On-Chip Optimization and Deep Reinforcement Learning in Memristor Based Computing.
IEEE/ACM International Symposium on Nanoscale Architectures(2023)
摘要
Reinforcement learning (RL) has shown its viability to learn when an agent interacts continually with the environment to optimize a policy. This work presents a memristor-based deep reinforcement learning (Mem-DRL) system for on-chip training, where the learning process takes place in a dynamic cartpole environment. Memristor device variability is taken into account to make the study more realistic. The proposed system utilized an analog ReLU module to reduce analog to digital converter usage. The analog Mem-DRL system consumed 191 times less energy than an optimized digital FP16 computing system. Our Mem-DRL system reduced the ADC usages by 40%, which led to reduced the overall system energy by 42%. Mem-DRL is 2.4 times faster than the FP16 system and performs 9.27 GOPS during DRL training. The system exhibited an energy efficiency of 23.8 TOPS/W.
更多查看译文
AI 理解论文
溯源树
样例
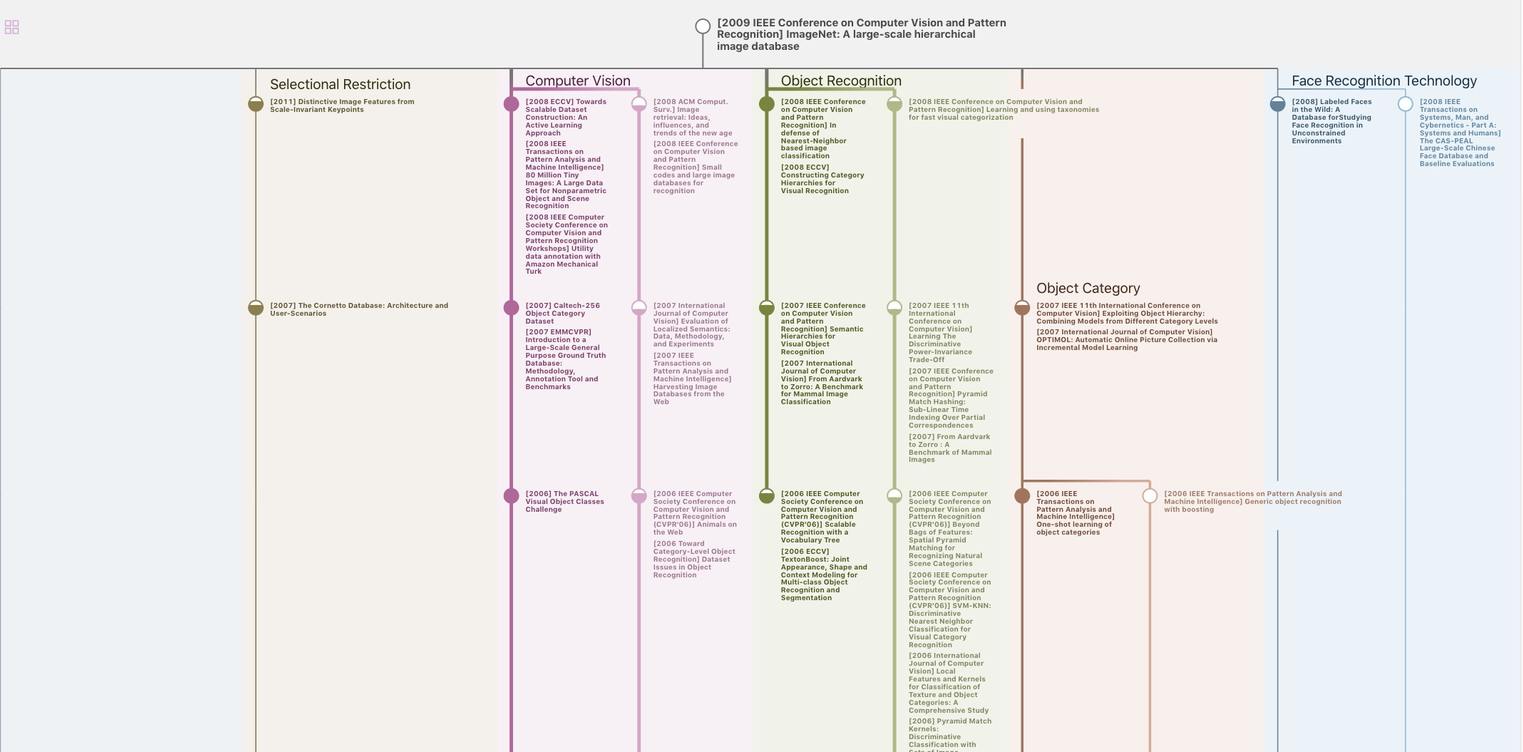
生成溯源树,研究论文发展脉络
Chat Paper
正在生成论文摘要