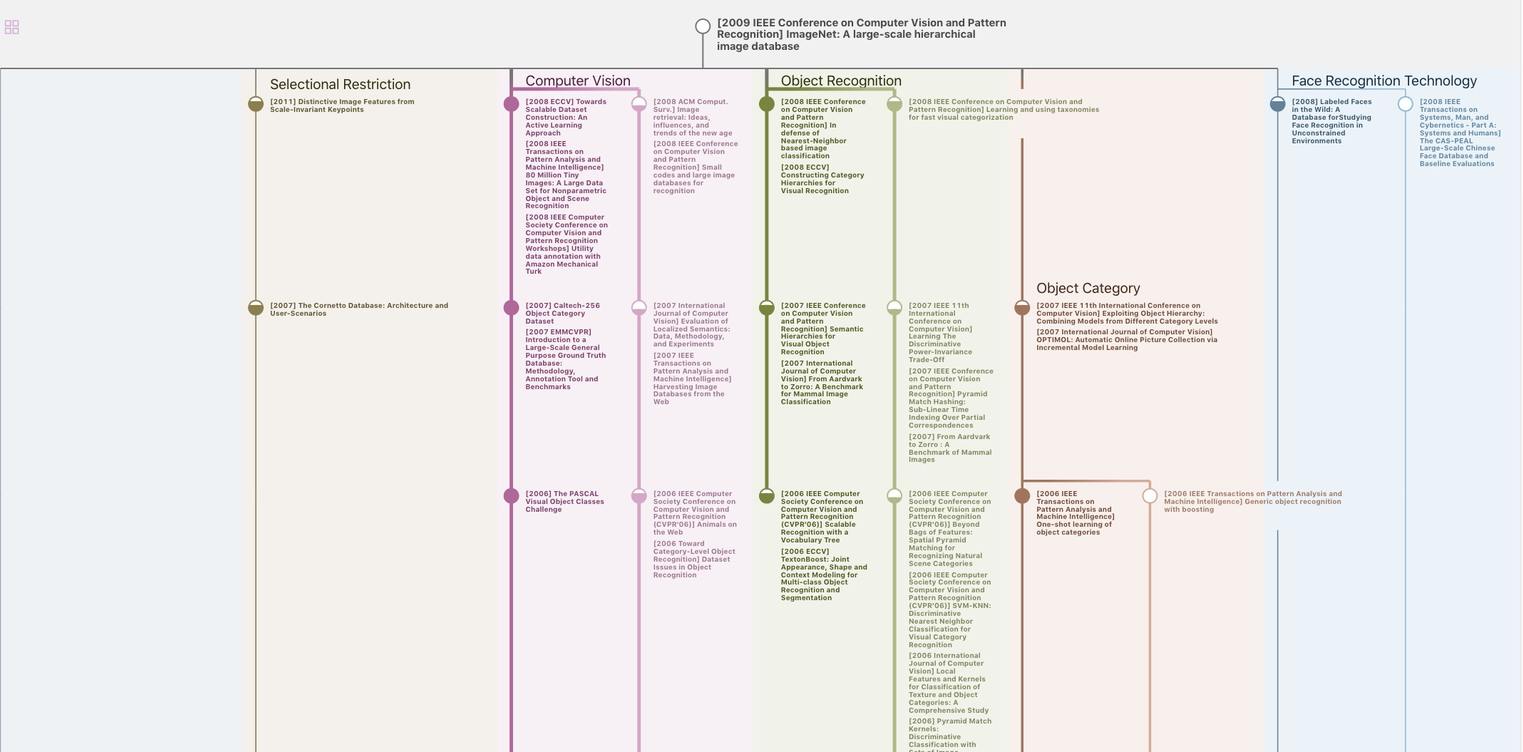
生成溯源树,研究论文发展脉络
Chat Paper
正在生成论文摘要