Black-Box Access is Insufficient for Rigorous AI Audits
CoRR(2024)
摘要
External audits of AI systems are increasingly recognized as a key mechanism
for AI governance. The effectiveness of an audit, however, depends on the
degree of system access granted to auditors. Recent audits of state-of-the-art
AI systems have primarily relied on black-box access, in which auditors can
only query the system and observe its outputs. However, white-box access to the
system's inner workings (e.g., weights, activations, gradients) allows an
auditor to perform stronger attacks, more thoroughly interpret models, and
conduct fine-tuning. Meanwhile, outside-the-box access to its training and
deployment information (e.g., methodology, code, documentation,
hyperparameters, data, deployment details, findings from internal evaluations)
allows for auditors to scrutinize the development process and design more
targeted evaluations. In this paper, we examine the limitations of black-box
audits and the advantages of white- and outside-the-box audits. We also discuss
technical, physical, and legal safeguards for performing these audits with
minimal security risks. Given that different forms of access can lead to very
different levels of evaluation, we conclude that (1) transparency regarding the
access and methods used by auditors is necessary to properly interpret audit
results, and (2) white- and outside-the-box access allow for substantially more
scrutiny than black-box access alone.
更多查看译文
AI 理解论文
溯源树
样例
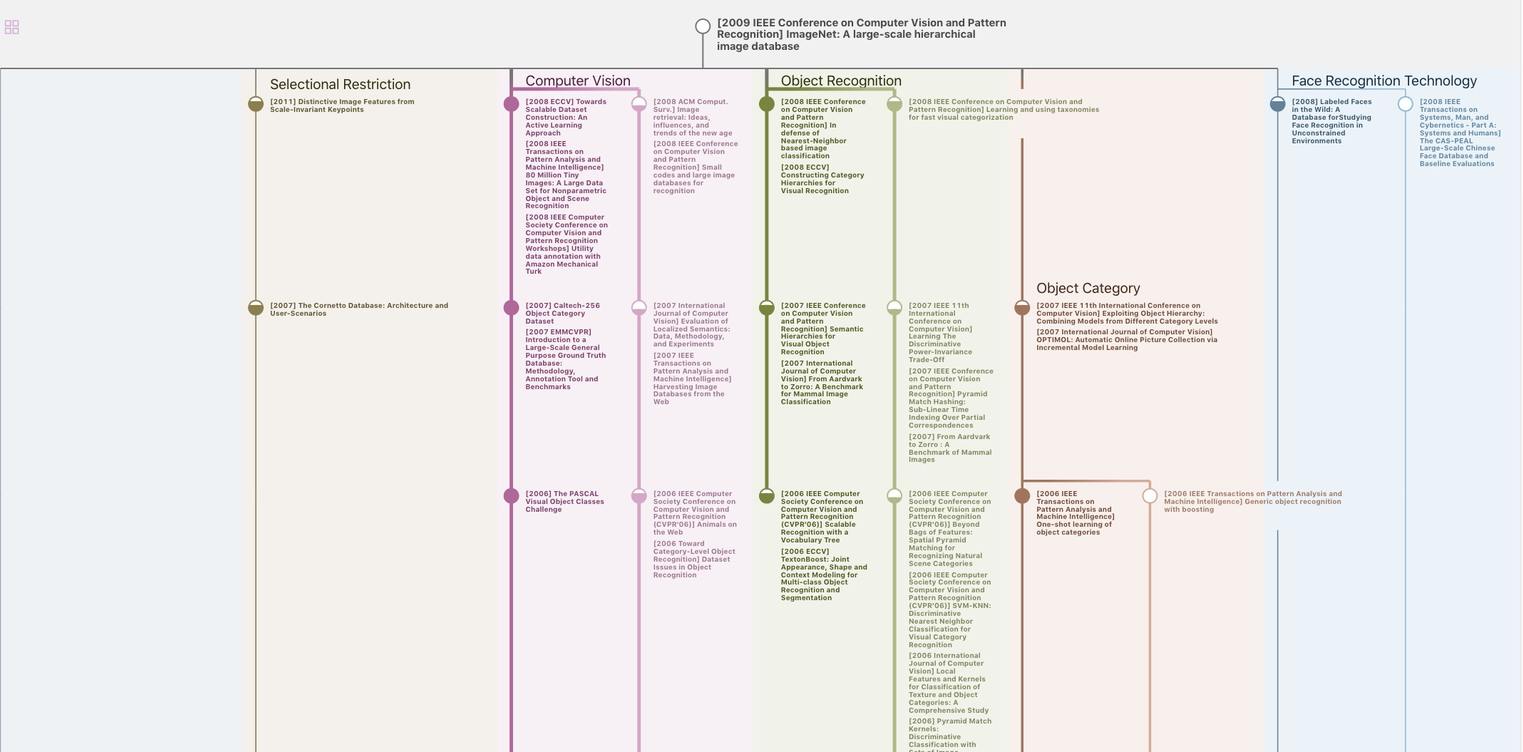
生成溯源树,研究论文发展脉络
Chat Paper
正在生成论文摘要